Entity v process: The collective power of AI
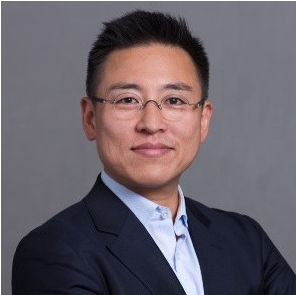
- Huw Kwon , Vice President and Global Head of AI at Cognizant
- 25.03.2020 08:00 am AI , Fintech , Huw Kwon is Global Head of Artificial Intelligence for the AI and Analytics Practise at Cognizant. Huw previously worked for McKinsey where he led global AI projects and advised C-Suite clients across industries as an Associate Partner. Prior to McKinsey he led Data Science and Analytics CoEs at Accenture, EY and Lloyds Banking Group. At Cognizant, Huw will lead our offering in Europe across Data Modernisation, Customer Intelligence and Operational Intelligence. He also sits on the Global AIA leadership team and provides leadership at a global level for the development of our intelligence offering and strategy.
Most, if not all, financial institutions are exploring how the range of new and emerging technologies collectively known as artificial intelligence (AI) can improve their performance across a range of activities and processes. AI offers methods and algorithms to solve various optimisation problems as efficiently as possible, that is, to close the loop in the system and achieve superior control of the phenomenon at hand. It is no wonder there continues to be keen interest in the field, despite the hype curve tailing off.
Research by Cognizant[1] shows that 74% of financial services executives said AI was extremely or very important to the success of their companies today, while 53% said it would be extremely important in three years’ time. The emphasis on the importance of AI is more pronounced in financial services than in other industry sectors. That’s because financial services possesses one of the key ingredients to make AI successful: plentiful sets of rich, labelled data.
However, while the enthusiasm for AI is clear, there’s less evidence of real progress on the ground. Only 29% of executives reported that AI projects were in full implementation. The majority say that AI initiatives were at the pilot, proof of concept. These often generated improvements that, while positive, were not enough to justify swapping them with existing methods or services such as customer lifecycle management models, NBA engines, credit scoring modules, pricing, etc
Such data-driven services in banks have traditionally been entity-based (whether that is a customer as an entity, or a firm, or a portfolio). This, in essence, is a data snapshot taken at a specific time, whether that is predicting a customer default, the sale of a loan or cross-selling of an insurance product.
AI and the customer as an entity
AI advances that banks and financial institutions are making today focus on the customer experience. Chatbots deploying natural language processing (NLP) offer customers 24/7 access to virtual agents that can provide routine account information such as checking account balances and requesting new products. Machine learning, a key AI component, can recognise patterns and continue to learn from experiences, feedback and interactions to improve the decisions and recommendations it makes. While this can be applied to accelerate and increase the accuracy of credit decisions or fraud detection, such systems and methods already exist and the AI plays more on the incremental opportunities. These areas are, in most banks, relatively mature and likely to be only marginally improved rather than through seismic innovation.
In customer analytics, greater improvements using AI (Deep Learning algorithms in particular) offer enhanced scope for personalising services, upselling and cross-selling, as well as recommending next best actions on the fly. Like the digital natives’ use of similar methods, these can help drive revenue growth and go beyond the bank’s existing NBA engines, which are more often than not based on old business rules.
Yet, there is an area that remains to date largely unexplored. That is the way in which AI and data analytics can effect dramatic improvements in operational efficiency by looking at process.
From RPA to Operational Intelligence
Many banks have deployed Robotic Process Automation (RPA) to address discrete and well-defined processes (which can be expressed as a logical flow diagram in most cases). These include document management in areas such as underwriting or product applications and some automation of call centre activities. Coupled with high accuracy character recognition, RPA makes it possible to automate a variety of mundane, time-consuming tasks that used to take hundreds of work hours and inflate payrolls.
Employing RPA for high-frequency repetitive tasks eliminates the room for human error and allows a financial institution to refocus workforce efforts on processes that require human involvement. However, despite these benefits, RPA remains relatively limited in its scope and impact.
