Transparent technology: How machine learning brings magic to next-generation PFM
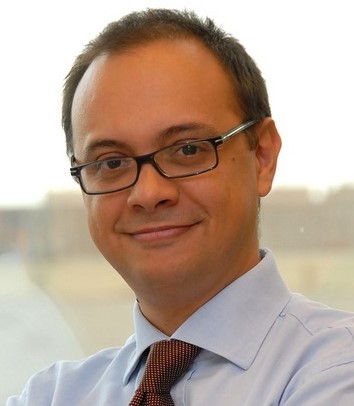
- Pau Velando, Charge of Channel Partnerships Management at Strands
- 20.11.2015 12:00 am undisclosed
Personal Financial Management (PFM) tools have become a very convenient solution for individuals and businesses to get a holistic view of their transactions and overall financial situation. It aggregates information from our transactions and allows us to see where we spent our money, the value of our assets and what liabilities we have. But those are just basic functionalities of first-generation PFM solutions.
Next-generation PFM goes a lot further: when logged into online banking, you'll see your expenses automatically categorized, your expected cash flow for the coming weeks and even your financial performance against your peer group, even if you have not defined it.
So what's actually behind all of these next-level insights?
TANGIBLE INSIGHTS = END USER DELIGHTS
On the surface, Machine Learning (ML) algorithms seem to bring a kind of "magic" to analyze and display PFM users' financial activities and profiles. As these algorithms work in the background, they constantly "learn" patterns that are used to autonomously forecast income and expenses, categorize transactions, simulate financial scenarios or recommend actions that are in the user's best financial interest. |
Another feature that also contributes to the smarter use of PFM is the possibility to aggregate accounts from different institutions and its transactions, products and services. At this point the solution shows even deeper insights when it classifies, predicts or recommends based on data coming from another institution. |
All these events undoubtly bring some magic to the experience of the user when interacting with her online banking, but also some trepidation wondering how the system knows this or suggests that - especially if it comes from outside the banking environment. Thankfully, today we have enough tools to make ML algorithms work in ways that protect personal information and respect users' privacy.
For the user, the benefits of ML embedded technology outweigh the drawbacks of volunteering their data (which, of course is always consensual and highly secure in an online banking context). The algorithms work in the background, transparently, analyzing the data generated by the activities of thousands or millions of users as they use banking services. The end results bring wider and deeper insights that can help end users to manage their finances more intelligently, with significantly less manual effort. |
DRIVING OF THE DIGITAL TRANSFORMATION IN BANKING
Not only does ML technology bring magic to the end user, but also to the bank. ML-powered financial services can uncover a host of powerful insights revealing exactly how the customer interacts with the bank, from spending patterns to purchase behavior, saving appetite and even investment acumen.
When applied to financial services, machine learning algorithms can uncover new spending patterns among its pool of clients, indicate previously unknown forms of interaction, discover new segments of customers and unlock new insights within the bank's omnichannel efforts. |
PFM solutions that embed cutting-edge ML capabilities suddenly become a key driver of digital banking transformation, impacting every channel from web to mobile, from branch offices and even to the bank's back office with products like recommendation engines or card-linked marketing tools.
As tantalizing as it sounds, paramount to all of this must be the user's privacy rights. Personal data protection is essential and needs to be communicated thoroughly in Terms and Conditions and strictly respected at all times. But how can analyzing customer data, cross-examining spending patterns against peer groups and even generating personalized offers be done in a way that does not violate customer privacy?
To meet this essential requirement, advanced analytic algorithms must be trained with anonymized data, meaning that the pool of transactions and demographic attributes that are often used to build the learning of the systems need to be pre-processed so that it does not contain any data that can be used to identify individuals.
THE MACHINE LEARNING FUTURE OF PFM
Next-generation PFM solutions have embedded machine learning algorithms that go far beyond the simple reporting of transactional data. Seamlessly integrated within the backend of core banking systems, ML technology works to bring insights to directly to both end users and banks in a secure and transparent way.
For users, it can help them discover spending patterns, compare themselves to groups of people with similar financial profiles. ML solutions can also help banks to better understand how their customers interact with their products and services.
If banks want to leverage the large amounts of data at their disposal, embedding machine learning within their digital offering is an extremely powerful step forward. The reward for both banks and users is obtaining deeper insights and behaviours faster and better than otherwise possible via traditional methods.