All Alone – the Liquidity Conundrum in Fixed Income
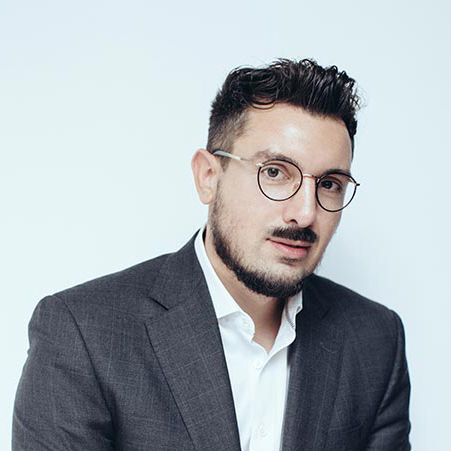
- Vuk Magdelinic, CEO at Overbond
- 29.06.2021 05:45 pm liquidity
Liquidity is a hot topic. It’s garnering increased scrutiny at funds and dealers and with regulators around the globe. The SEC recently enacted a rule requiring open-ended mutual funds to assess their liquidity risk, maintain a liquidity risk management program, classify their holdings into various liquidity buckets, hold minimum amounts of liquid assets and set limits on the illiquid assets they hold.
It’s imperative that portfolio managers, traders and risk managers know the liquidity profile of their aggregate holdings and of the individual instruments they trade. And instruments can’t just be subjectively classified as liquid, or illiquid – their liquidity needs to be quantified to be properly modelled and monitored and to be used by the AI models that are increasingly being deployed to automate fixed income trading on both the buy and sell-side.
But what is liquidity? How is it defined? How can it be measured? In what ways is it different in the fixed income markets than in the equity markets? While understanding liquidity in equity markets has become easier as the technologies have evolved, modelling liquidity in fixed income markets for dealers on the sell-side and risk managers in asset management is still far from straightforward.
One of the key differences is that there are many views amongst participants in fixed income markets as to how liquidity is defined, whereas in equities and vanilla derivatives the situation is more black and white. This is in large part a result of the fractured nature of fixed income, which bring together many different types of security and execution methods with some bonds trading very infrequently. As a result, it is much more difficult to model as there is often inadequate long-term data and missing pieces of data in the short term to plug the gap. But it is difficult, not impossible.
Overbond’s existing AI liquidity tool captures various liquidity measures including bid/ask spread, volatility of quotes and size of trades, and then converts them into live succinct intraday liquidity scores for more than 100,000 corporate bonds and fixed income ETFs. It’s indispensable as a contribution to low-touch and no-touch automated fixed income trading, but it’s based on liquidity in the short term which is only part of the picture.
This is where end-of-day TRACE data comes in. By integrating this, we are generating a liquidity score that will provide a general overview of the market conditions by taking a longer-term view of liquidity – for instance, looking at trading size over the past 22 days.
The model is driven by transaction cost data and volume data, but liquidity modelling is still difficult. The TRACE data contains both agency trades and principal trades, but they’re not identified as such. Since they each exhibit different transaction cost characteristics, the data science team are always looking at ways to identify these trades. It’s also important to analyze the data to identify and remove duplicate trades in transaction chains and to remove micro-lot size trades.
Ultimately, to enhance the understanding of liquidity in fixed income, data scientists are key to pushing new developments in liquidity modelling and much harder than in other asset classes. There are suggestions that some of the larger public cloud providers will break into this space using their powerful computing capabilities. But the progress will be made by more nimble firms with specialist knowledge and understanding of this complicated asset class (or more like classes) which larger cross-asset firms and tech providers don’t have. It’s for this reason why fixed income stands all alone in the liquidity conundrum, but also why specialist analytics firms are breaking ground to unlock this.