Anomaly Detection for Modern Banking will Keep Our Safes Safe
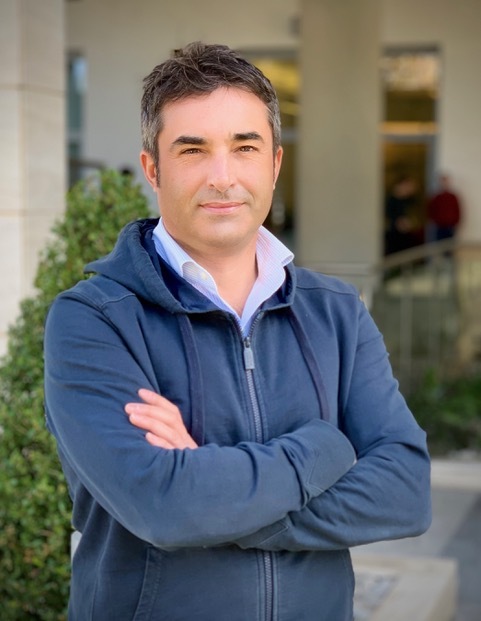
- Alessandro Chimera, Director of Digitalisation Strategy at TIBCO Software
- 07.06.2022 01:00 pm #banking #security
Banking has been through various evolutionary cycles over the centuries. Leaving ancient history aside, we have moved from the safe-deposit boxes of the Wild West, through the Victorian era with its great halls and marble facades, towards the present day.
In the current era, we have quickly progressed from desk clerks to the first Automated Teller Machines (ATMs) and, in some countries more than others, ‘drive-thru’ services that come with optional parking. But even these modern conventions are becoming surpassed, outmoded and outdated.
Ubiquitous, frictionless, seamless
Driven by their popularisation among the Generation-Z youth, electronic payment systems and banking app usage has spiralled. We now expect to be able to conduct transactions, access money and interact with banking services anywhere and at any time in a frictionless and secure way on a device of our choice.
With the new generation of so-called ‘challenger banks’ not even investing in physical premises and with the spectre of cryptocurrency on the horizon, we need a new approach to securing digital banking systems and the increasingly digitised economy against the risk of fraudulent transactions.
The risks emanating from today’s use of still-evolving digital transaction channels are multifarious and many. As we adopt more use of mobile wallets and banking apps, we naturally further increase the speed at which transactions actually occur. This makes it inherently harder to be able to scrutinise, examine and analyse every transaction in order to assess its potential for fraud.
All of this is happening against a backdrop where banks themselves are sitting on years or decades of badly managed, poorly maintained, haphazardly organised and often unstable data. As disconnected silos of incongruent data are brought together, old-fashioned organisational barriers can further hamper any notion of a connected, agile business.
Eradicating false positives
A major challenge for organisations operating modern banking systems is the fight against false positives. When transactions are flagged as potentially fraudulent when they are not, systems slow down, services are delayed and customers get frustrated and, ultimately, move banks.
False positives are everywhere. Global news analysis website Global Investigations Review (GIR) estimates that as many as 98 percent of digital banking systems alerts never result in a formalised Suspicious Transaction Report (STR). This kind of activity can lead to banks suffering from fines and reputational damage.
In a world of near-instantaneous payment processing, old-school rule-based fraud detection systems are only as smart as the code they are made up of which, in this case, isn’t smart enough.
There has to be a better way – and there is; we can use anomaly detection.
Anomaly detection
In terms of a formal definition, we can say that an anomaly itself is an unforeseen variation or deviation from an expected pattern in a particular dataset. The deviation tells us that one or more input conditions have changed; and this move outside of what is defined as ‘normal’ can be used to trigger an appropriate response and so act against fraud, security breaches or perhaps even operational performance issues.
Financial institutions old and new all process massive amounts of data across a wide variety of different datasets, databases and data repositories; by using anomaly detection, these organisations can spot transactions that break from expected patterns or deviate from previously observed behaviours.
Anomaly detection takes three basic forms: visual detection supervised learning and unsupervised learning.
Visual detection requires a data analyst, data scientist or industry specialist to study dashboards made up of charts, graphs, meters, gauges and other data visualisations to look for data variations. Limited by the realms of any given specialist’s industry knowledge, visual detection is useful, but relies on flawed human ability and capacity.
Supervised learning also involves humans who will work to label a defined set of datasets as normal or abnormal. In this case, a data scientist uses labelled ‘normal’ data to create machine learning models that can detect anomalies on unlabelled data. This technique is also useful, but flawed by virtue of the ever-evolving nature of fraudulent threats.
The most machine-driven of the three types of anomaly detection – unsupervised learning – analyses unstructured real-time data using autoencoders and machine learning algorithms to identify anomalies without human intervention. In modern banking systems where payment approvals need to be instantaneous, unsupervised learning is especially useful in detecting unknown patterns from massive datasets.
Reinforcing anomaly detection with AI, ML, event processing and advanced analytics, financial institutions can detect emerging fraud patterns appearing in real-time data streams. They can then analyse those patterns in the context of overall transactional history and instantly flag real-time potential fraud indicators for manual review.
One company harnessing these technologies is Asurion, a leading provider of device insurance, and warranty and support services, for cell phones, consumer electronics, and home appliances. Using an enterprise-class analytics platform, Asurion estimated a reduction in its fraud dispute ratio by up to 50 per cent. Additionally, advanced analytics helps prevent fraud and risk in its systems and provides a better customer experience.
A blended holistic approach
With so many factors to consider here – and with fraudulent attack vectors multiplying and morphing every day – it is perhaps no surprise to find that the most prudent tactics for operational safety in this space come down to a blended holistic approach. What this means is a combination of supervised and unsupervised models brought together through AI/ ML, event processing and analytics to provide the most accurate indication of potential fraud.
Adopting an anomaly detection platform to combat fraud in the digital banking and financial services arena means selecting a technology that is adaptable, modular and flexible. This way, organisations can adapt to new fraud scenarios as they arise.
By integrating data discovery and statistical modelling into one solution, next-generation financial organisations can create visual tools that collect real-time data from multiple sources and transform it in numerous ways to dig as deeply into an alert as possible.
From the gold bullion of the Wild West to the bitcoin of tomorrow, we can now use anomaly detection to keep our safes safe and our savings safer. Just remember, that while the sheriff is out of town, we still need someone to be on the lookout.