AI in Financial Services: Harnessing Emerging Trends to Unlock Opportunities
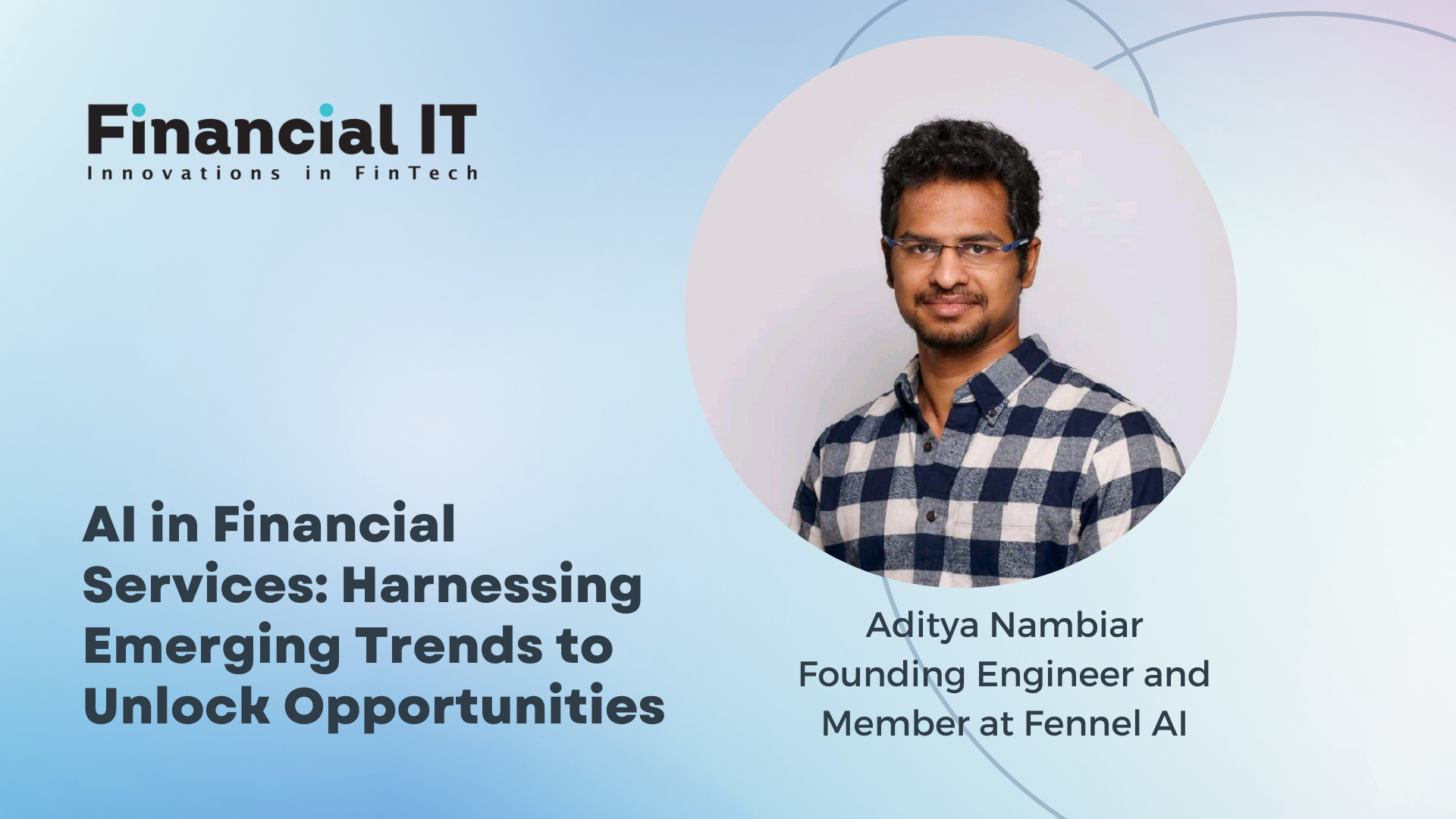
- Aditya Nambiar, Founding Engineer and Member at Fennel AI
- 07.08.2023 12:15 pm #ai #financialservices
In the rapidly evolving landscape of the 21st century, artificial intelligence (AI) has emerged as a game-changing technology and the financial sector is no exception. Over the past few years, AI has transitioned from a nice-to-have technology to a cornerstone of innovative financial solutions, shaping how we manage money, process transactions, and interact with financial services. This article aims to journey into the heart of this transformative movement, focusing on the two big emerging trends within AI - namely Generative AI and Real-time Machine Learning (ML) - and how they hold the keys to unlocking a treasure trove of new opportunities in the financial sector. Let's dive deeper into this exciting confluence of finance and AI and explore the possibilities it brings.
AI's Role Across Financial Domains
AI's potential to transform the financial landscape is already evident in several areas:
Fraud Detection: The ability to analyze vast datasets aids in pinpointing anomalies and suspicious activities that may indicate fraud. Machine learning algorithms can learn from each transaction, continuously improving their detection capabilities and reducing false positives.
Risk Assessment and Management: Predictive capabilities make artificial intelligence an essential tool for risk assessment. Lenders can leverage AI to analyze applicants' data to predict their creditworthiness more accurately, reducing the risk of default.
Personalized Financial Products and Services: By leveraging data analysis and predictive capabilities, financial institutions can create personalized financial products that cater to customers' specific needs and financial goals.
Operational Efficiency: Automation can streamline numerous back-office functions in financial services. Tasks such as document verification, data entry, and routine customer service can be automated with AI, freeing up human employees for more complex tasks.
Dynamic Pricing: Machine learning algorithms are used to adjust pricing strategies based on a wide array of factors. These can include demand, supply, time of day, customer behavior, and market conditions.
