Big Data in the Upstream Sector
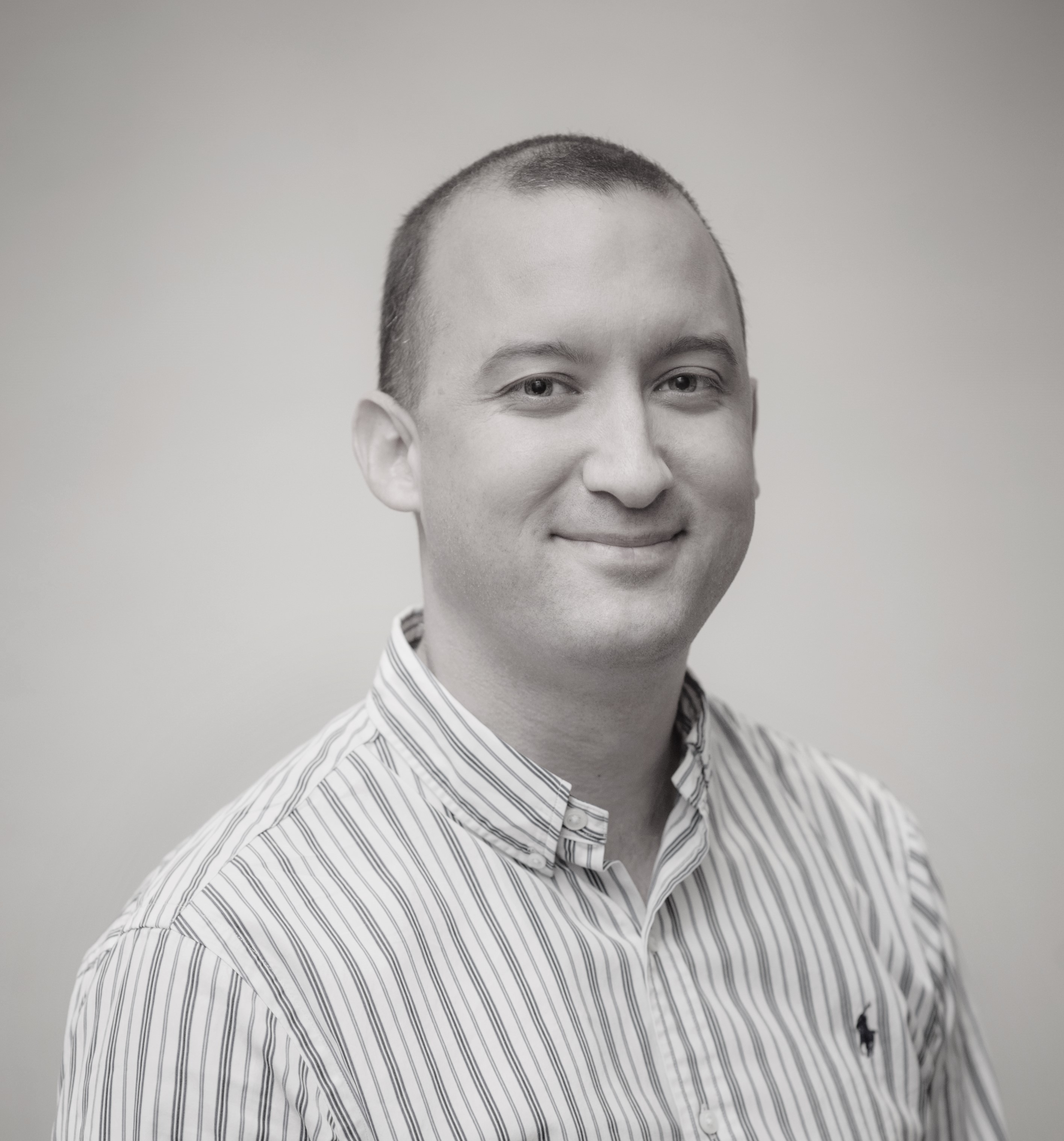
- Grant Eggleton, Vice President, Global Production Solutions at P2 Energy Solutions
- 09.05.2017 09:15 am big data , Grant is Vice President, Global Productions Solutions at P2 Energy Solutions. He has more than 20 years’ experience in the sales and delivery of software based solutions to the resource industries, with a particular focus on upstream oil and gas. In his role he has worked with many clients globally in the delivery of integrated operations solutions with a focus on production and reservoir engineering, surface operations and hydrocarbon accounting.
