Lenders bet on AI for credit scoring
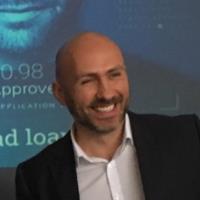
- Dmitry Dolgorukov , CEO at GiniMachine
- 14.08.2018 02:00 pm undisclosed
Banks have to struggle with a lot of challenges – from issuing credit to operational risks, technological troubles to good old fashion fraud. In addition to the risks of yesteryear, modern banks face falling long-term rates, growing fintech competition and low profitability. In this challenging environment, savvy modern banks focus more of their attention to mitigating risks.
Chief among these challenges are low performing loan portfolios which are a constant thorn in the side of lenders. For example, European non-performing loans stand above €1 trillion with more than one third of banks having NPL ratios above 10% (ECB, 2017).
This minefield of factors has driven lenders to seek out new ways to increase profits and cut funding costs in order to stay competitive.
AI in Fintech: Will it take over?
“AI is a powerful tool for banks thanks to its ability to harness vast quantities of data to learn more about customer patterns and behaviors”, says Steve Ellis, head of the innovation group at Wells Fargo.
As powerful as AI is, traditional banking is still heavily reliant on statistical methods that were developed over half a century ago. Lenders determine creditworthiness based on 20+ data points, which leave otherwise worthy customers behind.
Modern machine learning makes it possible to go much deeper when analyzing data, and allows lenders to extract valuable insights from available data patterns.
According to the McKinsey report, a number of European banks have already replaced the antiquated statistical-modeling approach with machine-learning techniques. The results speak for themselves: a 10% increase in the sale of new products, 20% savings in capital expenditures and a 20% decline in churn.
The data doesn’t lie: lenders are betting on AI. Evidence of this modern trend can be seen in numerous ‘banks and fintech collaborations’ and AI-based software releases:
- JPMorgan Chase pioneered a Contract Intelligence platform designed to “analyze legal documents and extract important data points.”
American MobileBank deploys AI software to lend to thin-file millennials.
Canadian TD Bank uses Layer 6’s AI engine for scoring and cybersecurity.
Deutsche Bank came out with new AI-based equities to predict their pricing and volume more accurately.
Wells Fargo employs its own AI team to provide more personalized services and strengthen digital offerings.
Bank of America Merrill Lynch implements HighRadius' AI solution to speed up receivables reconciliation for their large business clients.
Logistic regression is no longer the de facto standard
Nine times out of ten, logistic regression is used to build scoring models and solve classification issues. Before it can take over and provide predictive results, there’s an important step of preliminary analysis and data quality control that must be taken. If the dataset contains:
imperfect and missing values, outliers and unstructured data;
numerical and categorical values (age, income vs marital status, education);
raw data that doesn’t fit strict parameters(data with fractions or decimals etc.)
data analysts will spend days (if not weeks) just to preprocess the data before it can be assessed. Cutting corners and ignoring such data may lead to the loss of valuable insight and incorrect predictions.
How modern AI/ML methods build better risk models
Today, lenders have the ability to collect more data than ever about their clients. In addition to traditional socio-demographic data, this may include transactional data, records from credit bureaus, social media, Google Analytics as well as other non-traditional sources.
Processing and interpreting this data so that it can be used to issue loans to worthy credit-seekers is where modern ML/AI methods give banks the edge they need.
Machine learning techniques like gradient boosting, random forest or neural networks can better find hidden dependencies in a dataset, which helps to gain more accurate predictions. This assists banks in determining how collected parameters in a dataset should be weighed to predict whether borrowers will consistently repay their loans on time.
This is made possible by data signals, which define significant parameters that affect the power of a scoring model. Depending on the type of business, geography, target audience and data authenticity, significant parameters may differ. Modern ML can determine which data points contain the desired signal.
Traditional data sources like credit bureaus still remain an important part of the process and provide the data that contain the above-mentioned signal. Unfortunately, they do not cover noteworthy market segments such as millennials, self-employed entrepreneurs, small business owners, immigrants or the unbanked.
For instance, my team at GiniMachine carried out pilot projects to build scoring models with minimal data points and without access to applicant’s credit history. Some of the most promising predictive parameters included - the applicant’s industry and occupation, the size of their company, the total years they’d been in business, the size of their family, and data from social networks like their overall activity, and the quantity and quality of their connections.
Modern ML methods can build more accurate risk models because of their capacity to:
use built-in ‘raw’ data preprocessing tools
find hidden dependencies of arbitrary complexity
harness unstructured, big data and data from alternative sources
The financial world and lending business in particular have seen major changes throughout the last few years. Using ML and AI in concert with traditional practices is the way forward for banks that want to remain competitive in the modern world. It’s clear that making good loans to the people of the future requires a futuristic helping hand.