Data Analytics Strategies For The Future… And Beyond
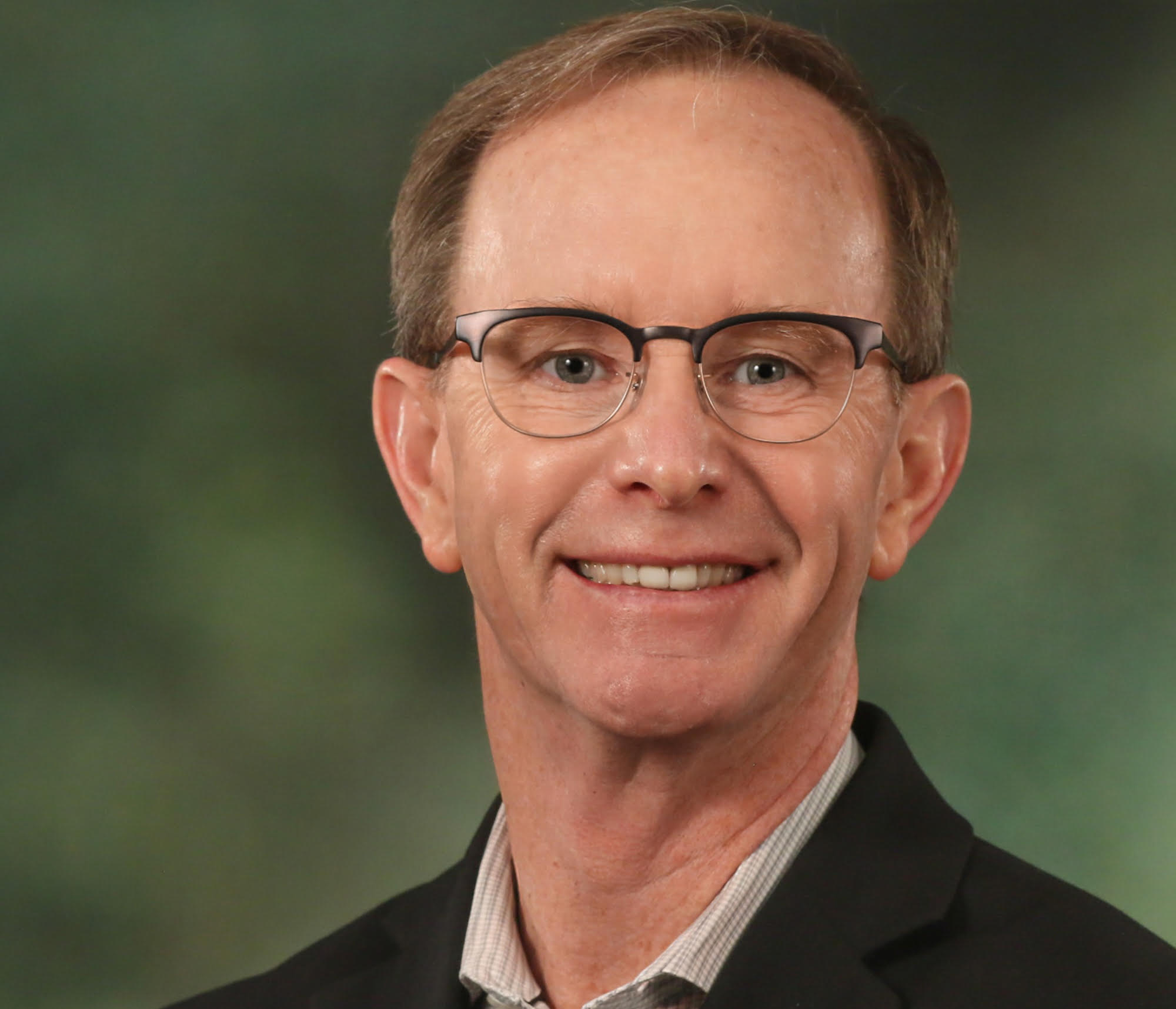
- Bob Eve, Senior Data Management Strategist at TIBCO
- 09.02.2021 06:00 pm Data Analytics
Information is everywhere, we all know it and we all accept the ubiquitous proliferation of digital platforms, tools and devices that exist in almost every aspect of our lives. Yet, despite this mostly willing embrace that we all exhibit, there are instances of data analytics management in organisations across every vertical that are less than optimal and poorly provisioned for future scalability, adaptability and all-round applicability.
With the deafening disruption of 2020 now behind us, 2021 and beyond can be regarded as a significant period of realignment and continued adjustment. With this rebirth (and perhaps even renaissance) in mind, how can businesses make sure that data analytics becomes part of their competitive advantage and not a bottleneck to their own success?
A multi-vector impact surface
When data analytics is used assiduously, judiciously and intelligently, its transformative impact is felt across a number of key vectors.
As a foundation, data analytics has the ability to enhance and improve existing business processes to ensure operational excellence throughout an organisation. Moving outwards, that excellence is then reflected in the ability to deliver more compelling customer experiences in a firm’s core market… and throughout its supply chain. Moving onwards and outwards, that same positive impact allows the business to innovate and engage in business reinvention initiatives.
It’s important to remember that there is no magic wand here. That is to say, no firm can just invest in a new raft of servers, systems and end user devices and then declare itself data-centric overnight. Data is an opportunity, but it is also a challenge. According to a new update to the Global DataSphere from International Data Corporation (IDC), more than 59 zettabytes (ZB) of data will be created, captured, copied, and consumed in the world in 2020* – that’s a lot of data to manage.
The data challenge is made more difficult by virtue of the fact that of our 103 Zettabytes, only 15% of the data created will be new original data. This is because the bulk of the new data mountain we must climb each year duplicating copies of data that already resides somewhere else in a different database, application, cloud service or some other information stream.
Even if we can zone in on the 15% new and original data segment, much of it will be raw, badly formatted, potentially corrupted or generally unstructured by some other measure. There is, in short, a lot to do… so let’s break it down into five core building blocks of action that every organisation can use a strategic guide.
Develop an aligned data analytics strategy
Information appreciates order, form and structure, so an organisation should reflect that adherence to systematic order with a data analytics strategy that supports and even accelerates its business transformation strategy.
But data is a living, dynamic and often unstructured element of business that may be called upon for a changing set of needs, for different use cases in different applications via different platforms over time. We can manage that uncertainty if we invest like a stock market portfolio manager and ensure that we never put all our eggs in one basket.
In this scenario, the organisation builds a data analytics strategy that leverages a diversified portfolio of assets, with focus on driving more value from the ones that drive the highest business impact, for example customer related data that helps drive revenue growth. That way we can act, learn and improve our data-driven business over time, while still observing the boundaries set out by risk management and compliance regulations.
An adaptive data analytics architecture
Nobody, just nobody, extols the virtues of closed narrow approach to data analytics architectures… and with good reason. Building and maintaining an open and adaptive approach to data analytics should be our only goal here. This is because today’s distributed cloud-centric topologies share the same decoupled and layered approach that intelligent data decentralisation exhibits throughout its DNA.
As we move to make use of modern approaches to data lake technologies (including so-called data lake houses), we can create new solutions for both people and machines that run on distributed data architectures designed to span data from any source, anywhere… and deliver it to any consumer, anywhere, on any device.
This is all part of what we often now call data democracy i.e. making both the data itself and self-service data analytics tool functionality available to all users regardless of their technical skills. An adaptive and open data analytics architecture that embraces open standards is ready for business change where and when it happens – and this is one constant that we can generally always bet on.
Agility drives business capability
When we talk about agility in technology circles we often capitalise the term in deference to the Agile Manifesto, which guides programmers to deploy rapidly and embrace change, early and often. Next generation data management and data analytics will use more agile development and deployment methods that integrate the people, process, and tools required to help organisations build and iterate new datasets and AI/ML models faster and thus deliver new business capabilities apace.
In adopting new DataOps and ModelOps techniques that ensure more agility across our total data lifecycle, we can take advantage of increasingly AI-based tools to help us operationalise, optimise and further democratise data analytics.
Augmented people, autonomous advantages
As it starts to manifest itself in almost every layer of the technology stack, we will also see the benefits of AI and ML brought to bear on new approaches to data analytics. Organisations can now start to take advantage of modern AI/ML capabilities inside the data analytics tools that they use and so automate some of the more definable, repeatable and quantifiable work that they need to shoulder on a regular basis.
And on the analytics side, smarter use of AI/ML can help us build analytical algorithms, learn and improve them iteratively, find new patterns and insights without programming and optimise data analytics run time performance. We can also see that AI and ML applied inside data management tools to reduce workloads by automating manual processes such as data discovery and matching, data model design and query optimisation.
Advanced data analytics for the future
As a fifth cornerstone here – and to reflect our original title – let’s think about advanced data analytics for the future. Organisations now have the opportunity to break down the previously disparate disconnected silos that form their total approach to data mechanics.
For example, at most organisations, metadata management, master data management, data governance, data catalog, data modeling, data quality, data security and data integration are typically separate solutions. The same can be said about reporting, visualisation, analytics and data science applications. These silos result in greater complexity, higher costs, and greater internal friction. In a nutshell, more effort, less value-add.
A more progressive future-proof approach sees organisations embrace technology convergence as they update siloed best-in-class tooling with data analytics that is capable of self-service visualisation, advanced data science, streaming analytics, and reporting.
Data continues to be everywhere, in every application, on every device and now inside every intelligent machine. As we start to build an increasingly powerful data analytics approach for the future, we will naturally work with more types of data from the upper end user layer down to lower log files and system events. Now is the time for future data analytics and, equally, the future is now… and you can work that one out yourself.
* IDC Press Release, IDC's Global DataSphere Forecast Shows Continued Steady Growth in the Creation and Consumption of Data, May 2020