Big Data doesn’t need to be big, or daunting
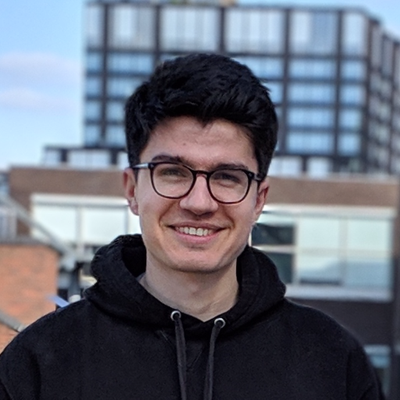
- Dr Nicolai Baldin, CEO & Founder at Synthesized
- 28.02.2020 12:00 pm big data
Collecting and collating customer information in order to create new, or enhanced products and services is a strategy as old as business itself, but the process involved has always been a huge time and resource drain for companies.
Yet in the digital era we now live in, companies are creating and handling more data than ever before. They are awash with it, to the point where many firms even struggle to identify what data is mission-critical. Digital transformation projects have put data at the centre of every organisation, with businesses estimated to have spent nearly $1.2 trillion globally to digitise their operations to fit the ever-expanding digital economy.
With such transformations underway across huge swathes of businesses, they do still face two, quite fundamental issues around “big data”.
Firstly, many companies simply overlook the hidden costs behind data such as the time and resources required. For example, for those involved in data science, it is estimated that 80% of their work is solely focused on the acquisition and preparation of data. This leaves them with just 20% capacity to focus on actual data analysis, which can provide crucial insights to propel a business forward.
Secondly, a significant issue is also how to actually access and share data to make it truly useful for commercial purposes, especially at a time when data is now seen as a competitive advantage for companies. For example, it is estimated that up to 60% of all data within an enterprise is unused.
Issues around how data can be safely shared are now paramount. In fact, businesses have never faced more complex regulatory requirements as they do now in regards to how data should be shared. The General Data Protection Regulation (GDPR), introduced in 2018 into the European Union to impose stiffer data protection regulations and require businesses to obtain consent in certain situations in order to process data.
Making this regulatory environment even more complex is that individual countries also have their own data protection laws. Germany has the Federal Data Protection Act (FDPA) which sets even stricter requirements for processing personal data than GDPR. Meanwhile, France has revised its Data Protection Act to impose stricter rules concerning biometric information collection. In Italy, the country is implementing more rigorous criminal sanctions around the unlawful processing of personal data.
The list goes on and on of countries seeking to deal with the issue of how data is collected and handled, with an eye, overall, on ultimately protecting personal, consumer data as much as possible.
With such hidden costs and regulatory requirements placed on businesses, big data would appear to be quite big, and quite daunting.
Yet technology is now disrupting (for the better) these central issues. Thanks to artificial intelligence (AI) and machine learning (ML) technologies huge advances are being made in how businesses can analyse data in a much more cost-effective and efficient manner, while also ensuring regulatory compliance.
These technologies have helped to create synthesized data, which is able to mimic original data, without any of the data protection headaches. How is such accurate data created? An ML engine generates synthetic data sets by learning and reinforcing the structure of information found in original data. It is able to move beyond surface statistics to capture and reproduce the complex multi-dimensional patterns underlying realistic data sets. Unlike anonymisation and encryption techniques, this approach enables any company to share better quality information in a format useful for machine learning and analytics, without, crucially, disclosing any information about individual data points.
You might ask how close to original data is synthetic data? Research from MIT found that synthesized data can give the same results as real, original data. Synthesized data also completely takes away the risk of non-compliance with data regulations such as GDPR. Finally, to top it off, the ML technology is so powerful it can generate this data in minutes and break the siloes associated with data completely, thus breaking those hidden costs associated with big data.
It can be hard to know where to start in trying to tackle the challenge provided by big data. Yet synthesized data is a real, viable approach which takes away many of the risks associated with collection and handling of huge amounts of data. The benefits of this approach are now too hard to ignore - knowledge and insights can be unlocked without jeopardising personal data.