Why Graph Database Could Be Key to Addressing Financial Services Challenges
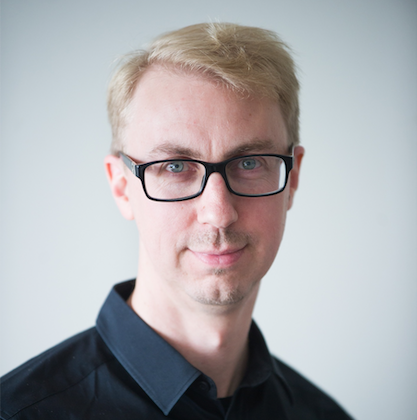
- Emil Eifrem , CEO and Co-Founder at Neo4j
- 28.03.2019 07:45 am financial services
In the database management world, graph database is huge right now. Industry and vendor-neutral site DB Engines has shown that, since 2014, the graph data model has far outpaced every other data model in terms of growth, while IT analyst company Gartner recently identified graphs as one of itsTop 10 Data and Analytics Technology Trends for 2019.
This explosion in technologist interest in graphs hasn’t passed the financial sector CIO by; it's 20 of the top 25 banks use graph database software, for instance, in a wide variety of use cases – from infrastructure to supply chain, in big data projects and supporting real-time activities.
That’s because financial services companies are now able to join the dots and read relationships in their data they were previously unable to do. Graph was, after all, the technology used to investigate thePanama and Paradise Paperswhich exposed the financial dealings or mis-dealings of a global elite – a project of incredibly complexity and which was also all about uncovering hidden patterns in big data that nothing else was able to crack.
Using graphs to get over key process hurdles
Graphs were built to do this. They differ from traditional relational databases in that they specialise in managing the relationshipsbetween a large number of data points, enabling the graph system builder or data investigator to better manage their data.
With graph databases, you aren’t constricted by semantically-limited data models and costly, unpredictable ways of running queries through joins the way the relational approach obliges you to do. Instead, graph supports working with extensive named, directed relationships between entities (or nodes) that provide a richer semantic context for the developer to work with. This means that features of the business universe being modelled can be outlined in much greater detail – and even more usefully, graphs are incredibly fast at creating these patterns. Financial assets, it goes without saying, are complex and interdependent systems: the good news is that via graph technology, financial services firms can get an overall and trackable understanding of the relationships between all of their critical assets.
Financial firms are also turning to asset graphs to work out derivatives pricing in real time, where the pricing formula needs to incorporate interdependencies between items and accurately spotlight the risk and reward ratios. Regulatory compliance is also a great fit for graphs in financial services firms, providing a deep dive into data, information and process flows. Sarbanes-Oxley and GDPR other regulation requires us to understand who has access to what data, what data resides in which systems, and how data flows across the organisation, and it’s notoriously the case that data is often copied across different systems in its infrastructure. Graph software is proving to be an invaluable aid here as it can efficiently model data lineage and data flows to provide a complete understanding of siloed data and systems. That means that as well as being incredibly useful for regulatory compliance work, it can also help accelerate any projects that may be reliant on this data.
Helping get one step ahead of the fraudster
Cybercriminals are continually playing cat and mouse with finance firms, continually looking for new ways to avoid being detected. In today’s Information-driven Age, financial services firms run up against major barriers in identifying and stopping online fraudulent activity. Traditional anti-fraud solutions, such as those that raise the red flag if a customer deviates from their normal purchasing patterns, depend on discrete data – which works in tracking down individual criminals, but it doesn’t keep pace in detecting sophisticated fraud rings.
Fraudsters, for example, may steal several identities and mix them up to form one ‘new’ synthetic identity to open bank accounts, apply for loans and credit cards, for example. Traditional fraud detection systems are simply not able to detect these. The answer is a solution to spot activities that at first sight may appear unrelated, but are actually connected. Real-time graph traversal, linked to the right type of events, can help financial services firms to determine such multifaceted fraud rings during, or even after, fraudulent transactions happen.
Another major task for financial services firms is money laundering; we need to know where funds are coming in from and where they are going. But criminals are very clever at using misdirection to make it very difficult to follow funds from their start to end point. Again, time-honoured anti-money laundering solutions have not been developed to link the dots between the many steps criminals may take but require a large amount of manual effort, making them incredibly time consuming. With graph, however, anti-money laundering teams at financial services firms can (and do) model companies, accounts and transactions much more efficiently in order to spot possible money laundering.
There are also great internal uses for graph databases at financial services customers we’re working with. We’re seeing customers use graph databases to help run their own IT environments more efficiently by ‘graphing’ their data centres, networks and other IT systems, creating graph-based maps of their topologies to optimise processes. Use cases number using graphs to help with dependency management, impact analysis, network management, downtime reduction, root-cause analysis and routing to quality-of-service mapping.
A way to map all sorts of complexity
Increasingly many bank DevOp teams tell us that because of the growing complexity and interrelated nature of their IT infrastructure, only a graph database can properly map and query all of the data they are required to model today. A typical tier one bank, for example, will have an enormous infrastructure sprawl of legacy and modern kit, all connected via a mesh of physical and virtual networks and subnets and all exposed to risk.
Here is where graph comes into its own as a graph data model can identify single points of failure within a network before the vulnerability is known, while real-time, connected data queries can notify internal security experts if typical attack patterns are occurring within a given network, even if individual data points don’t appear to be malevolent.
Finally, customer expectations are rising at a time when customer service is a significant differentiator. Today’s global, digitally-savvy customer expects companies to deliver personalised service that reflects an understanding of who they are, their communication preferences, the products and services they’ve purchased in the past and what they might be interested in in the future. Powerful, real-time banking and insurance product and service recommendations engines have become central to both a streamlined user experience and business success. For these strategies to work, financial services firms need a complete 360-degree view of customer data, something many brands really struggle with due to data being siloed and sitting in many disparate databases. The power of connected data in the form of data relationships is opening up new opportunities for financial services firms, enabling them to retain and improve their competitive edge.
All these use cases share an underlying theme – the need for the business to better understand connected data relationships. Graphs are emerging as an incredibly powerful way of helping deliver just that, at scale, in real-time – making it very well worth your while to see what all the graph buzz is about.
The author is CEO and Co-Founder of Neo4j, the world’s leading graph database company.