Historical Data is Holding Algos Back, According To Traders
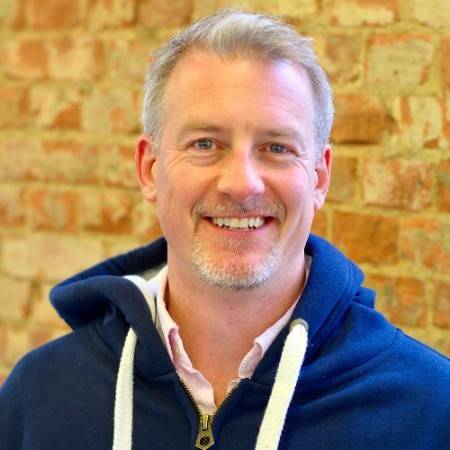
- Justin Lyon, CEO at Simudyne
- 03.07.2019 09:30 am algorithms
Today, trading strategies and algorithmic models have become a crucial differentiator to buy-side customers. With the unbundling of research and execution coupled with low-latency connectivity becoming the standard, ensuring algos are working as efficiently as possible is a primary concern for traders.
But what’s stopping them from producing near 100% efficient algos? We asked a number of senior executives at banks to identify the key issues they face in achieving – and beating - the benchmarks.
The priority? Getting more order flow
Competition among sell-side firms is fierce. Indeed, banks now see attracting client order flow as the main purpose of their investments in algo trading. More than 60% of traders we spoke to cited it as a key driver of their algo development activities. One trader noted: “What did we spend the most time on over the past six months? It’s probably regulatory compliance. But what is my priority? It is getting more additional order flow.”
To achieve additional order flow, a third of those we asked said the biggest challenge for them was demonstrating the applicability of an algo to meeting client objectives. “It’s about proving that our algos are better than others,” said another trader: “the hardest thing is getting the opportunity to demonstrate what it is that we can do.”
But firms told us that they often find themselves limited by their ability to backtest their algos in an attempt to ensure they are able to deliver what’s desired of them. Traders struggle with overfitting, multiple testing bias and the problems associated with using limited data in backtesting, or they may be unable to calibrate their algos quickly enough to respond to client requests.
Unknown unknowns
Having the right data is a huge issue when trying to backtest. Forty percent of those we spoke to said modelling against unusual market conditions and macroeconomic shocks was “challenging or very challenging”. Half pointed to the fact that finding high quality data to test their algos against any number of possible scenarios was a difficultly. “You don’t know what you don’t know, so it’s hard sometimes to factor in or incorporate market shocks,” said a Senior Vice President of Electronic Trading.
Another executive noted that their firm had its own development environment where traders are able to run historic simulations so that they can put algos through extreme situations modelling. Traders complained to us that, due to this reliance on historical data, it’s difficult to be able to confidently adjust more parameters of their algos – the traders we spoke to said a lack of foresight means a lack of flexibility. In fact, continuously tuning and optimizing algos is considered “challenging” by more than a third of respondents to our conversations.
This is unfortunate because traders indicated that better simulation capability would translate into more rigorous and expansive scenario testing. And, if they were able to better understand how small calibrations and changes would act in a future market, traders said it would give them a significant advantage when it comes to improving execution quality. Respondants estimated that the financial benefit of such an approach could translate into substantial financial advantages – 0.05% to 1% improvement in the bottom line for a 1% improvement in fill rates.
Creating a future market
To be able to make it onto the algo wheel and create more flexible alogrithms that could change ‘on the fly’ without fear of failure, it is clear that simulating future markets in a virtual environment is necessary.
Using simulation, firms can circumvent the issues presented by traditional approaches to backtesting, allowing them to train their algos for an unlimited range of trading regimes. While backtesting on real data commonly results in bias and overfitting, using synthetic data can reduce this bias, allowing the firm to focus on feedback dynamics, which aren’t supportable using simple historical time-series or standard stochastic processes. Traditional approaches are crude in comparison with agent-based models. They are like using a map drawn on a cocktail napkin when you really need a high resolution, live GPS to take you on an intercontinental journey.
Having recreated the underlying system, these kinds of tools have the advantage of parameter adjustment, allowing users to construct any potential scenario they choose. So, by introducing multiple potential market dynamics, sell-side brokers can ensure their algos function under many types of stressed scenarios and can perform efficiently in the broadest range of eventualities.