Financial Services and Generative AI: Approaching with Optimism
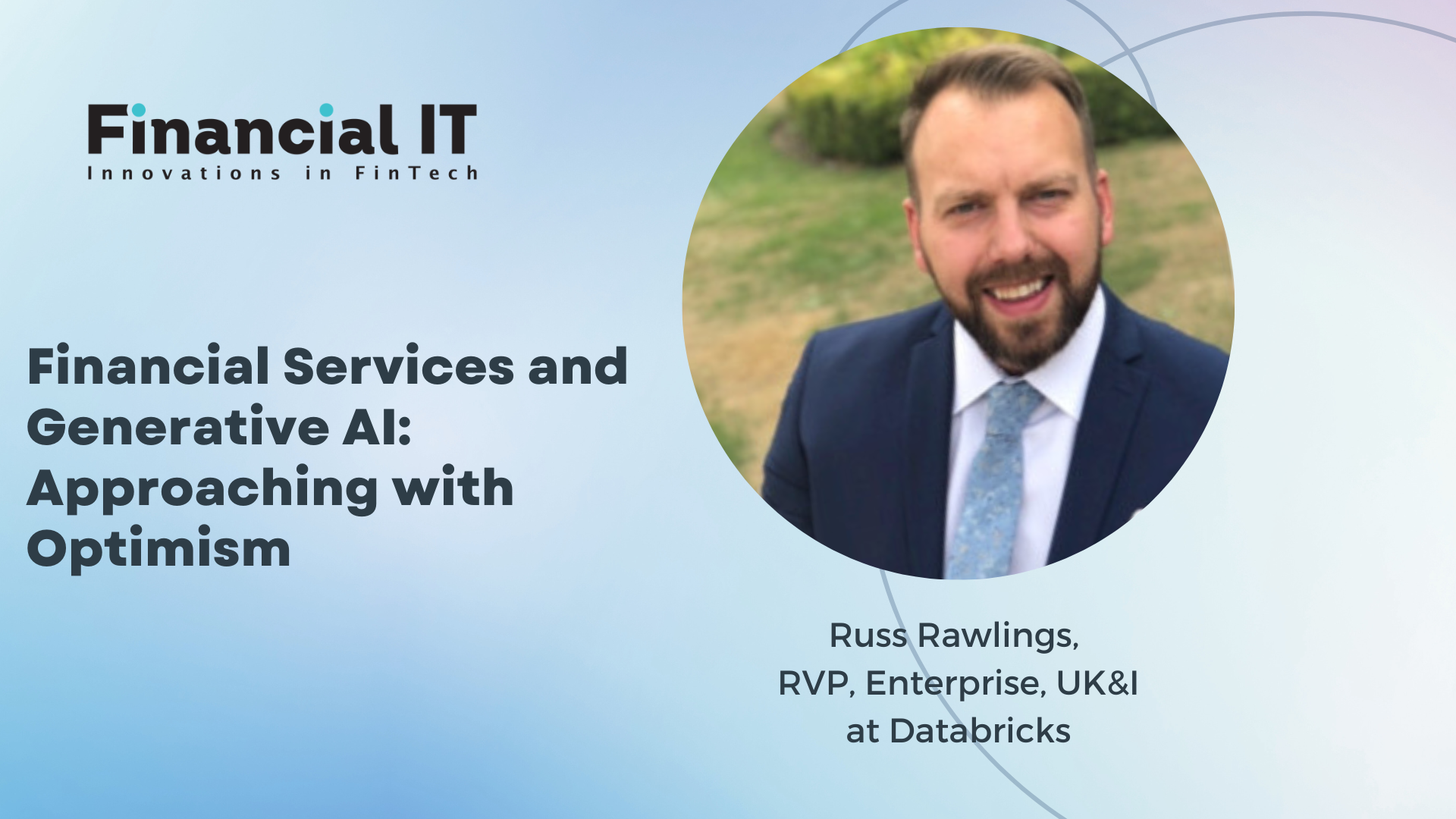
- Russ Rawlings, RVP, Enterprise, UK&I at Databricks
- 22.05.2024 02:30 pm #GenAI #AI #LLM #data
Historically, the financial services sector has adopted technology at a slower rate, often due to the industry's highly regulated nature and the time and cost needed to implement a new IT solution. But if organisations have a customised large language model (LLM) in place, there is a real opportunity to maximise the value of their data to improve internal processes and evolve customer experiences.
Organisations from Deloitte to IBM are predicting that generative AI will hugely impact the sector in 2024. Moreover, a recent survey found that 68% of the industry’s leaders believe that having a platform that enables the adoption of emerging technologies is of high importance. But between training a model on the right data, complying with privacy regulations, and creating a data culture, enterprises have a lot to factor into their generative AI adoption roadmap.
The benefits of a customised open source LLM
When it comes to adopting generative AI, selecting the right size model is crucial. LLMs have been at the forefront of the wider AI conversation for a while, famously powering many consumer chatbots. But financial enterprises need a model that is tailored to their specific business needs, such as determining risk, minimising fraud, or providing more personalised experiences. This is where custom open source models can be of value.
Such models can be created for a specific use case, and are reasoned on an enterprise’s proprietary data, making them more cost-effective to run, and capable of producing more relevant and accurate results. For instance, a model can analyse a consumer’s buying behaviour and flag any suspicious or fraudulent actions. Or, it can leverage specific algorithms in conjunction with a consumer’s data to determine if they’re eligible for a loan. General-purpose models, on the other hand, are trained on a much larger dataset, often composed of data scraped from the web. This can include irrelevant or poor-quality data, which could generate less pertinent outcomes. Moreover, a general model is at higher risk of becoming “contaminated” and hallucinating - which can have disastrous consequences for the organisation.
With this in mind, another key consideration is security. Since financial institutions work in such a highly regulated industry, deploying a custom open-source model can encourage them to experiment with generative AI, and unlock its benefits, whilst ensuring governance. They can control what data goes into building their model to help them adhere to regulatory requirements, and by deploying their own in-house LLM, they won’t risk the possibility of having to share their data with a third party. On a broader level, this approach democratises the training of custom LLMs and allows every organisation to extract the most value out of a model that is powered by their own private data.
Maximising impact with data intelligence
Generative AI will create an even bigger impact if financial services organisations establish a strong data culture, and build data intelligence. All employees, regardless of technical acumen, now need to confidently understand everything from how data is stored, to how it’s used, to how they can leverage it to improve decision-making and innovation. Not to mention, the finance sector must comply with more intensive legislation compared to other industries, and thus require a particularly rigorous data governance strategy.
To meet these requirements, enterprises can look to a data intelligence platform. Built on a lakehouse architecture, a data intelligence platform provides an open, unified foundation for all data and governance, and operates as a secure end-to-end solution. The platform is trained on internal data and concepts, which is crucial for financial services; the model’s understanding of technical jargon will ensure accurate and relevant responses. Users can input requests in natural language, and the data intelligence platform uses generative AI to deliver highly relevant responses. This means that all employees, including non-technical teams, have the ability to query the enterprise’s data to pull insights. Aside from empowering their workforces, banks won’t need to leverage a third party solution for data analysis, facilitating a smoother approach to governance and accelerating data-driven outcomes.
The future of financial services
No two financial institutions are exactly the same, but there are broader requirements that the industry’s tools must meet. Striking a balance between supporting specific uses whilst considering wider needs requires targeted solutions that will best facilitate innovation. Customised, open source LLMs, and data intelligence platforms, will do exactly that - and can spark large-scale industry transformation.