Data Management Platforms as the ‘building Blocks’ That Inform Risk Strategies
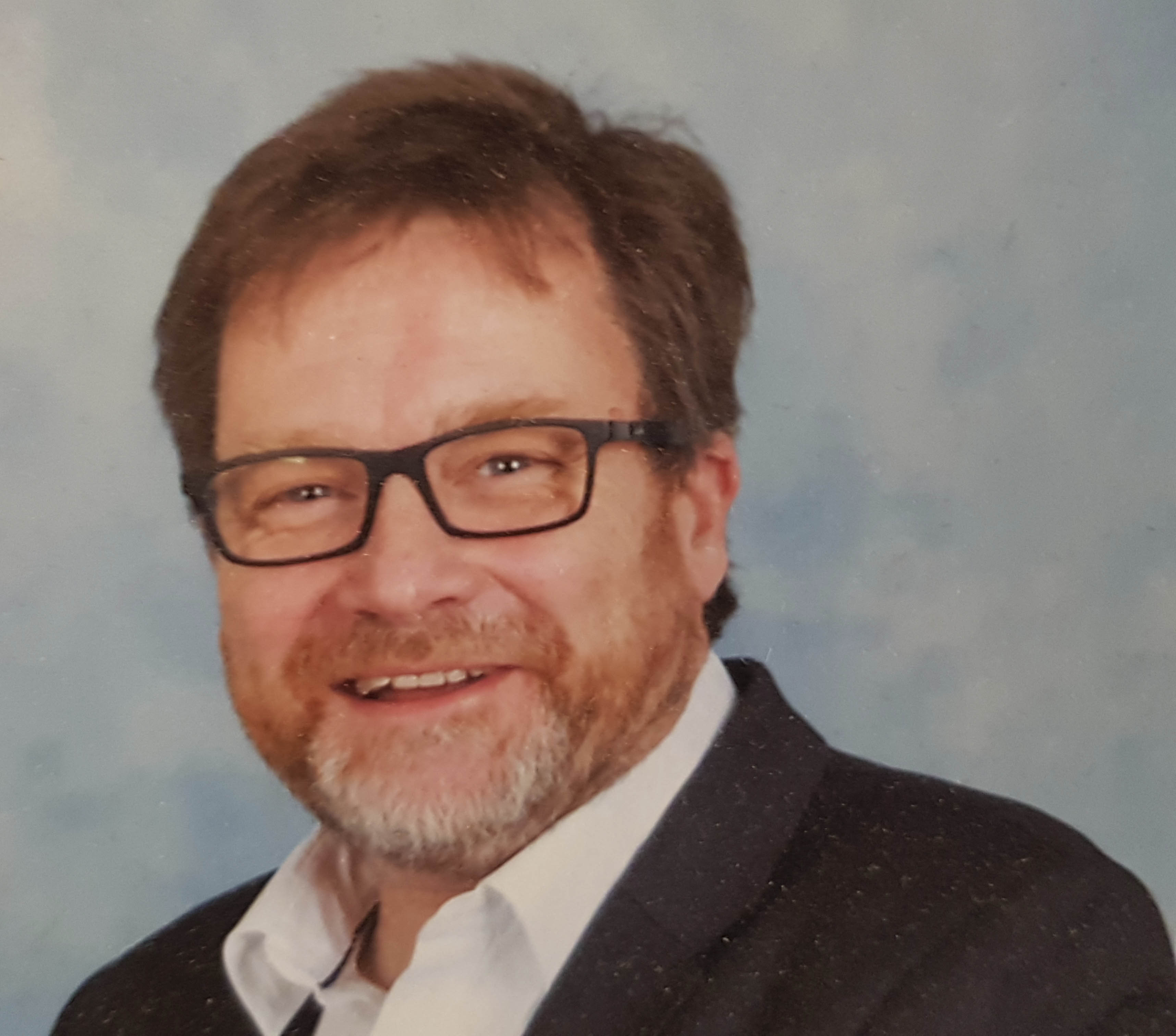
- Ian Pestell, Senior Data Scientist at TIBCO Software
- 10.09.2020 02:45 pm data
Data speed and scale require real-time analytics and accurate predictions for financial trading companies
Stock markets are the natural homes of big data, and with modern market data sets being so vast and generated so rapidly, they now require real-time analysis to inform actions. Take, for example, businesses that need to understand their liquidity position in the market; a single traditional data platform is simply unable to provide all the features and functions necessary to provide end-to-end business value. A more effective approach is to use a set of ‘building blocks’ on which to build a system of insights that provides organisations with deep, actionable analytics and predictions.
