Demystifying AI in Banking: The Case for a Unified Strategy
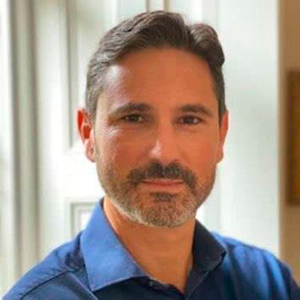
- Ed Lane, VP Sales EMEA at nCino
- 04.06.2021 11:30 am #AI #Banking
Digital transformation can mean different things to each financial institution (FI). For some, it’s a push to modernise legacy systems and acquire fresh talent. For others, a journey to adopt an organisational strategy that unites departments and teams. No matter the motive, nearly all FIs want the same result—to drive efficiency, revenue and cost savings. For many forward-thinking FIs, artificial intelligence (AI) is a key part of this process.
At first, implementing AI can feel like an arbitrary effort that requires too many stakeholders, too much technology, and too big a transformation. Yet, as AI in banking matures, it brings the potential for higher-complexity solutions that generate positive ROI across business segments. A recent financial services study showed that 85 percent of respondents had successfully implemented AI within their organisation. [i]An additional 64 percent plan to use AI across a wide variety of use cases including process automation, risk management, and new revenue generation.
These studies prove that AI is not only becoming more mainstream, but is necessary to help FIs achieve their business goals, strengthen customer relationships, and remain competitive. To demystifying AI and reap its benefits, FIs must embrace a multi-functional strategy that sparks innovation and encourages collaboration. The three use cases below show how AI can be implemented to most immediately impact a financial services organisation.
Elevating employees, not replacing them
AI has historically created fears of job loss and obsolescence. However, within financial services, AI is well equipped to automate manual and repetitive tasks - such as rekeying data - rather than autonomously make critical financial decisions on behalf the organisation. Due to the complexity of the decisions, degree of regulation, and importance of qualitative factors these tasks are – and for the foreseeable future will be – better managed by employees.
A key use case of AI for automation is the utilisation of optical character recognition to streamline the process of spreading financials when underwriting commercial loans. Previously, credit analysts would have to invest hours painstakingly transferring borrower financial data into various systems, reducing time for holistic credit analysis and increasing loan underwriting times. However, by employing AI-driven solutions in combination with powerful workflow automation, banks have been able to significantly increase efficiency in lending processes, reducing processing and cycle times by more than 50 percent and seeing a 10 percent increase in front-office capacity to focus on true value-add analysis and customer relationships. [ii]
Additionally, AI has the ability to empower bankers, not only by eliminating manual tasks, but also by being able to equip them with powerful insights around relationship profitability and credit risk. By refining sophisticated, machine-learning based models, banks can more accurately predict and leverage metrics such as probability of default and loss given default within risk-based pricing models to provide competitive lending rates to borrowers, while still maintaining healthy profitability at the relationship and portfolio level.
Reshaping customer engagement
The acceleration of digital banking due to the COVID-19 pandemic and the rise of customer-centric titans have put significant pressure on financial institutions to modernise and reshape their approach to digital banking to appease rising customer expectations.
Customers not only expect a frictionless and seamless onboarding process, but for their bank to act as an ever-present financial advisor, offering personalised insights on spending habits, money management and financial decisions. AI-powered virtual assistants and chatbots offer new levels of accessibility to common questions by utilising natural language processing to find past transactions, access credit scores, and view balances. However, institutions can take a further step of both anticipating customer needs and offering targeted product suggestions based on propensity scoring models. Proactively offering recommendations can be helpful to customers due to the complexity of different financial products and enables banks to simultaneously satisfy customers while unlocking new revenue opportunities.
FIs can leverage AI to operate as a dedicated advisor, offer a differentiated customer experience, and reduce customer churn. However, equally important to the underlying predictive models is having a single, end-to-end platform to drive direct actionability by delivering insights to the right banker at the right time.
Boosting back-end efficiency
In addition to empowering employees to focus on true value-added activities, AI offers enhanced methods to improve operational efficiency and risk management. Analysis of IDC data shows that AI technologies can improve the cost efficiency of financial institutions by over 25 percent across IT operations[iii].
As a part of fraud detection, institutions can leverage AI to oversee thousands of transactions and efficiently flag anomalies that are indicative of fraud. Historically, transaction monitoring has struggled with false positives, through which genuine transactions are incorrectly flagged. However, through machine learning, actual, fraudulent transactions can be compared to false positives, which can then be fed into the model, improving accuracy over time as the system incorporates learned, differentiating factors.
Finally, the COVID-19 pandemic has caused institutions to revaluate how they assess credit risk and problem loan management. Lenders have had to segment their portfolio by geography and industry to differentiate sectors which were more severely affected by the pandemic versus those that were less challenged. Additionally, there is now a greater emphasis on utilising real-time and transactional data in addition to other data sources to truly understand business performance and borrower resilience. As the uncertain pandemic recovery continues, leveraging AI-powered predictive models in combination with delinquency tracking, credit migration modelling, and other tools will continue to be critical to align actual portfolio risk with the risk appetite of the institution.
As AI adoption continues to mature, FIs should avoid sporadically focusing on isolated use cases. Instead, organisations should strive to align strategy, organisational culture, and digital infrastructure under a united AI strategy. This will help enable them to capitalise on revenue growth, operating efficiency and cost savings, from the front to the back office, and across all lines of business.