Forecasts, Automation and Risk Management: Machine Learning’s Impact on the Financial Sector
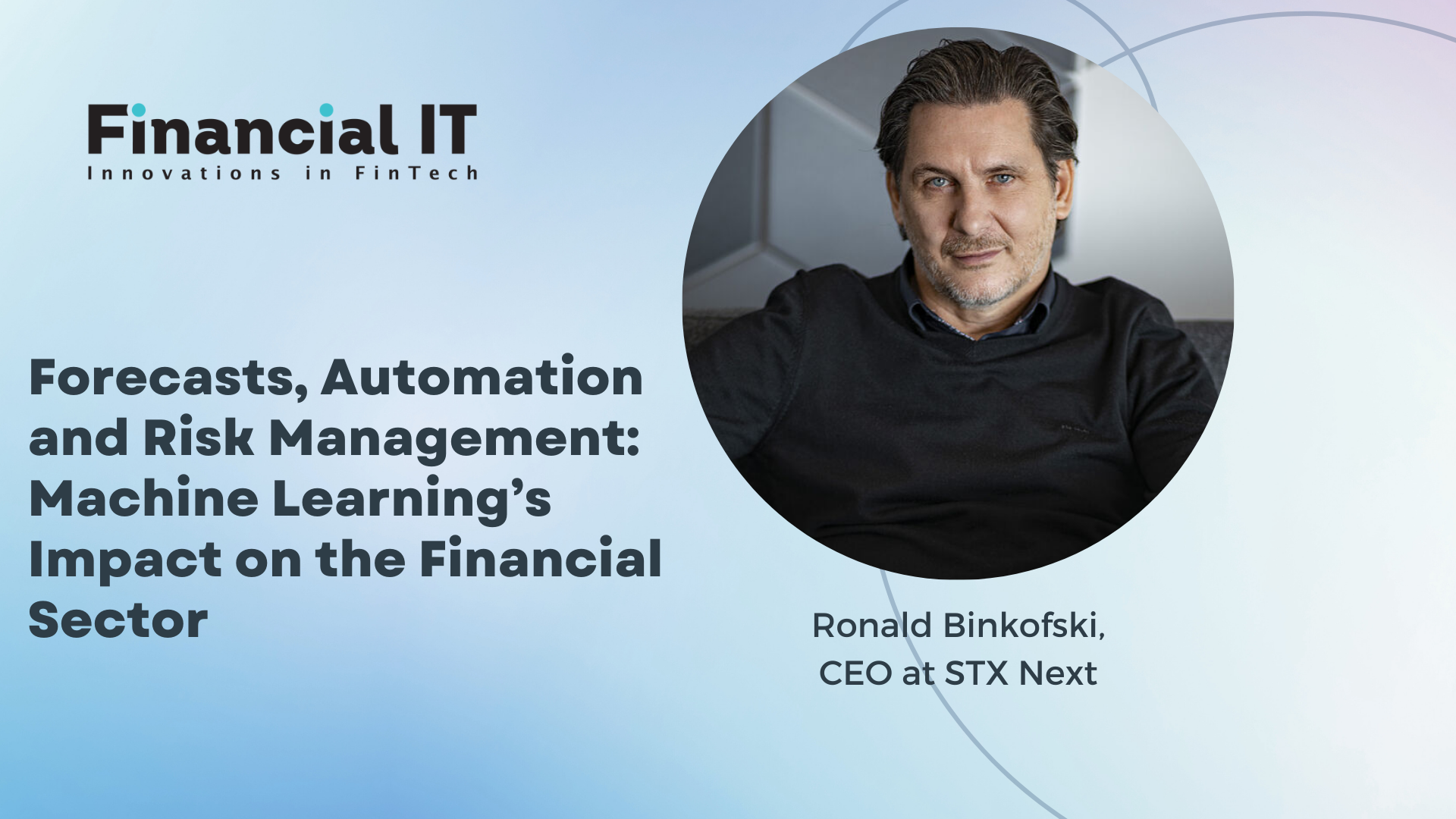
- Ronald Binkofski, CEO at STX Next
- 19.01.2024 10:30 am #fintech #riskmanagement #machinelearning
Armed with the capabilities of machine learning (ML), financial institutions and finance executives have long been turning unstructured information into insights that promote better decision-making and help them understand many key elements of the business. Without these insights, financial institutions are less efficient in several ways, such as being unable to make fast and accurate decisions on whether to approve a loan or invest in particular assets.