Having established the present impact of AI, let’s delve deeper into how emerging trends in AI, namely Generative AI and Real-time ML, can unlock opportunities previously unattainable.
Generative AI: Creating New Realities
Generative AI is a subset of artificial intelligence that leverages machine learning models to create new data that resembles the input data it was trained on. This capability to generate novel and coherent content - text, images, or audio - sets it apart in the realm of AI. Generative AI is powered by very large models (known as Large Language Models or LLMs) that are pre-trained on vast amounts of data and are commonly referred to as foundation models (FMs).
Within the financial services sector, industry leaders and engineers are keen to uncover the capabilities of generative AI and harness its power. Let’s look at how Generative AI can be helpful in the financial sector.
Synthetic Training Data Generation: Privacy concerns and data security regulations often limit the availability of financial data for AI training. Generative AI can create synthetic financial data, replicating real-world data's statistical properties without exposing sensitive information. This allows for robust model training and testing without compromising privacy or risking actual assets. Additionally, it paves the way for enterprises to cooperate without the danger of disclosing internally sensitive user data.
Enhanced Automation: Generative AI can further help the automation of various financial tasks. For instance, AI can generate comprehensive financial reports or summaries from raw data, reducing the time and effort spent on manual data interpretation and report writing. It could also be used to automate the generation of personalized financial advice or market predictions, increasing efficiency and accuracy. For instance, Bloomberg recently launched BloombergGPT, which can perform tasks such as semantic analysis and answering questions based on research reports.
Multi-modal Understanding: By processing and integrating data from different modes - text, images, and sound - generative AI can provide a more holistic understanding of a situation. In finance, this could involve correlating news articles, social media sentiments, and market trends to predict market movements more accurately.
Chatbots and Virtual Assistants: One of the biggest shifts introduced by Generative AI is that text is now the new interface. Previously, product users or engineers needed intricate user interfaces and workflows to use a financial tool. Now, these can be streamlined and replaced with a straightforward chat interface. A virtual agent, powered by generative AI, can comprehend a user's query, source pertinent data, and deliver a thorough, personalized response. This capability has the potential to greatly enhance customer service, creating a more individualized and efficient user experience. For eg JP Morgan recently rolled out an AI-powered virtual assistant that will make it easier for corporate clients to move money around the world,
Simulation of Economic Scenarios: Generative AI can be used to create a wide range of potential economic scenarios. By simulating different market conditions and analyzing the potential impact on investment portfolios, financial institutions can make more informed decisions, improving risk management.
Real-time Machine Learning: Swift Decisions, Enhanced Results
Another trend significantly reshaping the AI landscape is the increased corporate investment in Real-time Machine Learning (ML). But what is Real-time ML? Real-time machine learning uses real-time data to generate more accurate predictions and adapt models to changing environments.
This typically involves deploying ML models in production environments and using a real-time feature engineering platform instead of relying on batch predictions. At its most advanced stage, it takes the form of an online learning system where the model is continuously trained on incoming data.
Such an approach brings immense value in scenarios where patterns undergo rapid changes and swift decision-making is required. With the escalating trend of digital payments, Real-time ML has evolved into an integral and revolutionary component of the finance industry.
Let’s look at some of the primary advantages of Real- time ML in the finance sector -
Rapid Data Generation and Processing: The financial sector generates enormous volumes of data daily, from stock prices to transaction details. Processing and understanding this data promptly is critical for making sound financial decisions. Real-time ML models are designed to ingest, process, and analyze data as soon as it is generated. This swift data processing enables financial institutions to glean valuable insights in real time, offering a cutting-edge advantage in a sector where timing can make a significant difference.
Dynamic Decision-Making: Real-time ML allows for dynamic decision-making based on real-time data. For instance, in trading, it can quickly react to changes in market conditions, adjusting strategies instantaneously. This speed and adaptability can provide a significant edge in maximizing returns and minimizing losses. In contrast, conventional rule-based systems and static fraud detection models frequently fall behind, unable to keep up with the increasingly sophisticated methods employed by fraudsters.
Financial Monitoring: Real-time ML plays a critical role in financial monitoring, particularly in fraud detection and anti-money laundering measures. The system can immediately flag suspicious activity by analyzing real-time transactions, helping prevent fraud and maintain regulatory compliance. You now need systems that work at low latency but at the same time provide high throughput to meet the growing demands of the rise of digital payments.
Similarly, in asset management, real-time ML can continuously monitor market trends and generate alerts when significant changes might affect portfolio performance.
Cold Start: The cold start problem refers to the challenge an ML model faces in making accurate predictions when it encounters new users or items with little to no historical data. Real-time ML can help mitigate this problem in finance by rapidly learning from new data as it becomes available, allowing it to provide valuable insights even in the absence of historical data, which could be critical in use cases such as predicting credit risk.
Experimentation Velocity: Success in AI depends on the speed of iteration. Realtime ML can significantly accelerate the rate of experimentation in finance compared to traditional batch-based models. Because it can quickly adapt to new data, financial institutions can test different strategies or models in real-time and iterate on them based on immediate feedback. This rapid experimentation can drive innovation and improve financial products and services.
As we stand on the cusp of the new era of financial services, the transformative power of AI technologies like generative AI and real-time machine learning cannot be overstated. These advancements are not only meeting existing industry challenges head-on but are also unveiling opportunities that were previously out of reach. Integrating these technologies involves a maze of complexities, but the prospect of a more streamlined, innovative, and customer-focused financial ecosystem makes this venture unquestionably valuable.
As we chart the future of finance, one thing is clear - AI is not just an accompaniment; it is the torchbearer leading the path. Anticipate a future where financial processes are more efficient, decisions are data-driven and real-time, and services are personalized as never before. The future of finance looks bright, and AI is leading the way.