How to Overcome Barriers to Adopting Predictive AI in Investment Management Processes
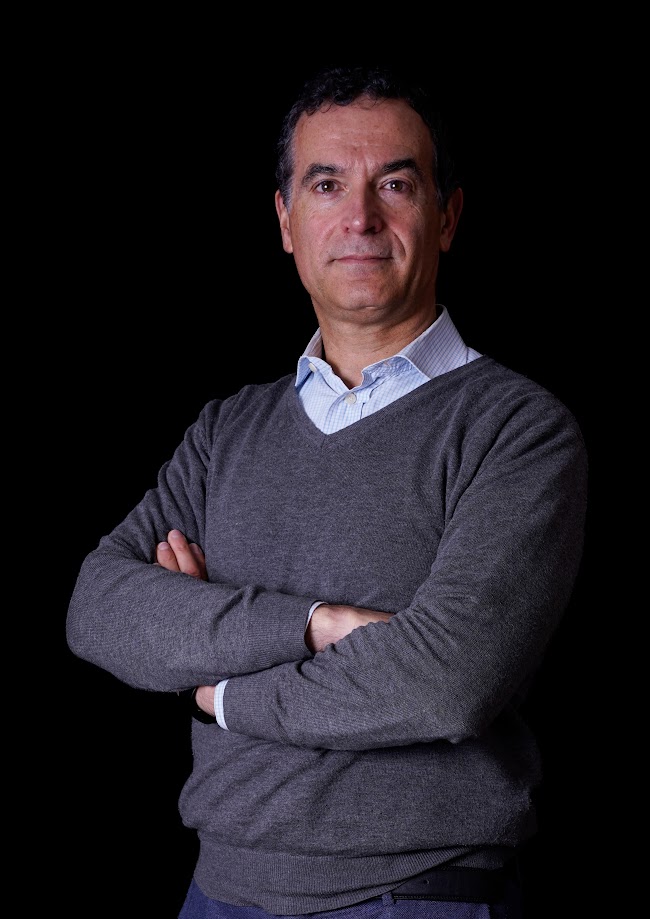
- Giovanni Beliossi, Head of Investment Strategies at Axyon AI
- 19.02.2025 04:45 pm #PredictiveAI #InvestmentManagement
Adopting predictive AI in investment management can be a transformative move for portfolio strategies, albeit one surrounded by hurdles. Despite these challenges, the potential benefits make it a pursuit worth undertaking. Key obstacles include inconsistent or poor data quality, a shortage of in-house AI expertise, and a general unease with the “black box” nature of AI-driven models. According to McKinsey, around 90% of AI initiatives in Europe remain stuck in the testing phase, underscoring the difficulties many firms face when trying to scale cutting-edge technologies within their operations.
A primary challenge is the need for clear model explainability. Investment managers must demonstrate a high degree of transparency when presenting insights to stakeholders and clients, yet AI outputs can often appear opaque or overly complex. Such opacity fuels scepticism and stalls broader acceptance. Additionally, many organisations still lag in digital maturity; low levels of company-wide digitalisation hamper AI’s successful integration and the effective use of its outputs. Without explainability, AI-generated intelligence cannot be seamlessly absorbed into institutional decision-making.
To overcome these barriers, investment management firms should consider introducing AI incrementally, embedding and testing models within existing investment procedures to build confidence. Using explainable AI tools—those that highlight the primary drivers behind daily signals—can boost trust by offering insight into the reasoning behind each prediction. Collaborating with specialised AI solution providers can also help close the talent gap, while internal education initiatives prepare teams to adopt and work with AI-based processes. Smaller investment teams or those with limited in-house quant talent, in particular, may find it challenging to procure the high-quality data and expertise required for sustainable AI projects.
Lastly, securing a suitable computing infrastructure is crucial. Training on vast financial datasets demands both sophisticated model development and considerable computational power, sometimes best accessed through partnerships with established data centres. Although there is no universal blueprint for integrating predictive AI into investment workflows, combining incremental experimentation, collaborative technology approaches, and human expertise allows firms to unlock AI’s transformative potential. In doing so, investment managers can gain a powerful competitive edge over those not yet ready to embrace AI’s capabilities.