Future Says... Ethical AI
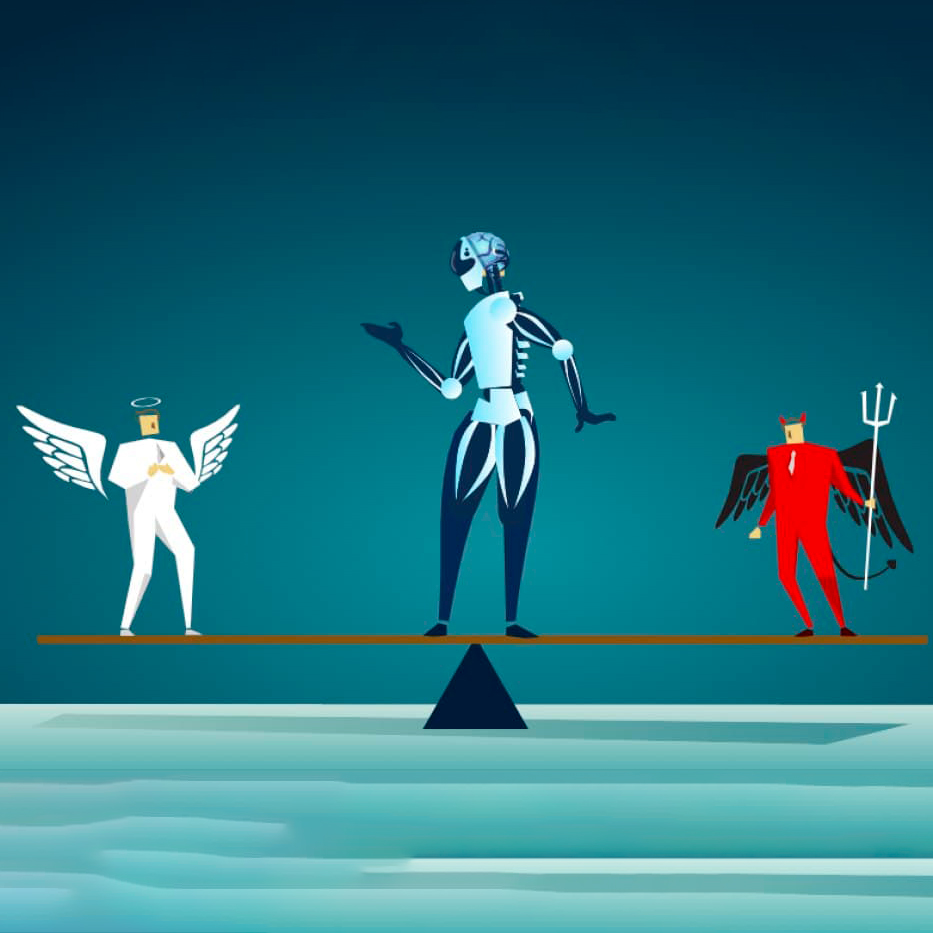
- Sean Lang, at Democratising AI
- 23.07.2021 10:00 am #AI
“AI is an instrument just like anything else. You can do harm and you can do wonderful things. ESG is the embodiment of all the good things you can do with AI. Squeeze all the juice out of AI but at the same time we need to understand the consequences so we can do things responsibly!”
The wise words from Aiko Yamashita, Senior Data Scientist at the Advanced Analytics Centre of Excellence in DNB Bank, during our conversation on Altair’s ‘Future Says’. Alongside her role at the Nordic’s largest financial institution, Aiko is also an Adjunct Associate Professor at Oslo Metropolitan University and holds a PhD in experimental software engineering. With her experience in both academia and enterprise, Aiko represents the perfect ingredients to ensure the next phase of AI is a successful and responsible one.
Over the past few years, interest in artificial intelligence has rocketed with no sign of abating. Adding to an already long list of optimistic statistics, Citrix has now crowned AI in their Work 2035 study, as the single biggest driver of organisational growth. AI transforming enterprises is not a new concept, but 2020 was the year that AI finally rose to prominence within society at large. It was an AI algorithm, developed by BlueDot, that first alerted the world to Covid-19 - a full 9 days before the World Health Organisation. Moreover, it was thanks to AI that scientists could predict the shape of proteins within minutes (rather than months) and have workable vaccinations a mere three months after the initial outbreak. Applications such as track and trace, vaccine and ventilator prioritisation, cluster analysis and curbing the spread of misinformation online were also made possible due to the advancements in AI.
More than ever, AI is indeed all around us, and it has fundamentally changed our lives in many positive ways. However, it also has the potential to be a major threat to the world and there needs to be a parallel track to AI deployment which focuses exclusively on the responsible use of data and artificial intelligence. Without this, AI has the potential to join Covid-19 and climate change as the biggest challenges facing our world in 2021 and beyond.
