Banking & Finance: Winning the Data Race in Strategic Decision-making
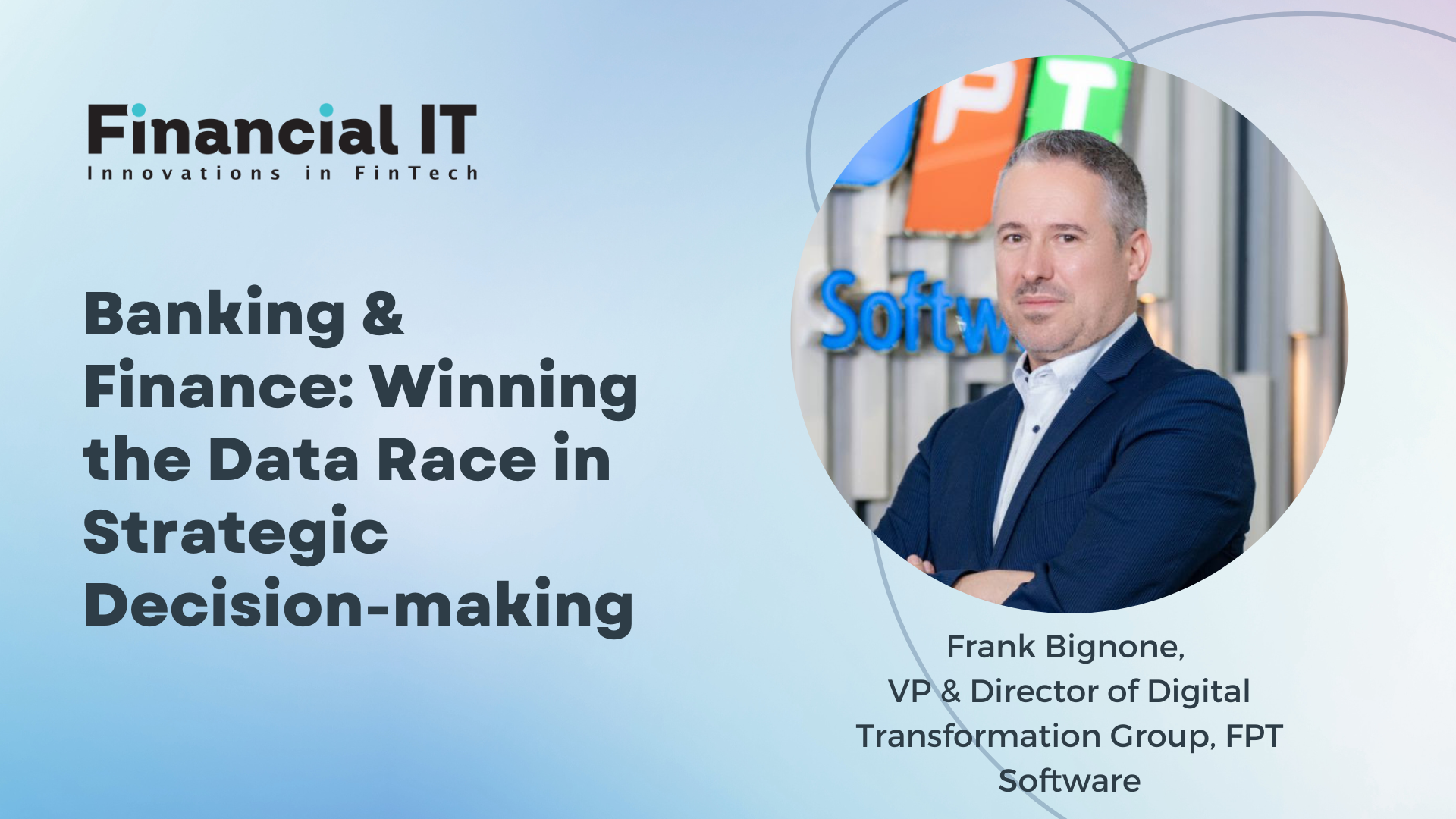
- Frank Bignone, VP & Director of Digital Transformation Group at FPT Software
- 21.06.2024 01:15 pm #data #banking #finserv
Data analytics in banking advances at breakneck speed, with banks and financial services companies racing to be early adopters to stay ahead of the competition. Traditional artificial intelligence (AI) has come a long way in improving efficiency and decision-making in the banking sector, but it has limitations when dealing with unstructured data, natural language understanding, and complex contextual analysis.
The industry is witnessing a revolution from rule-based AI systems to Generative Artificial Intelligence (GenAI) using Large language models (LLMs), driven by advancements in computing power, data availability, and machine learning techniques. No longer constrained by a fixed set of rules, LLMs can adapt to new contexts and language uses, making them more robust, human-like, and versatile for a range of applications. Recognising its state-of-the-art capabilities and potential, most banks have pilot GenAI-infused projects underway and are considering more whilst working out priorities for wider implementation.