It’s not the data itself, but the ability to put it towards some useful task that matters. In the oilfield, a simple yet powerful way of making use of data and predictive analytics is for automatic surveillance by exception. By learning from the past, it is possible to predict what should be happening today and compare the prediction to what is actually going on. If the two do not match, then a problem has been identified and a solution found. Addressing problems early is one of the cheapest ways to boost production.
The need for improved prediction
Wells that are big producers are typically watched by many eyes. Extensive instrumentation records pressures, temperatures, volumes and equipment performance parameters at regular intervals, which can be as often as every minute. This enables a large staff to quickly respond to unusual readings. However, engineers and instruments are expensive and, in oilfields with multiple small wells, a single person is often responsible for the production of a hundred wells for which they receive information only once daily. Horror stories abound in which wells failed to produce at expected rates before an operator caught on and the situation was remedied. Yet, if data can be used to effectively provide a metric against which to measure current production, a simple, inexpensive system that requires no instrumentation other than the production monitoring, well testing and production allocations can potentially save millions of dollars in deferred or lost production. Having a grounded metric for production is an effective means of computing automatic warning alarms and of correlating production alarms to geographical location, geology and particular operators.
It’s not just engineers who need improved surveillance based on data that is already being collected and generally available. There are many other parties like for instance non-operating partners, royalty owners and lenders, who too have an interest in making sure that production does not drop below reasonable levels. Yet, they have limited access to data other than production records.
The key to improving surveillance is improving prediction because the more accurately one can predict what production should be, the easier it is to spot deviation from the ideal.
How to achieve improved surveillance
The best tool to achieve improved surveillance is a library of accurate, probabilistic and unbiased oil and gas production forecasts for both individual and aggregate wells by reservoir, operator, basin or region. These forecasts should provide the full range of future production possibilities by combining map and production data with long-term production forecasts generated in an automated fashion and updated monthly.
But how can you forecast future volumes accurately to build your library? You will need a technology solution that gathers and interprets monthly oil, gas and water allocated volumes and records of working days (if available).
Such technology should consist of a physical part explaining data in terms that are physically plausible, and a statistical part explaining normal deviations from physical behaviour such as stimulations, shut-ins and bad data. Once the samples are drawn, they can be pushed forward to make a probabilistic forecast. Statistics are then computed over the samples. Rigorous calibration with blind testing are needed to ensure that the statistics of the samples match real data.
Because of its statistical nature, the solution can be used as an easily understood and controllable tool for surveillance. It can be used to set thresholds of a very specific nature – namely, the user can ask to be warned when production goes below a particular p-value and they can expect to receive warnings from each well under surveillance. The number of alarms can be reduced by using filters that reflect other factors such as changes in water cut, known shut-ins for maintenance and the like.
In summary, technology that helps you understand data and forecast events can provide constant watch over your operation and trigger alarms and alerts when defined events occur, allowing less staff to effectively manage larger portfolios and more assets. As the volume of data from across organisations continues to grow, it becomes harder and harder to monitor KPIs and operating conditions through traditional dashboards relying on manual views and analysis. The sensible approach is an automated exception management through user defined rules and conditions that monitor data and initiate alerts.
Conclusion
Knowing what to expect is ultimately the key to surveillance. Having a better understanding of how wells are going to perform in the future is a powerful tool that represents a step change for the oil and gas data information sector. Better understanding always leads to better decisions.