Riding Out the Storm: How AI and ML Can Make Banks More Resilient
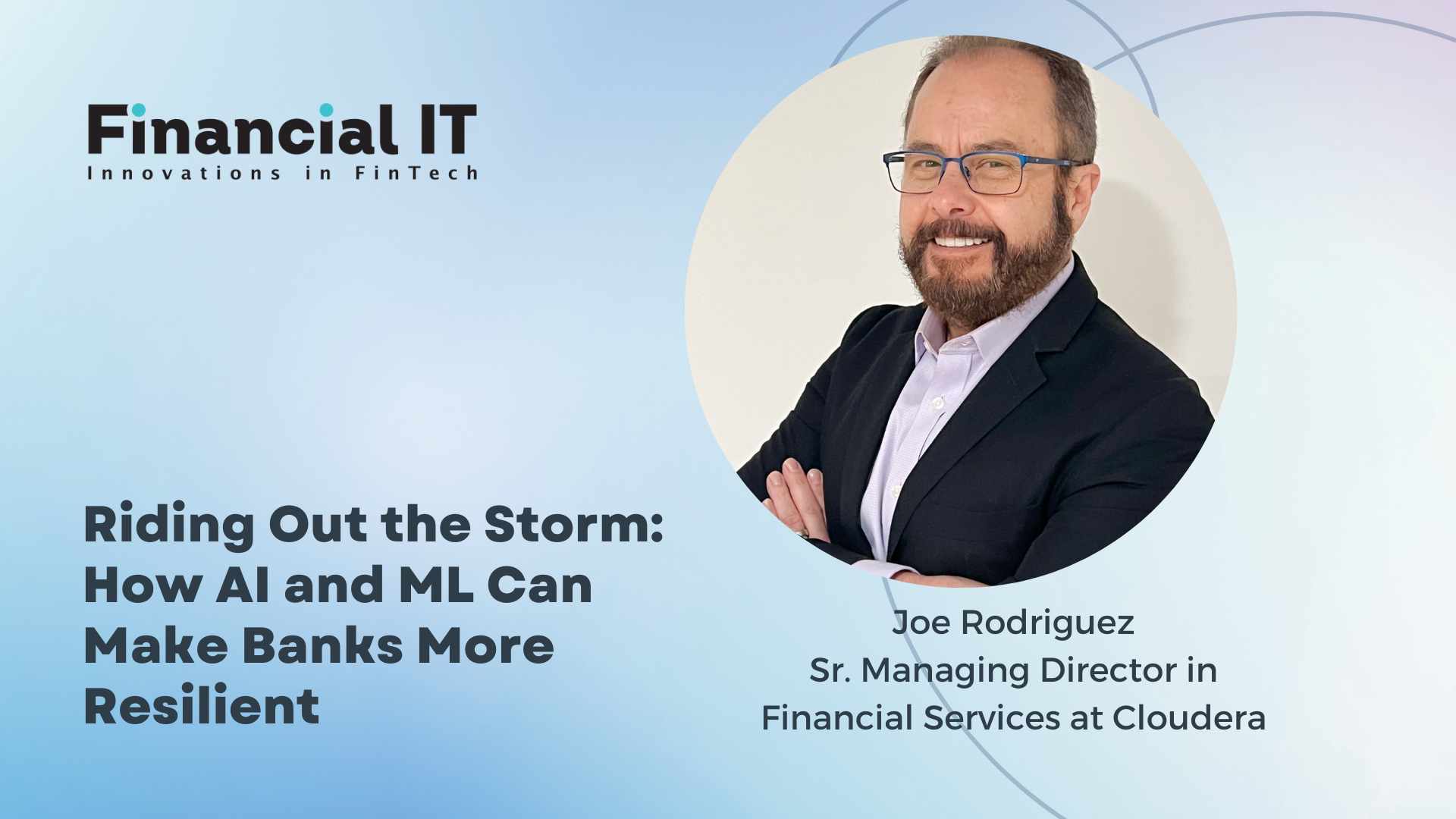
- Joe Rodriguez, Sr. Managing Director in Financial Services at Cloudera
- 18.08.2023 12:15 pm #ai #ml #banks
The notable failures of regional banks in the US, such as Silicon Valley Bank (SVB) and First Republic, have acted as a warning to financial institutions across the globe. Rapid increases in interest rates, unstable funding, and turbulent economic conditions are just some of the factors that led to recent banking upheaval and the collapse of SVB.
And while technology alone could not have prevented the banking crisis in the US, many banks across the world are still failing to leverage technology as innovatively and cost-effectively as they should be. For instance, to ensure the stability of the banking system, advanced liquidity risk models and stress testing using artificial intelligence and machine learning (AI/ML) could potentially serve as a protective measure.
To improve risk management, financial institutions need to modernise their data management and data governance practices by implementing a modern data architecture. This will make it possible to break down legacy data silos, simplify data management, governance, and integration – all whilst decreasing costs and increasing resilience.
Technology’s role in resilience
Previously, financial institutions could not accurately forecast and manage liquidity risk because of technological limitations. Now, due to the increasing maturity of machine learning, it is easier for institutions to analyse data at scale. Institutions can then layer this analysis with AI to automatically identify problems and initiate pre-labelled remediations in real-time.
For example, by incorporating data from new sources – such as social media feeds, geospatial systems or IoT streams – financial institutions can support liquidity monitoring and forecasting in real-time. If financial institutions can identify trends or events that could potentially impact liquidity using AI/ML, they can take pre-emptive action to manage risk.
Banks can also use predictive analytics and ML to formalise critical liquidity metrics. This enhances counterparty risk assessments and enables them to observe liquidity positions in real-time. Institutions can also use these techniques to design forecasting models that are more accurate at predicting intraday cash flows. Furthermore, by adopting real-time analytic dashboards that incorporate data from payment systems and other sources, financial enterprises can deliver the appropriate data to various stakeholders in a simple interface.
ML techniques can also be adopted to realistically model and simulate stress scenarios internally. Sophisticated ML models can simulate known credit, market and liquidity risks in various stress scenarios and embed them into existing risk-management processes. Banks can then design scenarios to automatically govern and manage this lifecycle and develop alerts whenever risk levels change or exceed certain thresholds.
Giving AI and ML the tools to do the job
The benefits of these innovative approaches are clear, but there is one common thread that links them all if banks are to realise those gains – a reliance on data. Currently, as most financial institutions lack a modern data architecture, they are unable to manage, integrate and analyse financial data at pace. This is holding them back. In fact, our recent survey revealed that banks are not able to use a third (33%) of their data effectively.
By addressing this issue, they can responsibly and cost-effectively apply AI/ML to processes like liquidity risk management and stress-testing, transforming their ability to manage risk of any kind. Through implementing a unified data platform, banks can use AI/ML to analyse data from across on-premises and cloud. This gives them a complete overview of data over every core process and system – from loan portfolios to financial market data. By deploying this modern data architecture and giving AI and ML the opportunity to drive value from data, no matter where it resides, banks will become more resilient to external forces.
Riding out the crisis
The tools to help banks to effectively manage risk, govern and integrate data and scale across on-premises and cloud environments are available. But failing to modernise and deploy AI and ML will be a missed opportunity, as these technologies have the power to completely transform risk analysis.
Those banks that are able to harness the power of AI and ML to streamline risk management will be better placed to ride out the turbulent economic landscape. Those that do not use AI and ML to become more resilient will be on the back foot, and risk falling behind at a time where they can’t afford to do so.