Explainable AI
There needs to be as much focus on model explainability as there is on model development. Alongside the fantastic applications and achievements listed above, 2020 will also be remembered by many as the year students protested against algorithms deciding their exam results, a court case was brought against Uber for robo-firing drivers, and facial recognition technology from behemoths like Amazon, Microsoft, and IBM was taken off the market because of discrimination fears - Amazon hoping it would give lawmakers time to “put in place stronger regulations to govern the ethical use of facial recognition technology.” It was also the year that deepfakes hit the mainstream – as we scroll through our social media newsfeeds, we now need to seriously consider what is and what is not real.
Time after time, we are seeing AI systems influencing decision-making in law enforcement, employment, and healthcare despite demonstrable bias toward marginalised groups. Goldman Sachs has been under investigation for possible gender discrimination in the algorithm powering the Apple Card with husbands having better credit limits than their wives despite their wives having better credit scores. Similarly, Optum (one of the largest healthcare firms in the world) is being investigated for creating an algorithm that recommended doctors and nurses pay more attention to white patients than to sicker black patients (as their predictive models considered previous healthcare costs as a predictive variable). In the same vein, Aiko touched on the importance of explainability within sensitive immigration policies:
“If you ask an immigrant, would you like to trust an algorithm if you would become a Norwegian citizen or not? Sometimes the things at stake are so high that no human will accept that. It boils down to risk and the context in which these things are done. Context plays an important role in terms of explainability. For some specific contexts, no matter how much you explain it, people will always want humans in the loop.”
If companies and governments fail to assure the public that they are trustworthy in their use of AI, there will be increasing public pressure for stricter regulation. Look at the stringent testing Covid-19 vaccines had to go through. Should there be an equivalent body to pre-approve algorithms used within sensitive areas – particularly in life and death scenarios in healthcare and justice? There is genuine concern that, as AI becomes more accessible, it also becomes easier to weaponize. This is not just a metaphorical scenario as the US President and Congress have just released a report focusing on US defence spending on AI. The report talks at length about the potential of AI-powered autonomous weapons and rival algorithms battling it out in the future.
As AI grows more sophisticated and ubiquitous, the voices warning against its potential pitfalls are stacking up. Elon Musk has said “Mark my words, AI is far more dangerous than nukes” while Stephen Hawking has stated, “unless we learn how to prepare for and avoid potential risk, AI could be the worst event in the history of civilisation”.
The opportunity is that AI will be a force for good, empowering people and organisations to achieve more. The risk is that AI is allowed to operate beyond the boundaries of reasonable control. The point to remember is that we have the power and responsibility to shape the future AI landscape and come up with answers to the ethical questions. That goes for transparency, equality, privacy, democracy, and fairness.
Energy Usage
Another often overlooked by-product of the increase of Artificial Intelligence is the environmental after-effects. AI needs a lot of data, and data processing and data storage uses a lot of energy. Unbeknownst to many, the algorithms that tell us what to watch on Netflix tonight will have an environmental impact. Many of the high-profile advances in AI in recent years have had staggering carbon footprints. According to some studies, training a single NLP model can lead to emissions of nearly 300,000 kg of carbon dioxide, equivalent to five cars over their lifetime. Certainly, not all models are as large as these and often models are not trained from scratch but there will always be a significant environmental impact in terms of electricity use. As Aiko Yamashita explained:
“Companies need to become wiser in weighing up the advantages of using heavy deep learning solutions rather than simpler solutions that could provide a high level of explainability. We don’t want to use a firewater hose to water the garden. We need to understand in which context it makes sense.”
AI and data science practitioners should recognise energy use as a relevant ethical value and indeed an actual success metric. It should be a requirement for developers to track the energy and carbon footprint of their models. A public company’s financial report has always been obligatory to publish. Now, it's compulsory to publish your non-financial report and detail your carbon footprint and human rights initiatives. What is missing is more granular detail on AI projects – companies must measure the energy consumption of their models and begin designing greener algorithms. According to Aiko:
“The requirements for due diligence and reporting are going to be even higher. It is not going to be all environmental - it is going to be a close interconnection between the environment, society, and governance at large. If Covid has taught us something, it is how interconnected they all are.”
AI is rightly being championed as a critical component in the fight against the climate emergency. There is a long list of applications for AI within this space - improving predictions of how much energy we need via smart grids, transforming transportation with electric vehicles and more efficient routing, monitoring ocean ecosystems and enabling precision agriculture to name just a few. According to a recent report by Capgemini, AI has the potential to help organisations fulfil up to 45% of their emission targets detailed in the Paris Agreement. However, it can also be a big contributor to carbon emissions, so companies need to provide further transparency into their algorithm decision-making process.
The movement
Thus far, grassroots efforts have led the movement to mitigate algorithmic harms and hold tech giants accountable. One great example of this is Tristan Harris and his Centre for Humane Technology – check out ‘The Social Dilemma’ on Netflix for further insight into their tremendous work. Similarly, conferences like NeurIPS, now require researchers to account for the societal impact of their work.
