The Importance of Artificial Intelligence in Receivables and Credit Management
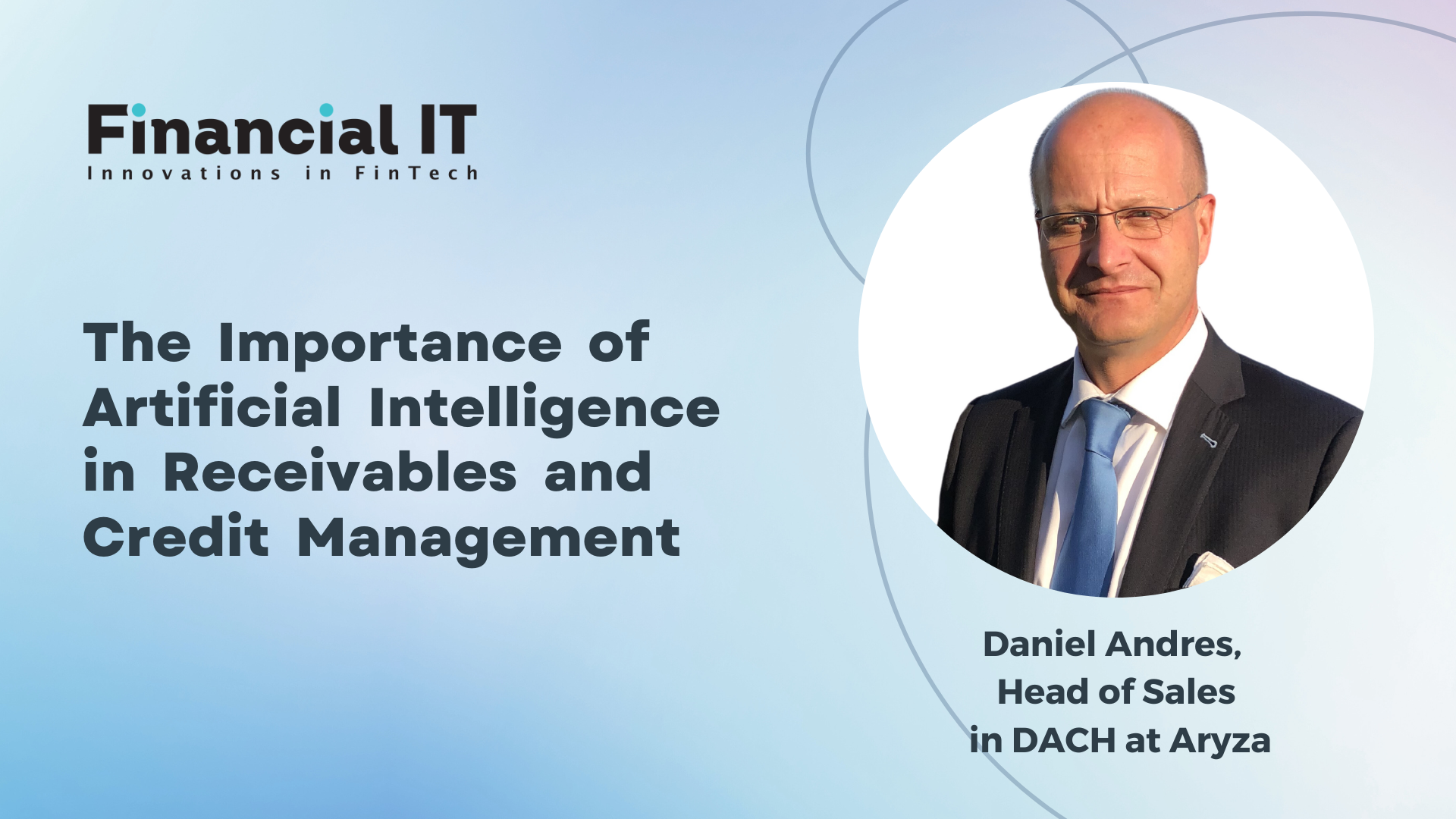
- Daniel Andres, Head of Sales in DACH at Aryza
- 24.07.2024 01:30 pm #riskmanagement #AI #regtech #data
The digital revolution, fueled by artificial intelligence and big data, is reshaping the landscape of receivables and credit management in the DACH region. As fintech companies and payment service providers introduce innovative payment and collection methods, traditional financial services must adapt or risk obsolescence. This transformation, driven by customer-centric services and data analytics, offers greater efficiency, effectiveness, and an enhanced user experience.