Beyond automation
AI, coupled with its technology enablers, now offers the chance to go much deeper and capture the true picture of operations, providing intelligence and insight that was not available, and do so at lightning speed. This process insight will transform financial institutions’ understanding of complex, end-to-end processes. That will enable them to take actions and make changes that can have a materially significant impact on costs. And at a time when many banks have ‘squeezed the juice’ out of optimising and restructuring to improve their cost-income ratios, the appeal of true, data-driven process improvement is self-evident
Operational intelligence covers everything that is process-driven . And, after all, what isn’t a process? Aside from the simplest business processes, banks have found it near impossible to see in detail how most operational activities really work end-to-end. A logical model of A-Z operations – or what the business thinks those are - describes in theory what should be happening across operational processes.
Even when operational processes are documented in rigorous detail, it remains impossible to identify the dynamic or even chaotic delays and manual interventions that drive costs up and increase complexity. However, in an increasingly digitised environment, where every interaction with an app, writing of an email, search, telephone call, request ticket raised, every click of a mouse or stroke of a keyboard, leaves a trace, it is feasible to capture every single element of every process.
A vast wealth of such process data is now available to reveal the detailed inner-workings of an end-to-end process. These are by their nature dynamic and varied, which paints a very different picture from the logical process diagram banks are used to but which are far from reality and in which ample potential improvements lie hidden.
From complexity to insight
Of course, without the tools to understand what all that data shows, it would simply represent a highly detailed record of massive complexity. But advances in big data analytics, process-mining AI capabilities make it possible to assess the logical model of how an entire operational process is supposed to work against a true, data-driven representation of actual performance.
This process-data-driven reality shows the enormous complexity of what is really happening. The question is, what can be done with that to start making significant improvements that will enable real gains in operational efficiency? Using the computer processing power and algorithms that we now have available, it’s possible to take the highly complex process journeys that the data reveals, consolidate them, and identify where problems are in order to address them and transform performance.
Using AI to create operational intelligence offers a game-changing ability to isolate a specific point in the execution of a process journey. The data analysis is able to show exactly why a problem is happening – for example, the length of a delay, which department or even individual made an intervention. And as the picture improves over time, it’s possible to gauge the impact of improvements. AI’s ability to create a new monitoring capability, removes the guesswork from introducing process improvements. Now is the time to rely on machine learning and AI with real-time, click-by-click, second by second process-data-driven analysis of banking operations that can reveal significant opportunities for measurable improvements.
Data at the heart
At the heart of operational intelligence is data. In fact, data is at the heart of all AI initiatives. More than that, having the right data in the appropriate form is a fundamental but all too easily overlooked prerequisite for success with AI. It’s an issue that financial services executives are aware of. Forty-five percent of them said that getting access to accurate and/or timely data was very or extremely challenging for their companies in deploying AI. Data engineering is the hidden, yet most important, element of any AI or digital work. Everything that banks want to do must be built on the basis of modernised data.
It’s essential that data engineering links directly to business value. AI initiatives often take a top-down strategically-driven approach. All too often, that results in a pilot or proof of concept that is unable to scale into production. In contrast, a bottom-up, process-data-driven approach connects the people, processes and technology that can deliver real AI-led value at scale. It does this this by bringing together all the right talents and capabilities – from data engineering, platform technology, data science, UX/UI designer and the business – all in an agile team with a shared objective.
People before technology
Having the right data foundation in place is essential. In reality, that often means democratising data to a wider audience of users (ie by moving to the cloud) and it’s a change that many may find uncomfortable. Overcoming their resistance will require support for re-education into the ‘new’, which is key to sustained success especially in larger organisations.
That is a key aspect of any AI initiative. The change it creates has to be seen in human, rather than technological, terms. An effective AI strategy therefore requires a human-centric approach. Forty percent of executives say that one of the AI challenges they face is the need to retrain employees, and 38% say that the ability of employees to interact effectively with AI applications is a further challenge. Banks need to be ready to reskill employees to work in an AI-powered organisation. And with 50% of financial services executives citing access to talent as the biggest AI-related challenge they face, financial institutions must be prepared to reshape their organisational cultures to attract and retain sought-after skills and capabilities. This can include broadening roles, pushing decision-making authority to the individual contributor level, and building attractive environments that enable the adoption of AI.
To find out more about Cognizant’s AI capabilities and POV contact Huw at Huw.Kwon@cognizant.com or visit https://www.cognizant.com/operations-two-point-zero
[1] Cognizant AI research of 230 financial executives in the US and Europe.