Risk professionals need to embrace machine learning – before it’s too late
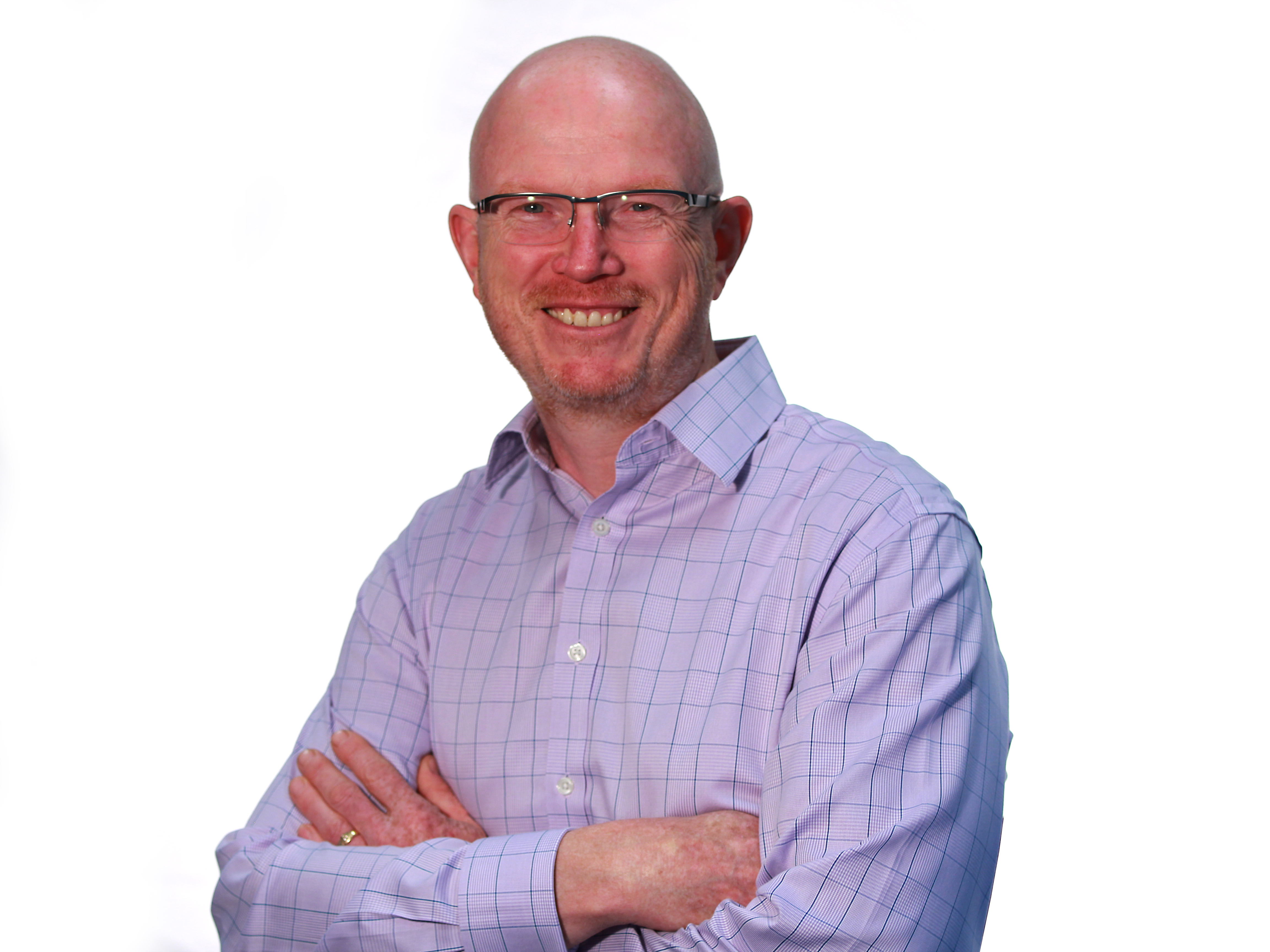
- Dave Webber, Director of concept management data strategy and innovation at TransUnion
- 04.09.2018 11:30 am undisclosed
Over the last few years, smart home devices have become increasingly popular amongst consumers. From retail to social media, and finance to marketing, machine learning technology is leading to substantial improvements in service and productivity. But, for many, the idea of opening a bank account with your Alexi or Google Home is a long way off. This nervousness is reflected in the credit risk industry, which has struggled to warm to the use of machine learning. Why has the industry remained hesitant about using the technology and why should machine learning actually be at the heart of their financial decision-making?
Creating a buzz
It seems like machine learning has suddenly become a buzzword. But the reality is that its use has been growing over the last few years because of the sheer amount of data we have at our disposal and the computer power we have available. In addition, regulators around the world are beginning to understand the effectiveness of these techniques and therefore see them in a more favourable light. For the credit risk industry, it’s never been easier to use machine learning to make more informed decisions on lending or credit. Yet, something is holding risk professionals back.
Breaking down barriers
Scepticism about machine learning largely stems from fears about the ability to explain the output from the models. Understandably, some financial services businesses are reluctant to adopt machine learning to make financial decisions instead of a person (or pre-existing trusted algorithm) until they are 100% confident in its ability. In comparison, their counterparts in other industries such as retail or customer service, are often less risk averse and more willing to test new technology. Even though the technology’s benefits are clear, to make it work effectively, risk analysts need more depth of data and a critical understanding of the area they’re working in.
As a first step, this suspicion needs to be overcome and we must recognise that the technology behind machine learning already surrounds us, even if we aren’t always aware of it. It’s crucial that credit risk professionals start their journey towards embracing machine learning now. This may require a leap of faith for it to become an integral part of credit risk processes but the advantages they will reap will be worth it.
Better lending
One of the key benefits will be enabling them to make better decisions – especially as regulators have begun to loosen the parameters within which lenders and fiscal institutions work. This will not only encourage greater competition but greater transparency around decisions and practices from those who are already there. As the credit risk sector pushes transparency to the forefront, lenders should use machine learning more frequently as a new way to solve existing problems.
When using risk and affordability modelling, lending decisions need to be justified and risk professionals need to ensure that the data going in is in line with the final decision. Lenders can analyse large quantities of data using a model called supervised learning, allowing them to discover relationships that previously haven’t been visible before making a decision and use the machine’s output to explain their decision to a regulator or a consumer.
The evidence
Some may ask: what evidence is there that machine learning actually works for the credit risk industry? At Callcredit, we ran a year-long trial to show the benefits that machine learning’s predictive accuracy can provide. The results were encouraging: they hint at the potential financial benefits for adopters of the technology in the credit, fraud and insurance industries. We found that in one scenario, the level of default in a portfolio of 60,000 credit cards was reduced significantly, with overall bad debt dropping by 10 per cent. This is just a fraction of the benefits that machine learning can bring.
Unlike what some may think, machine learning will not give human jobs to robots or change the nature of what creditors do. Rather, it will complement the systems already in place, improve their efficiency and enable better lending decisions. Rather than allow their doubts about the advantages of using machine learning to stop them from enjoying its benefits, risk professionals should embrace the technology to see for themselves the improvements it can provide.