The need to make the very most of AI and ML is clear. According to research from the World Economic Forum, 77% of businesses believe that the two closely related technologies will be essential to their business within the next few years.
Aside from helping make faster business decisions, machine learning algorithms can help financial services companies identify and thwart fraudulent activities and cyber threats, or easily incorporate solutions like chatbots and voice assistants to automate customer service tasks. Such activities, powered by machine learning models, are essential for financial companies to stay ahead of their competitors in as the industry continues its rapid evolution.
Processing and analyzing raw data to create valuable insights is especially crucial for the following use cases:
Banking and customer service
At a customer-facing level, machine learning helps the banking industry deliver better customer experience and improve operational efficiency. Teams can eliminate manual, repetitive tasks to free up time for strategic work, with machine learning technology offering greater automation, accuracy, and data-driven predictions. Banking institutions can easily detect anomalies in accounting entries by comparing them to other entries for similar transactions.
When it comes to customer service, machine learning-based chatbots and virtual assistants reduce the time customers have to wait before being served. Some of those tools can perform simple tasks like sending notifications, while other AI functions can also manage customer claims, ensuring each of them is handled efficiently and accurately.
Insurance
Machine learning is also an integral part of the creation of new insurance technologies and applications. Paired with automation capabilities, machine learning algorithms can bring improved efficiency in areas such as policy management and financial monitoring. It also supports risk assessment processes, enabling financial companies to analyse vast amounts of financial data and predict risk profiles accurately. With the resulting insights, companies can offer personalised insurance products that cater specifically to the customer’s requirements.
Corporate finance
Machine learning solutions can be widely implemented by data scientists in corporate finance as they are perfect for demand forecasting, as well as anomaly and error detection. This can be used to highlight transactions that have been made in error or potentially violate accounting policies. These models forecast the percentage of completion metrics such as hours, cost, and units, to predict POC revenue and the total completion effort remaining. Algorithms can be also used to forecast when customers will pay invoices, triggering proactive collection efforts before payments are past due.
Risk management and fraud
Banks and financial institutions can use models to determine whether a client would be a good candidate for a loan by using the available financial data to determine if a customer is likely to default on it. Such processes can significantly improve loan application processing efficiency and accuracy. Machine learning automates risk scoring and financial monitoring, which also improves decision-making and increases accuracy in risk and portfolio management in financial markets.
Fraud and abuse are major problems for banking institutions, accounting for billions of dollars in losses each year. ML algorithms can scan through large data sets to detect unique activities or anomalies and flag them to users for further investigation.
Trends, forecasts, and predictions
Machine learning also assists in building online applications that provide automated financial advice to investors, making portfolio management seamless and easy. Machine learning can assign categories to transactions affecting customers’ bank accounts, while AI learns how to categorize transactions based on the title of the transfer, its originator, or recipient.
Companies can also use models to automatically predict stock prices. The models are provided with vast amounts of historical data on a company’s stock and then used to extract key trends and essential features that define the stock performance. If the parameters, features, and trends are extracted effectively, the model can accurately predict future stock prices and performance, enabling it to make smart trading decisions.
In the near future, algorithms will have an even more accurate picture of what will work and what won’t, based on the vast amounts of historic data they will possess. AI-powered applications will undoubtedly continue to flood the market, providing more value at a faster pace than traditional approaches.