How Big Data and AI are Shaping Innovations in Fintech
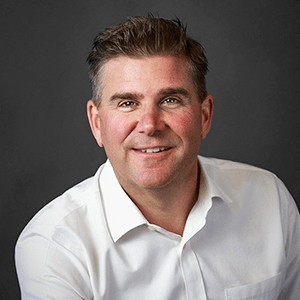
- Dave Mariani, Co-Founder and CTO at AtScale
- 01.11.2022 05:15 am #data
Recent technological advances in big data, artificial intelligence (AI), and machine learning (ML) have opened new opportunities for fintech firms. With new capabilities, fintech teams can speed innovation and increase focus on how to enhance the customer experience and more.
Big data is a big advantage for fintech companies and financial institutions – more so when non-technical business users can access data and use it in their day-to-day work. According to a report from IBM, 71% of banking and financial markets firms say the use of information and analytics is giving them a competitive advantage, a 97% increase compared to 2010. And Morgan Stanley has dubbed the 2020s as the data decade, where the evolution of computing will be driven by leveraging internal data.
In this article, we will look at how fintech companies can apply data science and a semantic layer to speed innovations and fuel their growth via self-service analytics. We will also highlight how one fintech company, Trumid, is using advanced analytics and data science capabilities to help facilitate self-service trading.
Big Data in Finance
Data is useful both for understanding “what happened” and “what’s to come” and can be broken into three categories:
Reporting: Reporting answers questions about what happened. This includes performance reports for external audiences or compliance requirements and internal reporting about customer behaviour.
Analytics: Analytics drives into the higher-order questions about how or why something happened. For example, suppose you release a new product or feature. Has it changed customer behaviour?
AI/ML: Machine learning (ML) techniques can leverage big data to make predictions. Since it addresses unknown unknowns, machine learning is a tool for data scientists to address open-ended problems. For example: how can you leverage data to make smarter decisions or improve customer experience?
Forward-looking financial firms are investing in all three of these use cases for big data. USAA partnered with Finicity to facilitate reporting in Intuit products without needing to share logins.
And JPMorgan Chase applies machine learning models to deliver personalized research and recommend services to customers without human intervention.
The “holy grail” for fintech and finance firms is to enable non-technical users to explore data without a lot of training or onboarding. Many fintechs are starting to achieve this accessibility to the data through a common platform and a “semantic layer” that provides business users with an easy way to understand the data. This multiplies the number of data citizens in your organization. It also frees data engineers to focus on developing use cases for machine learning, which can help uncover trends or patterns in data sets that are too large to analyze using traditional systems.
Accomplishing this requires careful thinking about the technology platform your company uses and how your data and analytics teams are organized. Let’s explore the roadblocks facing fintech companies, then consider how to develop the data strategy and self-service platform so it empowers business users to make real-time data-driven decisions.
The Roadblocks to Data Access
There are three common roadblocks facing fintechs:
The Self-Service Roadblock: Each time business users want to dig into a particular question, they must ask someone on the data team to build a data pipeline for that specific question.
The Data Freshness Roadblock: In traditional systems, data is extracted and aggregated prior to the query. Without these pre-calculations, visualizations would be too slow to be useful. However, this means data freshness suffers. Business users are unable to access same-day data and use it to inform decisions and the critical details of the data may be lost in the aggregation.
The Consistency Roadblock: The combined punch of the self-service roadblock and the data freshness roadblock means users are working with slightly different data each time they run their analysis, so the results are often inconsistent.
Let’s consider how to address these challenges using existing technologies.
Creating a Data Strategy and Self-service Platform
To overcome these obstacles, it's important to create a data strategy and reconcile business goals with technical infrastructure. The strategy should be created with both the business and the data analytics organizations with clear data initiatives to help them achieve their business outcomes.
From a technical infrastructure standpoint, there are three major areas in data and analytics to address:
The Data Storage Layer: Raw financial data typically sits in internal databases or external sources like third-party data vendors or SaaS applications. The first step is to feed this raw data into a data platform where it can be processed into something that’s usable. This storage layer is typically managed in the cloud due to the efficiencies, scalability, and “pay for what you need” characteristics of cloud data warehouses.
The Modeling Layer: Data sitting in a warehouse or data lake is complicated. There may be hundreds or even thousands of tables to sift through. Exposing this to end users would require them to have an expert-level understanding of how the data fits together. Instead, we store that knowledge about the table operations in an intermediate layer. Data engineers build models of the data, and those models are exposed to end users in the business language they already understand.
Consumption Layer: The users interact with the data using a consumption layer. This is where end users answer questions and build content to present their decisions.
Many fintechs choose the best-in-class tools for each layer. Ultimately this enables a lot more people to create and share their own dashboards, reports, or visualizations.
Real-World Example: Trumid Provides Credit Market with Business Intelligence
Trumid offers an electronic trading platform that leverages data science to empower all credit market participants with business intelligence to make more informed decisions. The company has about 130 employees and trades about $2 billion a day in corporate bonds. Their accounts include large and small hedge funds, big asset managers and sell-side institutions at major Wall Street banks like JP Morgan and Goldman Sachs.
The bond market is not as automated compared to other parts of the securities market. For example, if you place a trade for a stock electronically, there are no humans involved. However, if you want to trade an institutional bond, there’s likely not an electronic trading option and instead requires a phone call or instant message. Trumid is trying to change this process by moving as much of the bond market onto electronic platforms as possible. Its mission is to build the best customer experience for that type of trading.
To embark on this mission, Trumid first developed a data strategy that involved identifying its business objectives and then mapping them to the necessary technology and data science. To allow for self-service analytics, while keeping data consistent and up-to-date, it chose best-in-class tools for each component:
The Data Storage Layer: There are many options for cloud data platforms. Trumid chose Google BigQuery due to its infinite scalability, along with its ecosystems and managed integrations with Google Cloud Platform and GitHub.
The Modeling Layer: Trumid chose AtScale. AtScale allows data stewards to expose business-friendly data to end users while concealing the complexities of data formats and locations.
Consumption Layer: Options here include Power BI, Excel, and Looker. Trumid chose Looker due to its ease of use, ability to create fast visualizations and the way its permission model allows customers to expose data while dynamically enforcing rules about who is able to see that data.
Trumid now has a high-performing semantic layer that is driving self-service of data consumption and has improved its enterprise data management significantly. Ultimately, Trumid has given its employees and its customers the ability to make data-driven decisions based on the most current data. This empowers all credit market participants with business intelligence to make more informed decisions.
A Final Thought
The fintech market is huge, with hundreds of billions of dollars at play. Innovation is a key differentiator and essential for improving the way you run your business.
With the right data strategy and self-service platform in place, everyone wins. Non-technical users are able to ask questions, explore data to find answers, then create content to present those decisions – without any help from data analysts or engineers. And data scientists can focus their time and energy on innovation to drive the future of the business.