Data as a Service: Discovering Value from Financial Services Data Silos
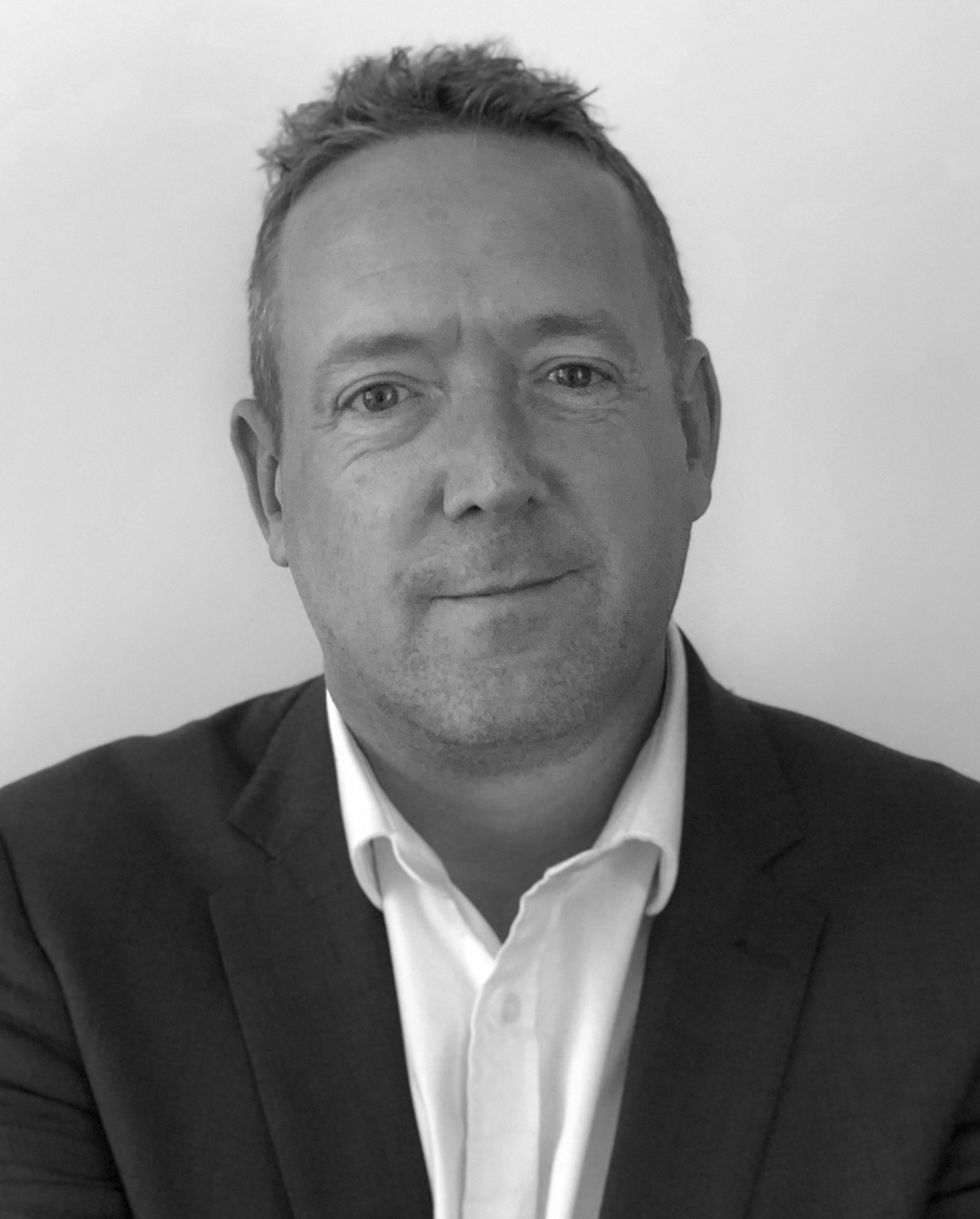
- Simon Rayfield, Head of Operations at Alveo
- 12.01.2023 02:15 pm #data
The financial services market continues on a growth trajectory and is expected to breach $37.34 trillion in 2026 with compound annual growth (CAGR) at 9.6%, according to The Business Research Company. Accompanying this growth is a tsunami of data, which accumulates as a by-product of everyday business activities. To harness its benefits, financial services firms must understand how they can best work the data they possess and how they can translate it into a big-picture view that serves as a tool for better-informed management decisions and more effective operations.
