In Open Data We Trust?
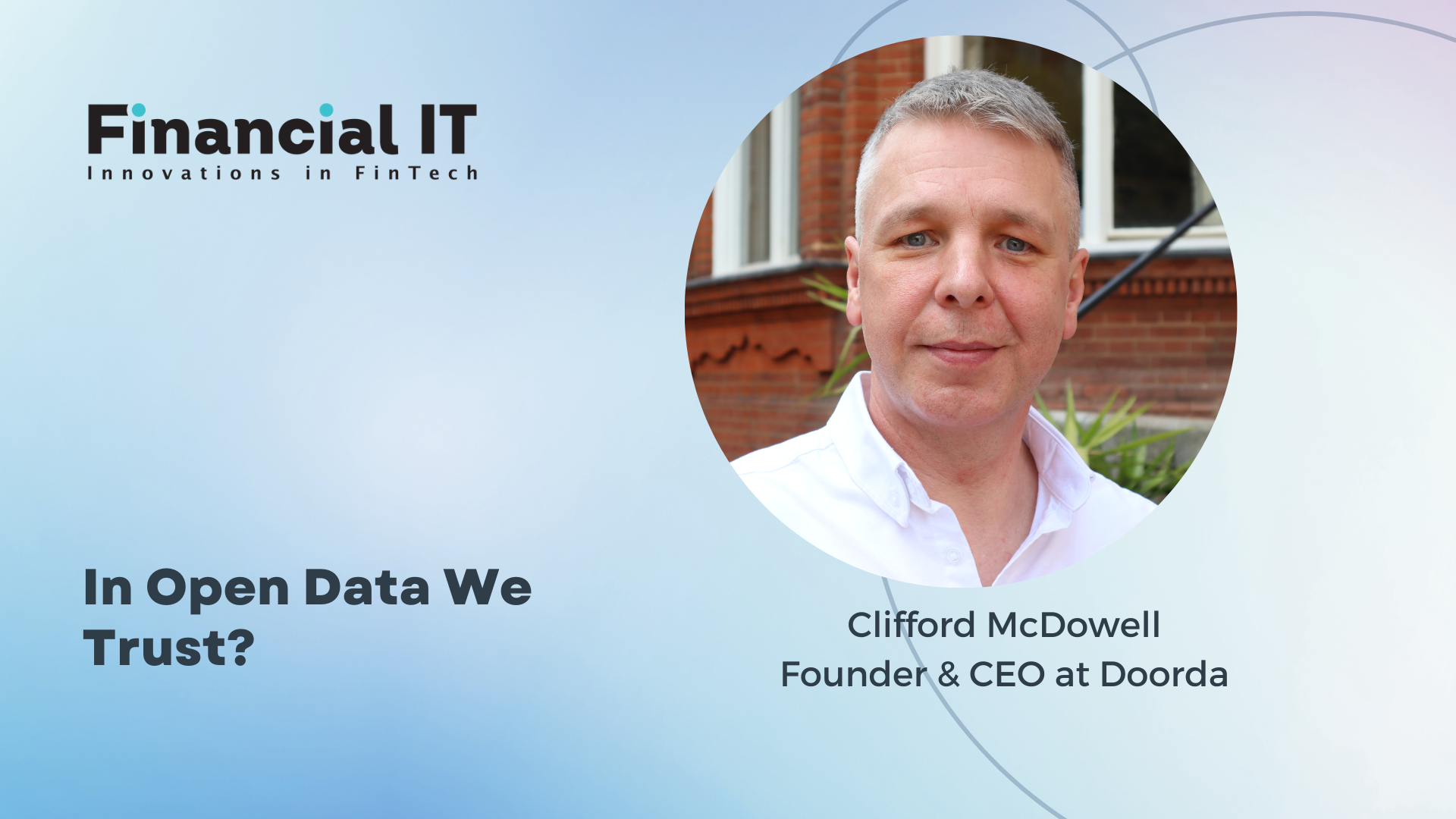
- Clifford McDowell, Founder & CEO at Doorda
- 19.07.2023 02:00 pm #data
Data scientists, in their desire for precise knowledge, are faced with a conundrum when it comes to Open Data published by official bodies – because it must be included, yet it is often not in a fit state for purpose.
It has been claimed that poor data quality leads to poor decisions, but while this may be the case for a company’s own internal data, this is an over-simplification of a complex situation – especially in the case where data is obtained from outside sources.
Data governance frameworks and data quality processes need to include valuable Open Data resources in order to have a complete picture in order to make good business decisions, but in reality that data is often equivalent to unrefined ore rather than sparkly ready-to-use gems.
Open Data – by which I mean public data sets around non-personal information related to market trends, demographics, companies or properties – can be freely used, re-used and redistributed by anyone subject only to the requirements to attribute and share.
The quality of Open Data from an official source will not usually be 100%. This is because of the method in which Open Data, even for a national system of reference such as Companies House, is collected. But even when flawed there is often no better source and, despite its quality, value can still be obtained.
Making the most of Open Data
To derive value, we need to be able to objectively trust external sources by augmenting data governance processes associated with the curation of the data. Indeed, for the US Department of Commerce, “structuring the data and tracing the source are just two of many important aspects of data governance that are carefully considered.”
Ideally, a data governance framework will be able to judge open datasets by understanding if there is a problem with the data and quantifying the extent of this problem, at the same time as identifying the source of that data. Importantly, the concept of the data source includes both the provenance and the dates when the data was created, or updated and harvested.
At the point of use, the data must be assessed with respect to its intended use. How it is utilised can then be adjusted - by giving it a lower weighting in a predictive model, for example, or by altering the algorithm.
Essentially, gaining a critical understanding of Open Data, and developing a data framework accordingly, involves the following three crucial areas:
Data provenance: Ensuring datasets are obtained directly from an official source or data publisher, and have not been filled-in, corrected or altered in any way
Freshness of data capture: An important amount of information can be gained by looking at the metadata of a data source. For example, business rates of 2023 vs. 2022.
Data Quality: The ability to quantify the missing or invalid values, quantify the missing records, and identify the inconsistent values and links to other data, etc.
If there are known issues with Open Data, especially around inconsistencies which might be due to differing extraction dates, the data can be linked and combined with other internal and external datasets to obtain a better consensus picture. Of course this requires the aforementioned knowledge to trigger the activity.
“Any job worth doing…”
Open Data is not perfect, but it does contain enormous value. Performing data governance and data quality is by far a task worth undertaking in order to establish the right level of trust in the data, for whatever application.
By establishing the details of provenance, quality and timing through correctly curating and formatting Open Data, firms can use it with confidence, contributing to incredible insights.