How we got to here – quickly
JPMorgan Chase & Co fired the starting pistol for the modern data analytics race in banking with the launch in 2016 of their Emerging Opportunities Engine, which uses predictive algorithms to identify which clients are primed for issuing or selling equity based on AI analysis of granular datasets. Others quickly adopted what became known as augmented data analytics, using artificial intelligence and machine learning (ML) technologies to enhance traditional data analytics. This heralded transformative advantages as new uses were adopted through the expeditious and precise analysis of copious datasets and has been highly successful at reducing costs and enhancing revenue while paving the way for more targeted promotional campaigns and improved customer satisfaction.
Composable analytics then began overhauling augmented data analytics, taking advantage of more advanced machine learning and new technologies such as intelligent data management and microservices to transform how companies use data. To simplify it, companies can update and add new technologies that leverage all the available tools without disrupting other applications. Data and analytics systems can be more modular, adaptable, and flexible to scale dynamically and be more streamlined to meet growing and changing business needs and enable evolution as the business and operating environment inevitably change. By simply adding in or removing a solution at will, banking institutions can pilot new technology, evaluating whether it is suitable for their businesses or systems. Composable analytics can benefit banking institutions due to the large and diverse nature of the data that banks typically handle.
Given that AI-facilitated automation and prediction are now the norm in banking, both still have a major role to play and will have for the foreseeable future.
New Opportunities – and Challenges
The sheer scale of data now available to, and used by, banks and financial institutions is daunting in terms of producing immediate and appropriate output. With the emergence of GenAI marking a significant shift in banking analytics, the industry is investing in and adopting tools driven by even newer, Generative AI-powered systems. McKinsey Global Institute estimates that Generative AI in banking is expected to have an annual potential impact of $200 billion to $340 billion (equivalent to 9 to 15 percent of operating profits), largely from increased productivity. GenAI is altering how some jobs are done and how customers interact with banks, and might even lead to entirely new business models, said McKinsey, which is already a prediction coming true.
Compared to conventional AI, GenAI, incorporating large language models, can learn patterns and structure from even larger volumes of data, including information from unstructured inputs. In banking, this represents a shift towards more sophisticated and creative AI models that can generate highly personalized content for communicating with customers. While traditional AI simply analyses data and makes predictions by following pre-programmed rules, Generative AI, built upon deep neural networks, autonomously creates coherent and contextually relevant outputs. GenAI models, such as Generative Adversarial Networks (GANs), Variational Autoencoders (VAEs), and large language models like GPT, can create synthetic data that replicate the statistical properties of real-world datasets. This is particularly important when real data is scarce, expensive, or sensitive. It opens up a new frontier for banks: by analysing historical data and incorporating real-time market trends, GenAI models can generate more accurate and nuanced forecasts, enabling banks to make informed, real-time investment decisions; and create customized financial plans for individual customers, factoring in their financial goals, risk tolerance, and market conditions. A practical example could be generating transactional data for anti-fraud models.
Albeit its immense potential, the adoption of GenAI also presents challenges. Banks face concerns about data privacy, model interpretability, and potential biases embedded in the algorithms, data privacy and security, regulatory uncertainty, blending with legacy systems, and finding the talent to create and operate the new systems. This is where the concept of using GenAI as a "Copilot" emerges. Instead of a complete AI takeover, the Copilot envisions a collaborative approach, with human analysts and AI systems working together. AI handles the heavy lifting of data processing and pattern identification, plus suggesting options, while human expertise focuses on interpreting results, identifying business context, and making final decisions. Several companies are vying to become the premier copilot solution providers, including Microsoft Bing rebranded to Copilot, MachEye, Google Bard rebranded to Gemini, Chat GPT-Turbo, Claud, IBM Watson Financial Services: FICO TONI, Palantir Foundry, and others. These companies offer platforms that integrate various AI functionalities, allowing banks to build and deploy custom applications tailored to their specific needs.
Learning to Succeed
Amidst this fast-evolving technology landscape, GenAI is undoubtedly the new key to lower costs and higher levels of service, empowering businesses to be ahead of the curve. However, it is not ready to replace everything that has gone before. There are, of course, obstacles to be overcome in introducing this new technology, not least the major skills gaps and how to address them: reskill or upskill existing employees, hire new personnel, or bring in contractors to implement new systems while training existing staff to run them.
Advantage is only gained if the implementation is effective, appropriate and everyone involved knows what they are doing. To capitalize on all these real-time insights and opportunities, banks and financial institutions require sufficient expertise from managing systems to interpreting complex data and developing algorithms. Investing in skilled talent, as well as the support of specialized providers and tech partners with profound expertise and global outreach, is crucial.
To this end, FPT Software has been adopting an ecosystem and partnership approach, covering various areas from education and research to solutions development, to propel innovation and responsible, practical applications of AI. Strategically partnering with transformation leaders like Microsoft, AWS, and IBM, we aim to become a trusted partner for global businesses to successfully harness the power of AI and navigate the next wave of transformation.