Challenges of Feeding Data Hungry AI Models
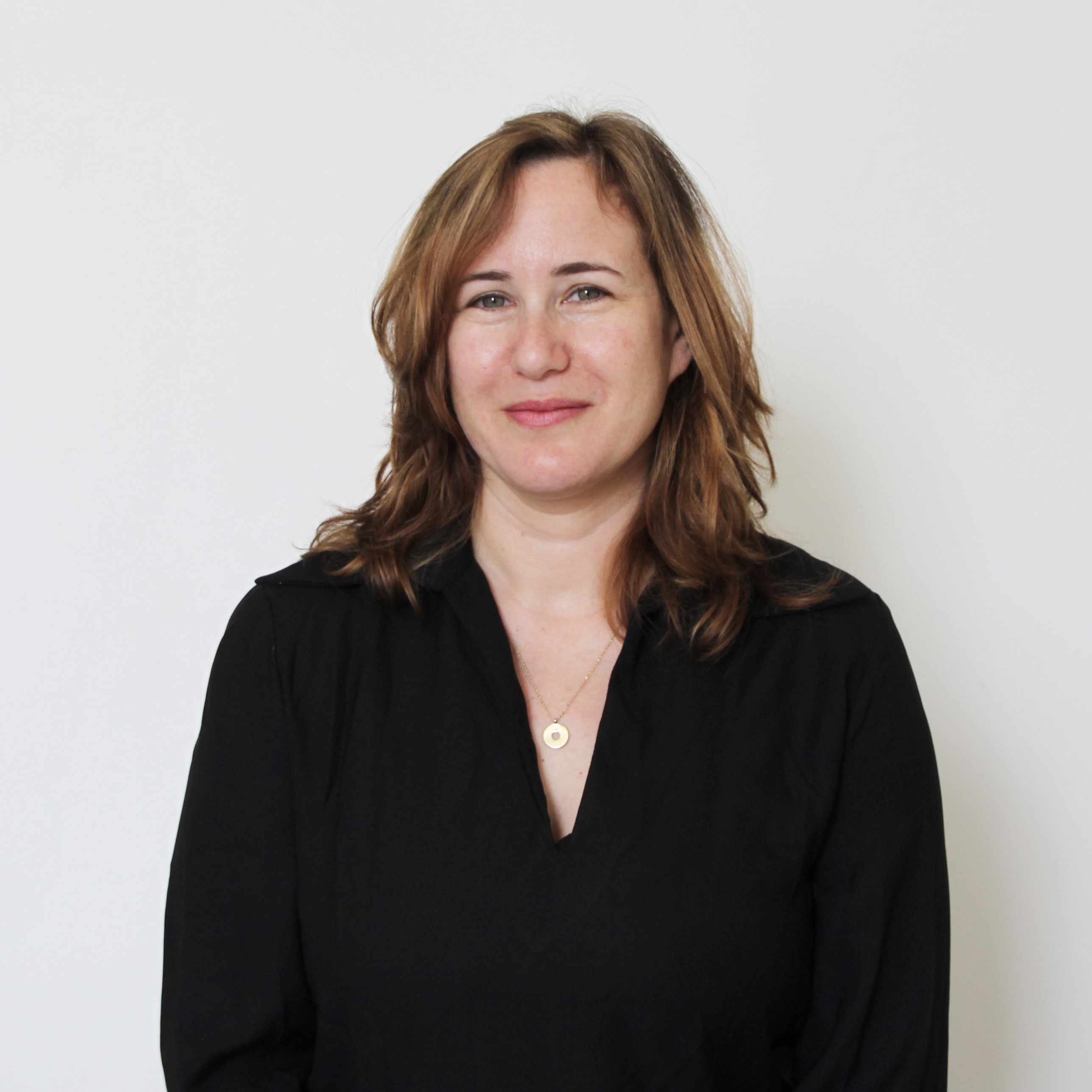
- Sigal Shaked, CTO at Datomize
- 13.04.2021 06:30 pm data
Financial institutions are more dependent on AI models for every aspect of the enterprise, including limiting risk, preventing fraud, and making hyper-personalized offers based on a customer’s last interactions. However, according to a McKinsey survey, 24 out of 100 organizations stated that the biggest barrier to AI implementation is the lack of usable and relevant data.
AI models are starved for data
To provide a steady stream of meaningful insights, AI models need lots of data. In general, the more data you feed, the more accurate are the results.
There are enormous datasets that are very common in the financial services industry, like transactions, customers, bills, money transfers, etc. However, regulations are putting huge limitations on how personal data can be obtained and used, including the General Data Protection Regulation (GDPR) and the Payment Card Industry Data Security Standard (PCI DSS).
For financial institutions that rely on legacy systems built before AI became a reality, the problem can be as simple as the fact that data is not available. A bank’s information is often stored in separate data stores, data lakes, or data warehouses for different business units, geographical locations, and technology teams.
Data that is available can be missing key populations required to see the complete picture. If that data tells a skewed or incomplete story, the rules it creates will be fundamentally unsound. There is also the classic problem of ensuring that the data has integrity. Even if the data is safe, accessible, and representative of every segment of the population, it can still be unusable because it’s incomplete, irrelevant, or out of date.
How to build a data pipeline
One way to break data silos is to build a data collector that will harvest data from different sources. However, this data may be unusable due to regulations, so it will have to be anonymized. There are techniques such as data generalization, pseudo-anonymization, and data masking, but these methods can often be duped by hackers putting an organization at risk of leaking sensitive data.
Another option is for enterprises to generate safe synthetic data sets. Synthetic data can also be used to fill the gaps where information is lacking, so that data models are unbiased, accurate, and complete. New technologies such as Generative Adversarial Models (GAN) have increased the accuracy of synthetic data, making it more cost-efficient and quicker than data cleaning.
In the financial world, AI models are becoming an essential part of keeping relevant. But with companies racing ahead to streamline operations, identify new revenue streams, and provide engaging customer experiences, a data drought can put a massive obstacle in their path. By creating a healthy pipeline of quality data, the quality and wisdom of the insights gleaned by AI models can become a reality.