Optimizing Risk Management with Machine Learning in Banking
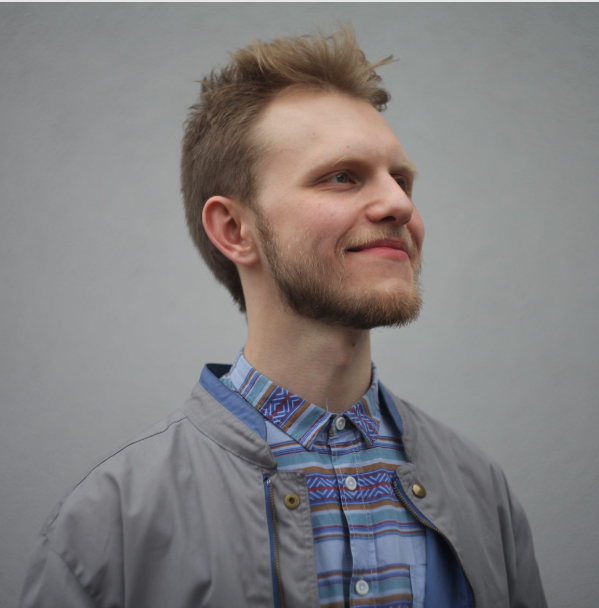
- Andrey Koptelov , Innovation Analyst at Itransition
- 26.05.2021 05:45 pm Banking
The enormous amounts of data accessible to banks and their high demand for forecasting make the financial industry a perfect area for machine learning (ML) to shine. In this article, we explore the current applications of machine learning in banking when it comes to risk management, define its challenges and provide a future outlook.
Credit Risk Management
For the past few decades, banks have mostly used logistic and probit regression models for credit risk assessments and internal risk management. However, all conventional models inherit the same flaw — they predict outputs based only on linear relationships between input variables.
This limitation was exposed in the catastrophic 2008 housing market crash. Although the crisis’s negative consequences have been multiplied by uncontrolled sales of credit default swaps and other complex financial instruments, the fundamental reason for failure was in the inaccurate credit risk model.
In the aftermath, with the intent to force financial institutions to provide more detailed reports, The Federal Reserve’s CCAR now requires banks to account for more than 2,000 economic attributes. Consequentially, this also led to other regulating authorities introducing new standards that improve supervisory data quality and reporting.
At the same time, with the proliferation of banking apps, social media, and digital communication overall, financial institutions now collect lavish amounts of unstructured data. If gathered and processed correctly, these new datasets can help gauge critical insights for a wide range of banking operations.
This is where machine learning comes into play. More advanced non-linear approaches to credit risk modeling including neural networks enable banks to make predictions with a previously unseen level of accuracy and granularity.
Challenges
The utter superiority of machine learning over traditional credit risk modeling approaches comes at the cost of the prevailing ‘black box’ problem. While we can decide to trust ML algorithms based on statistical evidence of their feasibility, current regulatory constraints won’t allow it to happen.
However, machine learning can still be used to a great extent while being regulation-compliant. Even simple linear machine learning approaches still yield more accurate results than conventional ones. Many banks also use unsupervised machine learning methods to explore data, while using traditional classification and regression models to make predictions.
Fraud Management and Surveillance
Nowadays, the majority of banks’ fraud detection systems use rule-based approaches. This causes banks to deal with a significant number of false positives, forcing them to spend inordinate amounts of resources to distinguish meaningless behavioral deviations from real threats.
The ability of machine learning to capture subtle trends and uncover non-linear relationships allows banks to get a complete picture of a client’s activity and significantly lower the probability of false positives. For example, by integrating ML into its fraud detection model, Danske Banks managed to reduce false positives by 60%.
Challenges
Similar to many other AI-based solutions in the financial space, the biggest adoption hurdles concern regulations and the unexplainability of AI systems. For example, depending on the jurisdiction, banks are often unable to provide developers with sensitive information related to past breaches. Next, the outputs of unsupervised monitoring systems sometimes can’t be explained, which makes them non-compliant.
However, financial institutions have found a way to at least partly leverage the power of ML for fraud management. A fraud prevention system’s alerts will still be triggered by rule-based models, but the integration of an ML algorithm on top of them can allow adjusting surveillance methods to a person’s behavior fluctuations. Such ML models are typically less complex and explainable, which makes them applicable in a regulatory context.