How Can AI Help You Today? Uncovering LLMs Value in Financial Services
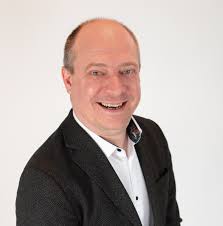
- Chris Royles, EMEA Field CTO at Cloudera
- 07.10.2024 08:45 am #AI #LLM #FinancialServices
In financial services, advances in AI have the potential to enhance a whole host of business processes, from predicting consumer behavior to fraud detection. Yet, in the race to establish a competitive edge, some companies have rushed into AI deployments, resulting in some early teething problems. For instance, last year a major bank allegedly charged customers overdraft fees based on data from an AI-powered system, even when the customers were not actually overdrawn.
So, while some fintechs and traditional banks are beginning to take AI from the lab to production, many are only just starting to dip their toes into the water and are still in the exploratory phase.
Financial services companies need to adopt a thoughtful strategy for AI deployment, carefully evaluating the associated risks and implementing safeguards to mitigate them. Identifying the most beneficial use cases is crucial. But given the proliferation of AI applications—ranging from chatbots to risk modeling—deciding where to start can be a challenge.
The irony is this is where AI can also help.
Enhancing Financial Services with LLMs
By using an LLM to conduct background research, financial services companies can gain a better understanding of exactly where AI can provide the most value in just a few minutes. LLMs can also help to prioritise these use cases by answering questions such as:
1) What are the top 10 AI use cases in financial services? Identifying these can help companies cut through the noise and find where AI delivers the most value. While AI powered chatbots are becoming increasingly common, AI is particularly effective in areas like fraud detection, regulatory compliance, personalised financial planning, and accurate credit scoring.
2) Can these use cases be prioritised based on their impact on revenue? If revenue growth is the primary aim, the next logical step is to determine which of these use cases will impact revenue the most. Here AI can help financial services companies quickly identify which use cases to prioritise first.
3) How do these use cases align with the risk categories outlined in the EU AI Act? Regulators worldwide are increasingly focused on AI safety. The EU is leading the charge with the EU AI Act, which applies to both EU-based companies and those operating within its jurisdiction. Mapping AI use cases against the EU AI Act can provide financial services companies with insights into the potential risks.
4) My company operates in [insert locations]. Which regulations might these use cases impact? Financial services is a heavily regulated industry, subject to legislations beyond AI such as the Digital Operational Resilience Act (DORA) and Basel III. Here, LLMs can help shed light on how different AI use cases could be impacted by wider industry regulations.
5) Can you provide 3 reference examples with links and a summary to show the art of the possible. It is important to use the LLM to provide a reference list of examples which can then be checked and validated.
Asking these questions is a great place to start. But the next step is to delve deeper and conduct further research, as informed decision-making relies heavily on comprehensive knowledge. Large Language Models (LLMs) can point to references for their insights, which helps to direct further research of potential use cases to present to the business.
Armed with this knowledge, financial services companies can have a better understanding of where AI will potentially help them the most. But having the ability to utilise AI is one thing, successful deployment is another – it means more than understanding use cases, risks and regulations.
Data: The cornerstone of AI
To truly harness the power of AI, financial services companies must first ensure their data is primed for success. In today's hybrid, multi-cloud environments, data can often reside in isolated silos, making accessibility difficult. Furthermore, maintaining consistent control and compliance across such extensive, distributed landscapes presents a significant challenge.
This is where a unified data platform, underpinned by a modern data architecture, becomes key. It allows financial services companies to feed AI with trusted data, from cloud or on-premises environments. Strict governance can also be enforced to ensure that data doesn’t leak outside of an organisation and evoke the wrath of regulators.
Driving value from AI
As AI integration becomes more widespread in financial services, prioritising use cases will be key to success. However, financial service providers must first ensure they are genuinely prepared for enterprise AI rather than simply following trends. Establishing a modern data architecture can help to lay a solid foundation for success. Without this initial step, companies risk starting AI projects that are destined to fail before they have a chance to show value.