At its core, the financial services industry has always been about information. Customers today require more detailed information than ever, whether from asset servicers, banks, or asset managers. Regulators too require more granular reporting. Examples of this include more detail behind valuation for banks and mutual funds, performance of investment products as well as the ESG characteristics of investment products and loan portfolios. Other requirements pertain to the tracking and tracing of data flows, both for the protection of personal information as well as for the tracing of the lineage of information: understanding a data point’s origins before it flows into a workflow such as a risk model or regulatory disclosure.
Most banks struggle to capitalise on the competitive advantages their data sets provide, according to the 2020 World Retail Banking Report by Capgemini and the European Financial Management Association, with only a few able to manage, let alone leverage, their most valuable and actionable datasets.
Financial services firms can no longer afford to take a reactive approach to data collection and data curation but need to proactively find ways to effectively provision business users and the application landscape with the data required. This includes slashing the time to onboard and operationalise new data sources and the effort required for “last-mile integration”: anchoring the data into decision-making workflows.
A piecemeal and decentralised approach to data management evidenced by the persistence of data silos, data tucked away in applications or isolated in hard-to-access data warehouses, complicates firms realising the value inherent in their getting company data.
According to a recent Vena Industry Benchmark survey, 57% of business leaders, finance executives and operations professionals reported that multiple disparate and disconnected data sources remain a key data challenge. When data is trapped across multiple instances and across different databases, then operational efficiency is impacted, and can hamper innovation, external reporting and client interaction. Different silos create a need for aggregation and introduce ambiguity and the need for unnecessary reconciliation. This problem is most acute for enterprise functions such as finance, risk and external reporting. Harnessing data from across silos provides opportunities for financial services firms to keep and sustain their competitive edge and enhance customer experience.
Finding a solution that works
Financial services firms must therefore focus on integrating existing workflows across different departments and providing them with consistent, high-quality data. That’s where we see a growing role for Data-as-a-Service (DaaS): offerings where solutions providers use cloud technology to deliver aggregated, cross-referenced and quality-vetted data sets in different formats and delivery methods to speed up onboarding. Using DaaS is a data management strategy that introduces flexibility, transparency, fast integration and that enables firms to quickly “put data to use” and maximise its ROI. DaaS puts companies on a solid data foundation and can help break down siloed information and be an expert data service bureau to the entire organisation.
DaaS can cover any dataset including corporate actions, security master, issuer and pricing data and can range from use-case specific offers to providing data services at an enterprise level. Data collection and curation typically includes the tracking of quality metrics, the cross-referencing and verification of different sources, the set-up of business rules for data quality and data derivation and root-cause analysis on gaps and errors. Cleansed and fully prepared data then feeds into a customer’s operations, risk management, performance management and compliance.
DaaS enables easy access to data and the swift onboarding of new data consumers or new external reporting requirements. It includes cross-referenced identifiers; data lineage and other metadata including quality characteristics and can assist financial service providers to develop personalised customer experiences with predictive analytics to understand consumer behaviour and patterns.
The best solutions make data simple to visualise and manage and help provide easy access to help firms make the most of their data assets. They empower users with a real-time view of data, including its acquisition, distribution and delivery to downstream applications, along with data lineage capabilities and a summary of completed and running processes. A DaaS solution provides transparency as to the provenance of data as well as indicators on data quality and remediation. DaaS solutions give users the flexibility to request changes to the service such as additional or different data sources, new delivery formats, changes to the data in scope, integration with their cloud data warehouses and its delivery frequency.
DaaS solutions are a logical progression from managed services, which embrace hosting and application management but usually exclude data operations and quality management. Specialist companies with domain expertise in integrating financial sources present a clear value proposition. Through utilising this expertise, financial services firms benefit from shorter change cycles, improved data quality and greater transparency into the collection and verification processes, which combined with quality metrics across diverse data sets leads to a step-change improvement in data operations.