Data analytics and predictive analysis are especially applicable to risk management in investment banking, brokerage and asset management. Here, organisations are constantly trying to understand liquidity trading risks for stocks (and other asset types) held in multiple positions. Getting a clear picture of these financial assets, underlying instruments and transaction types, is a big data challenge - especially when considering different asset types such as equity, FX, bonds and derivatives.
To understand risk, traders must have the latest information on regulatory environments, compliance and the roles and interconnections of different counterparties. This complexity comprises a set of real-time data management challenges that require numerous technologies for data streaming, large-scale high-performance databases, master data management, data virtualisation, visual analytics, and data science.
The tasks to be executed are also vast and include data preparation, data management, creating federated virtual views to build models, using data streams to tie it all together with visual analytics, and the application of data science for predictability.
Technology to understand investment risk
While the regulatory environment might be complex and the assets vast and varied, technology should take centre stage in helping to understand and quantify risk.
When looking at trading oversight operations, real-time risk control, corporate risk compliance and execution, the challenge for organisations is the need to understand and report their liquidity position. They will want to be able to move in and out of positions for lots of different stocks with different profiles, while assessing market volatility. For example, where a player holds multiple positions in a thinly traded stock, it can’t be simply dumped, as this is likely to impact the market price.
Indeed, a strategy is needed to liquidate stock over a period of time, which may be days, weeks or even a financial quarter. Such a strategy is best executed using the best features of various different technologies.
Any trading position involves dealing with market data, which is both real time and historical, and comes from multiple sources. A trading company will have portfolio data on what is held, along with who is trading and when, which also comes from multiple sources. There is also the huge volume of trading data coming from the market – from information providers and many other sources – in real time.
It is only by using the most suitable features from data management ‘building blocks’ that companies can stay better informed on market conditions, understand their market position and calculate and analyse the risks associated with decisions.
The building blocks for success
As with many data science challenges, when applying a data strategy to trading risk, a clear building block approach makes sense.
- Data at rest. Trading data is, by its nature, a set of real-time events. To support deeper analytics, this data needs to be at rest. The data begins its journey by being moved into a high-performance database, combining streaming, interactive analytics and artificial intelligence. It is in a memory-optimised database ingests data streams to support the query of the real-time data.
- Data virtualisation. Data virtualisation provides the mechanism for combining at rest trading data with market data, historical data and business rules. This provides 360o views of the data for down stream analytics and modelling.
- Master data management (MDM). A master data management platform is used for governing, understanding and consuming all shared assets, including the master data, reference data, hierarchies and metadata. As well as allowing for management of the assets, this provides security guidance to the data virtualization layer to control access to the data..
- Data science platform. Against the 360 data views, risk models can be created and evaluated using the data from multiple locations. Using data science machine learning and feature engineering, models can be created and evaluated. Trained models can be then injected back into the live streams to provide real-time risk assessment or scoring of trading data.
- Visualisation. Visualisation tools create real insight into patterns and behaviours. Visualisation can, for example, surface trading hotspots. Visual analytics is a key ‘building block’ when backed with an AI engine, while a visualisation platform that also applies analytics creates intuitive understanding of positions and trades.
It’s essential to remember that anything involving data sets at scale runs the risk of slowing systems down, so it is important where possible to avoid moving multi-terabyte and petabyte sized workloads from multiple sources around the network. Technologies that use sourcing, such as data virtualisation and management, should ensure that calculations are delegated down to the source database to avoid performance problems.
Real time risk
More data is moving around financial markets at a greater pace than ever before, yet traditional monolithic data management platforms are often restricted to handling static or batch data.
In a financial trade, determining risk in real time is the key to success or failure. For success, the ability to control, manage and ultimately exploit the knowledge in the data, through analysis and prediction, is often best achieved through this ‘building block’ approach to data management challenges.