Artifacts of trading behavior
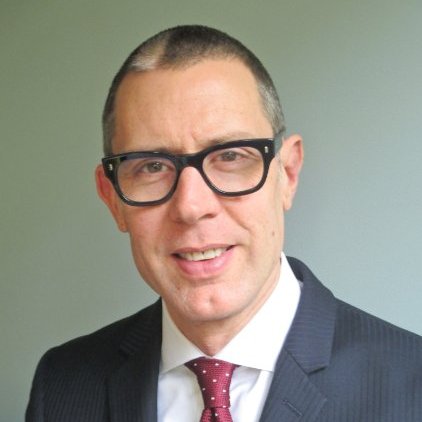
- Mark Brennan, Head of Business Development at Americas
- 07:00 am undisclosed
This week marked the five year anniversary of the Flash Crash, but for several weeks now the financial press have been running articles on a daily basis on the spoofing indictment brought against a UK trader, whose actions the CFTC alleges “contributed to an extreme E-mini S&P order book imbalance that contributed to market conditions that led to the Flash Crash.” The original analysis of the event, published jointly by the SEC and the CFTC, attributed the cause to market structure complexity and transmission mechanisms: an extremely large S&P e-mini sell order in the futures market by a fundamental trader was thought to trigger a profound liquidity imbalance in related cash markets.
Thus the spoofing case based on artifacts of trading behavior alleged to have taken place nearly five years ago has caused surprise and consternation, as the regulators’ focus shifts from market complexity and instability to manipulative trading. And yet there is a view that complexity in market structure and complexity in trading behavior are similar problems: prices, orders, transactions, and their latent and manifest strategies and intentions are, one and all, delineated and defined by myriad streams of real time data.
Good or bad, professional traders have always tried to derive alpha in their execution strategies; and markets have always been defined by their dynamic range of price signals. Regardless of asset class, and at a macro level, markets are driven by supply and demand; and yet we know there are numerous other economic influences that impact the micro structure of markets. Many argue that technology has made markets more efficient and cost effective (the tight spreads, reduced commissions and ample liquidity of US cash equity markets are canonical examples); while at the same time we know that technology amplifies issues – the rapid and precipitous drop in stock prices during the Flash Crash, due in part to “stub quotes” and complex technology system behavior, is an oft-cited example, especially as the Flash Crash is revisited during this news cycle. So too with the fragmented nature of equity markets and the relationships and linkages among asset classes: technology offers unique ways to navigate such complexity (e.g., smart order routing for fragmentation, and quant-driven statistical arbitrage for multi-asset alpha trading strategies); but technology also exacerbates and accelerates risk – it’s possible that the tight, technology-facilitated relationships among the S&P e-mini futures contract, the SPDR ETF, and constituent stocks contributed to the wild price swings observed during the Flash Crash.
And if a market’s order book is a collection of signals, how can we judge those traders who derive profit from the timing and interaction of such signals? Well, spoofing as a manipulative practice is formally forbidden through statute, CFTC “guidance”, and new rules added to exchange rule books. If monitoring for system stability has long been a focus of market participants, especially banks running large trading infrastructures (and Reg SCI takes this up a notch, codifying best practices and introducing an administrative onus), the regulators are now implying that monitoring behavior is equally, if not more, important. The regulatory burden now shifts from monitoring system data and metrics to business intelligence. Sophisticated trading systems typically offer a variety of mechanisms for viewing and trading the best bid and offer, as well as multiple ways of viewing level 2 data, including, in some cases, denoting which bids or offers in the book are comprised of a trader’s own orders. But the behavior of transactions, whether initiated by a human via a screen or an algo, is typically not scrutinized. In order to ensure their trading patterns cannot be construed as manipulative, operators need to understand their fill ratios, and the proportion of executions to modified or canceled orders, among other metrics. Real time streams of data must be analyzed alongside historical transactions, prices and events; and these disparate sources must be collated and collected.
Thus the need for transparency transcends system stability and health and moves to a more sophisticated understanding of behavior.