The Missing Piece of the Banking Tech Puzzle: the Best Privacy-enhancing Tech Use Cases for Finance
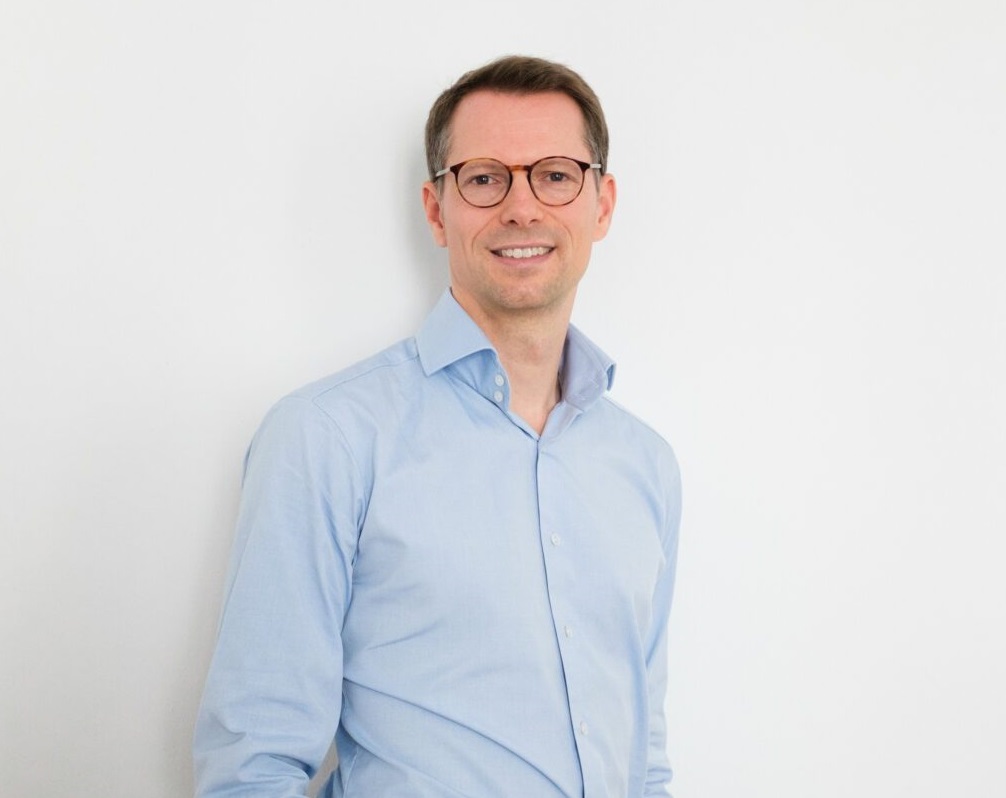
- Tobias Hann, CEO at MOSTLY AI
- 11.04.2022 10:45 am Banking , data , privacy , Tobias - or simply Tobi - joined MOSTLY AI in 2019 and took on the CEO role in 2020. As such, he is responsible for the entire company. Prior to joining MOSTLY AI he worked as a management consultant with the Boston Consulting Group and as Co-Founder/MD of three start-ups. He holds a PhD degree from the Vienna University of Economics and Business and an MBA from the Haas School of Business, UC Berkeley.
Innovators in banking are facing a huge dilemma. Implement high-value data use cases with success and stay ahead of the curve or watch neobanks and big tech slowly conquer their market. Increasingly strict data privacy legislation and the growing complexity of legacy data architectures put banks and financial situations in an even tighter spot. Banking has always been the trusted data repository of transactions and so the bar for protecting data is very high. The reason why traditional financial institutions are falling behind is the reluctance to go data-driven, for fear of endangering customers’ trust. Luckily, this is no longer an either or choice.
Privacy-enhancing technologies (PETs) can be the lifeline traditional banks and financial institutions can grab. There are numerous PETs ready to be integrated into existing architectures, from solutions like AI-generated synthetic data for high dimensional anonymization to homomorphic encryption, enabling encrypted data analysis and anonymized computing technologies like federated learning. Each comes with advantages and disadvantages and the use case must decide which one is the right choice.
One of the most prominent PET technologies with lots of high value use cases is AI-generated synthetic data. Synthetic data in banking is set to transform the data landscape for good with forward looking banks already heavily investing in the technology. According to Gartner, by 2024, 60% of the data used in AI and analytics projects will be synthetically generated. Synthetic data sets look and feel like production data since they are modeled on original datasets but contain no re-identifiable data points. In contrast to old-school anonymization technologies, the intelligence contained within the original data is fully preserved.
According to J.P.Morgan, synthetic data is one missing piece of the financial AI puzzle, demonstrating true human behavior in business scenarios. For example, when banks look for 360-degree customer views, synthetic customer journeys might be their only option. In an increasingly regulated environment where customers demand privacy, personalized services, and algorithmic fairness, synthetic customer data offers a compliant and very effective tool to tackle all of these challenges. While traditional data anonymization tools like data masking destroy the statistical correlations and merely obscure embedded biases, synthetic data retains the intelligence contained within the data and can help fix bias.
These qualities make synthetic data especially useful for the development of AI and machine learning models, hungry for pattern rich, representative training data. Another synthetic data banking use case gaining traction is test data generation for software development. Due to strict internal policies, an intricate web of legacy systems, and third-party involvement, meaningful test data is hard to come by. Some banks resort to testing their flagship mobile banking apps with 1 cent transactions and leave development and QA teams deprived of meaningful transaction data. Even though having highly realistic customer and transaction data is mission-critical for developing cutting-edge and robust digital banking apps offering seamless and personalized services. Synthetic data generators are a perfect fit for test data generation. They are capable of augmenting datasets, for example, subsetting a representative but a much smaller and manageable version of your production data.
Other privacy-enhancing technologies include homomorphic encryption, an encryption method that allows for basic data manipulation of encrypted datasets. This method is especially useful in cases where the original data needs to be decrypted at some point in the data life cycle, and some data processing by a third party needs to take place. The most prominent use case would be Anti Money Laundering, where perpetrators need to be reidentified.
Federated learning is another PET banks can take advantage of. Here, the need to share sensitive data is removed completely since the models are trained on edge devices, such as mobile phones. The model is shared instead of the data, which still holds some privacy risk.
No matter which use case we look at, there is a lot to be gained from using PETs instead of legacy anonymization technologies. Compliance teams, cybersecurity, and those looking to build great software products and BI will be equally excited about the prospect of a peace deal between privacy and utility.