AI Utilisation in Receivables Management
AI's integration into receivables management marks a significant shift. By leveraging empirical default data, AI improves scoring models, leading to fewer defaults and more accurate risk pricing. Machine learning techniques enhance these models further, ensuring robust development and precise backtesting. This results in better scoring and stricter risk management, crucial for lenders and financial service providers aiming to thrive in the digital age.
Moreover, AI optimises the reconciliation and forwarding of payments to customer accounts, streamlining debtor processing. This not only improves operational efficiency but also elevates customer service, creating a seamless experience for end-users.
Enhancing Customer-Centric Services with AI and Big Data
The digital revolution prioritises customer-centric service models. By harnessing AI and big data, companies can conduct comprehensive data analyses to gain valuable customer insights.
This enables predictive tracking of spending patterns and account balances, facilitating more targeted product sales. Additionally, AI-driven debtor segmentation allows for precise targeting, reducing wastage and adapting services to specific customer needs.
For instance, AI can assign debtors to different segments based on their behaviour and risk profile through the debt segmentation model. This ensures tailored treatment, enhancing the amortisation rate and minimising costs, illustrating how AI can customise interactions to maximise repayment potential. The challenge lies in doing this accurately and automatically. The debtor segmentation model categories include:
True Low-Risk: Use the least experienced agents with set scripts, guided by on-screen instructions to handle the likelihood of broken promises.
Absent-Minded: Use interactive voice applications, saving time and reassigning agents to more difficult customers.
Dialer-Based: Match agents to customers and provide live instructions to customise scripts, increasing connection and payment likelihood.
True High-Touch: Focus on customers able to pay but with a high risk of non-payment, addressing higher failure rates in this segment.
Unable to Cure: Offer early restructuring or payment plans for customers in financial distress, increasing the chance of partial debt recovery.
Using the debtor segmentation model to predict the optimal treatment for a fixed set of debtors can maximise repayments. Integrating confidence intervals and estimating the costs for mailings or calls within the operational strategy allows for precise resource allocation and improved outcomes.
AI-Driven Innovations in the Credit Lifecycle
AI's applications span the entire credit lifecycle, from scoring and matching to monitoring and communication. In scoring, AI uses empirical default data to refine models, ensuring better scoring accuracy. Matching involves AI-optimised reconciliation of payments, enhancing debtor processing efficiency. Monitoring employs predictive analytics to anticipate future customer needs, enabling targeted sales efforts.
Communication, a critical aspect of receivables management, benefits immensely from AI. AI-supported selection and optimisation of communication channels and times ensure efficient and effective interactions. Additionally, chatbots facilitate routine customer service and account management, providing a more efficient self-service option for debtors.
The Role of AI in Enhancing Efficiency and Effectiveness
AI promises significant improvements in efficiency and effectiveness across receivables management. Automation in workflows and optimal resource allocation reduce costs and streamline operations.
Recommendations for optimal payment plans, based on AI-based segmentation and transaction data analysis, boost collection performance.
For example, predictive analytics can determine the optimal rate for payment plans, improving recovery rates. AI-driven negotiation and automatic creation of these plans ensure a more efficient accounts receivable service. Furthermore, intelligent routing assists call centre agents in handling specific cases, enhancing cash collection efficiency.
The Future of Receivables Management in DACH
As fintechs challenge traditional financial services, the integration of AI and big data becomes imperative. The digital revolution offers lenders and financial service providers in the DACH region an opportunity to transform their operations. By embracing AI-driven innovations, these companies can enhance customer experiences, streamline processes, and achieve greater efficiency and effectiveness.
The potential for AI in receivables and credit management is vast. From improving scoring models and optimising payment reconciliation to predictive monitoring and targeted communication,
AI offers a comprehensive toolkit for modernising financial services. As the DACH region continues to evolve, embracing these technologies will be crucial for staying competitive and meeting the demands of an increasingly digital marketplace.