In general, companies however have tended to grapple with data and AI ethics through ad-hoc discussions on a per-product basis. The image of ‘Whack-A-Mole’ springs to mind. There are of course exceptions to this rule and DNB Bank now believe that “trust is a currency that we have managed to keep and of course newcomers need to gain that trust [startups like Klarna, Trustly, and Holvi], which makes it imperative for us to go beyond compliance and really be a leading role model when it comes to the responsible use of data”. Additionally, DNB Bank has also collaborated with other industry players on seminars around the responsible use of data and AI in the past.
Certainly, most of the leading tech firms have established ‘ethical principles for AI’ and companies like SONY have started screening their AI-infused products for ethical risks. Ethical frameworks and toolkits are definite steps in the right direction, but the removal of revolutionary AI ethicists Timnit Gebru and Margaret Mitchell from Google in recent months has flashed another spotlight on the limits of voluntary corporate standards. If leading corporations like Google cannot make space for ethical critique, is there any genuine hope for truly advancing ethical AI?
Fortunately, companies are now realising that failure to operationalise an ethical AI strategy is a distinct threat to the bottom line which could expose them to serious reputational and legal risks as well as inefficiencies in product development. For example, Amazon spent years working on AI hiring software, before scrapping the program because of systematic discrimination against women. Similarly, Sidewalk Labs, a subsidiary of Google, cancelled their plans for a Toronto smart city project over concerns around the ethical standards for the project’s data handling – following two years of work and $50m investment.
Clearly, companies need a plan for how to use data without falling into ethical pitfalls along the way. They must systematically and exhaustively identify ethical risks throughout the organisation - from IT to HR to marketing to product and beyond. To help, I would urge all companies to review The Harvard Business Review’s 7 steps to building a sustainable data & AI programme:
Identify existing infrastructure that a data and AI ethics program can leverage: a data governance board that convenes to discuss privacy, cyber, compliance, and other data-related risks.
Create a data and AI ethical risk framework that is tailored to your industry: an articulation of the ethical standards of the company, an identification of the relevant external and internal stakeholders and an articulation of how ethical risk mitigation is built into operations.
Change how you think about ethics by taking cues from the successes in health care: an essential requirement of demonstrating respect for patients is that they are treated only after granting their informed consent — understood to include consent that, at a minimum, does not result from lies, manipulation, or communications in words the patient cannot understand.
Optimise guidance and tools for product managers: While your framework provides high-level guidance, it is essential that guidance at the product level is granular.
Build organisational awareness: It is important to clearly articulate why data and AI ethics matters to the organisation in a way that demonstrates the commitment is not merely part of a public relations campaign.
Formally and informally incentivise employees to play a role in identifying AI ethical risks: When employees do not see a budget behind scaling and maintaining a strong data and AI ethics program, they will turn their attention to what moves them forward in their career. Rewarding people for their efforts in promoting a data ethics program is essential.
Monitor impacts and engage stakeholders: Due to limited resources, time, and a general failure to imagine all the ways things can go wrong, it is important to monitor the impacts of the data and AI products that are on the market.
As AI applications flood our world, we must recognise the urgent need for a new set of rules to protect our privacy and our dignity. Just like when cars were first introduced, humans adapted to the new ‘rules of the road’ but were helped with speed limits, road signs, traffic laws and safety regulations to ensure a smooth transition.
Without a doubt, it will be up to lawmakers to establish these stricter regulations. Fortunately, this transition has already commenced. Members of Congress have introduced bills to address facial recognition, AI bias, and deepfakes. The European Commission have announced a package of reforms while the UK have imposed a ‘duty of care’ on social media platforms.
However, like Covid-19, ethical AI will not be tackled by governments or activists alone. It needs a united response. Aiko shone a light on the consumer themselves:
“The consumer has an important role to play in the direction of how AI products and services are going to move forward, on what kind of things we are OK with. You need to be critical towards technology. This gives signals to big tech and innovation companies – like the Whatsapp scandal which saw a lot of users moving to Signal and Telegram as their main chat channels.”
Shaping a positive scenario for our future will require technology regulation, new standards to mitigate environmental impacts and training the next generation of responsible AI technologists. It must begin with a top-level commitment to ethical leadership and a focus on technical and ethical literacy. With ethical guardrails in place to reduce human errors, everyone can work together to produce solutions that represent AI’s potential. According to Aiko, we all have the potential to be data-driven:
“You don’t have to be a scientist or technologist to understand AI. At DNB, we have data scientists who have a background in statistics or applied mathematics, but we also have people with an informatics background, people with natural sciences like physicists or chemists who have all jumped into the data scientist wagon. We have a zoo of different backgrounds.”
The pandemic has demonstrated how quickly radical measures can be taken for the sake of public health. I would encourage every enterprise to take similarly urgent actions to ensure ethics are at the forefront of all technological developments. Follow Aiko and DNB’s example:
“One of the key values in DNB is to be bold. We want to be bold, responsible and curious. To be bold is to dare to test things but in a controlled setting so we can move forwards responsibly.”
If you want to see this interview in full, as well as all upcoming episodes in Altair’s ‘Future Says’ Series, please follow this link: