The business of transforming risk
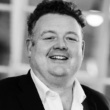
- Richard Price, Head of FSI, UK & Ireland at TIBCO Software
- 29.07.2019 11:30 am undisclosed , Richard is an experienced leader with extensive IT Sales, Marketing and Partner enablement experience within EMEA. Having joined TIBCO in late 2016, Richard assists TIBCO clients and their partners deliver business advantage with the challenges they face across the financial services and insurance sectors. Prior to joining TIBCO, Richard held a number of senior sales positions at Open Text over a period of eighteen years, and has a proven track record running sales and partner collaboration across a number of financial services sectors including Retail, Corporate and Investment Banking, as well as General, Life and Specialist Insurance. Front Office projects delivered include direct customer portals in wealth management, global marcomms and marketing asset management platform in investment banking, brand management & product delivery in retail banking and underwriting & claims re-platforming in insurance. Back office projects include delivering transformational global HR management processes projects, ERP management delivery from invoice management to expense processing, and AUM & market positional compliance programmes with High Performance Compute, Analytics and Content Management projects.
The business of transforming risk
Chief Risk Officers in the financial services sector currently have their work cut out to stay on top of complex regulatory change while overseeing the digital transformation of their bank’s risk function. At their fingertips, they have a wealth of knowledge and data resources that they must make best use of in achieving this task, applying data science and smart, AI-driven analytics as well as appropriate governance to help them.
Striking a balance
The shifting nature of risk and risk management, and the drive to modernise and automate it, is being played out against the backdrop of major industry challenges that all banks are facing. These range from squeezed margins to the need to sustain and, where possible, improve performance in the face of a changing competitive landscape to the more down to earth imperative of increasing the wallet share that each client spends with them.
The banks have a wealth of data on their side to help address these issues, provided they can find a way to exploit that data successfully, something that most have experienced trouble in doing. Their central difficulty can be defined as the need to strike a balance between the horizontal requirements of the data problem from a market and a regulatory perspective, as set against the vertical requirements seen from a credit perspective. And this in the context of an industry that is rapidly digitising every function.
Digitising the risk function with the right platform
The banks that are managing the process of transformation best are those that are making full use of the right kind of AI-driven analytics platform. Some institutions are deploying machine learning, applying AI technology and scaling algorithms across trading businesses on a much broader scale than rivals, and indeed than they themselves were doing even two years ago.
A crucial part of the task is digitising the risk function. In order to be able to digitise risk, you’ve first got to use advanced technology to put a very clear data governance structure in place that allows you to attack the horizontal market and regulatory requirements, while also facilitating all of the vertical requirements.
This approach, and only this approach, will allow banks to flip the current paradigm that most of the financial services sector is facing, whereby organisations currently spend 80% of their time gathering data and only 20% actually analysing it. What they actually want to be able to deliver is spending only 20% of the time gathering data, because their analytics platform and their governance structure is good enough to allow this to happen. Then they can begin to address issues such as the current shift in approach by regulators who are now saying ‘Don’t tell us you’re compliant, show us you are – now.’
An end-to-end data and analytics platform is needed which provides fully functional operational uses for data across the enterprise and down into business lines. Such a platform continually learns, either through users utilising the data or applying advanced technology to the data itself.
Data science allows the bank to take that data, operationalise it and apply market-based algorithms and machine learning tools to it.
The issues behind the model
The intellectual and operational challenge that most banks face in relation to undertaking projects like this is they don't want to, as it were, boil the ocean.
Better that they should start by tackling one very specific regulatory requirement, like delivering reports to the regulator. By deploying the right tool set to that specific regulatory report requirement, they can define the starting point of a data model, and then identify other use cases right across the enterprise.
They can end up, in our experience, moving through the organisation applying the model to different structures within the organisation, while also applying it to different business lines. They can, in so doing, address credit risk exposure for the regulator, and then push that credit risk exposure into, say, equities, fixed income and commodities. This ultimately improves profitability.
The alternative is to start to work at a much broader level across the organisation, applying AI-led analytics to the employee organisation, the supplier organisation and consumer credit organisation.
The other issue lying in wait for the risk professional is around regulatory risk management. There are many different regulatory bodies around the world, each with its own rules and requirements, from the Securities and Exchange Commission and Federal Reserve in the US to the Bank of England and FCA in the UK, not forgetting numerous EU bodies. All the different regimes are after different bits of data in different ways, reported in different formats. They're all measuring the systemic risk of the institution based on slightly different criteria. The challenge for the bank is to be able to invest in an infrastructure once that allows them to be able to cater for all of those different and evolving regulatory requirements which have changed dramatically over the last 10 years.
This is in essence a data problem, because traditionally the banks have built up silos of technology that don't really operate together. The regulator isn't really interested in a bank's individual exposure to a given risk class, they're interested in the overall aggregate exposure of the institution. And each regulator is interested in it from different perspectives, so the challenge for the institution is being able to collate all of that data in a very timely and efficient fashion in the way that the regulator demands. They must do this across multiple jurisdictions and at the lowest cost possible.
You can break down banking, in a simple way, into three core objectives: to make money, manage risk and cut costs. The right AI-driven analytics platform delivers on all three.
But half measures won’t do it. The banks that manage the data challenge and the process of digitising risk the best are those that really invest to get it right, not those who are just ticking a regulatory box.