Is Agent Based Simulation the Window into our Chaotic World?
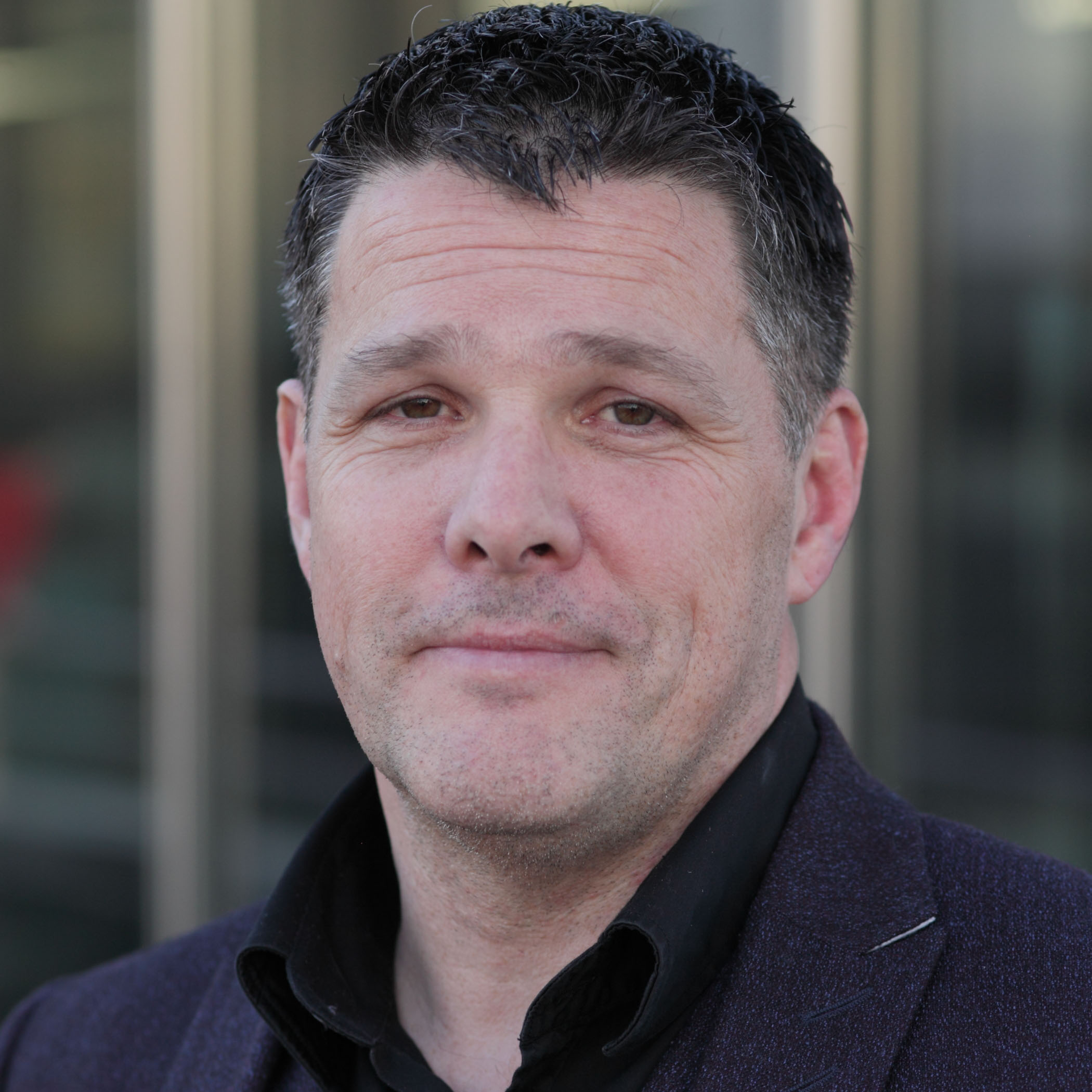
- Craig Beddis, Chief Executive Officer & Co-Founder at Hadean
- 27.04.2021 05:30 pm financial market
Financial markets become more and more unpredictable by the day. How do you account for a ship blocking the Suez Canal? What could prepare you for a global pandemic, emerging markets or Redditors taking on Wall Street? Among these new external factors, we are seeing the hugely positive drive towards greater financial inclusion; from modern banks reducing transaction fees and encouraging the flow of cash across borders, through to disruptive startups using big data to redesign lending models and risk assessment.
The cumulative effect of all of this is a seismic change in the agents at play. The number of individuals, companies and systems which can influence markets is growing, and with it, the frequency of emergent factors. Emergence occurs when an entity holds a property only through the sum of its parts. A classic example in nature may be the overall intelligence conveyed by an ant colony, despite the ants being simple creatures on their own. Seeing one action on its own would not allow you to understand how the eventual property emerged, only when seeing them altogether is this possible.
The concept can also be used to help explain unusual outcomes relating to game theory, including ones that seem to have a counter intuitive logic to them. The Braess Paradox for example, is an observation concerning traffic described by the German mathematician Dietrich Braess. Intuitively, it would seem a way to decrease traffic on a road would be to add another lane. However, what actually occurs when this happens is that people are able to swap lanes more in order to try and get ahead, slowing down the traffic even more. How people behave on an individual level collectively causes a counterproductive result. This effect has been shown with numerous real world examples, where the flow of traffic has actually been improved by removing roads. Emergence can help explain how prices are set in a free market, how clerical mistakes can result in huge losses and even how the actions of individual Reddit users can bring down hedge funds.
Creating Agent-Based Models
Often financial modelling techniques, such as regression analysis, work by taking particular macroeconomic factors and equations and then applying them to the situation. In this sense, they are ‘top down’ methods and are often unsuccessful when applied to emergence. Agent-based modelling, in contrast, works by starting from the bottom and analyses the actions of individual entities. In this sense, it is far better suited to tackling emergent phenomena, due to them always finding their origin in individual actions.
Agent-based models analyse large data sets from multiple disparate sources - data from social media, stock prices and supply chain information needs to be collated and synthesised into single, large-scale simulations. The unpredictable nature of its volume and velocity results in highly volatile demand for computational resources. For financial modelling, a system that can dynamically scale and handle these surges in computation will be key in encouraging widespread adoption of agent-based simulation. The current architecture is ill-equipped, restricting the scale and detail of simulations. At the same time, large data sets cannot be effectively aggregated, limiting scope and accuracy.
Moreover, such setups present a high barrier to entry both in terms of cost and accessibility. As it stands, creating and deploying models is a complex engineering feat that requires constant maintenance and management. As workloads continue to migrate to the cloud, there is a significant risk of expensive overprovisioning or the alternative whereby the underlying infrastructure is overwhelmed and crashes. By contrast if infrastructure concerns are automatically taken care of, much of the engineering burden is alleviated. Smaller, agile teams can create repeatable simulations where variables are adjusted in order to find the optimal strategy. The real-world information proving more meaningful than abstract estimations.
The new agents at play have changed financial forecasting for good. They have created a chaotic environment where the emergent effects can only be understood through complex simulation of their individual actions. Modelling these interactions will be a crucial mission for computation and require infrastructure equipped for massive scale and fidelity.