Deploying AI Strategies to Mitigate Banking and Fintech Fraud
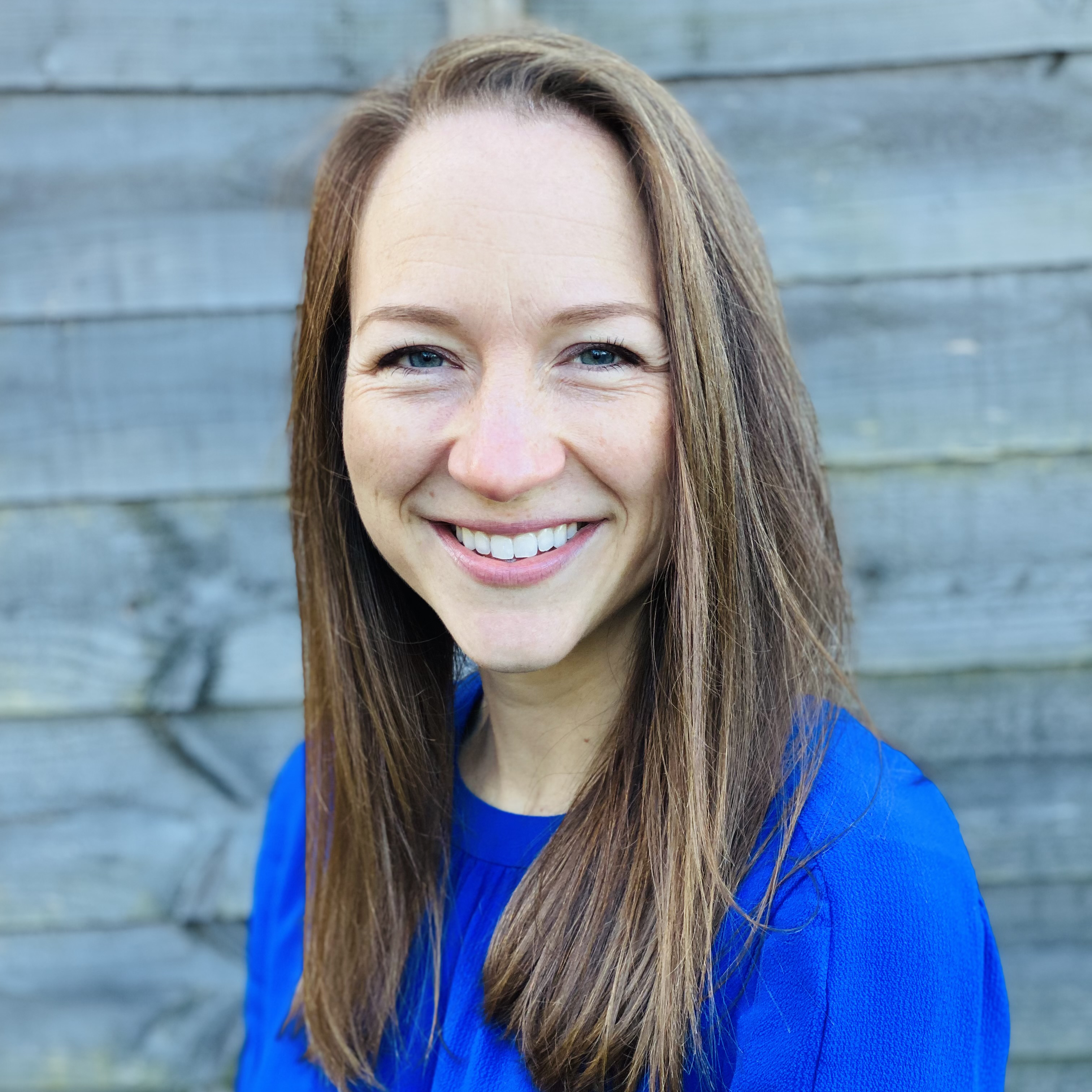
- Carol Hamilton, Chief Growth Officer at Provenir AI
- 12.12.2022 05:00 am #ai #baking #fintech
Research shows that 43 per cent of financial services organizations expect the cost-of-living crisis to increase the risk of financial crime and fraud over the next 12 months, as scammers target vulnerable consumers struggling with rising bills.
Fraud detection and prevention are at the top of the list of reasons why banks and fintech providers are deploying artificial intelligence (AI). According to a recent survey, 78 per cent of financial executives cited fraud prevention as a key driver in the adoption of AI-enabled risk detection in the past year. Additionally, 65 per cent of respondents said improving fraud detection and prevention is one of the primary reasons for using alternative data in risk analysis.
The global business spend on AI-enabled financial fraud detection and prevention strategy platforms is expected to exceed $10 billion globally in 2027; rising from just over $6.5 billion in 2022
As financial fraud and risk vectors are constantly evolving, accessing real-time data and applying it to the latest defensive measures in a fully automated fashion makes AI ideally fit for the fight. Currently, there are solutions available that will automate this process, using automatic testing to select the most effective models for the business problem an organization is trying to solve. For example, there may be 30 models that could show positive results, and to pick the right one, the automation will run through these models to show which model or combination of models is most effective based on the specific needs, such as lowering delinquency rates or supporting more inclusive lending; and when it comes to fraud prevention -- reducing risk and preventing significant losses.
However, deploying models can be daunting – 47 per cent of executives find it difficult to integrate cognitive projects into existing processes and systems. When deployed, performance monitoring is often limited and not real-time, meaning that when the models drift, the reduction in their effectiveness isn’t noticed or addressed as soon as it should be. This directly impacts their ability to make accurate predictions.
Even more, traditional policy-based approaches often fail to identify potential fraud and can produce large volumes of false positives, which then require manual review. What’s needed is a new approach to improve the speed and accuracy of fraud decisions without producing large volumes of false positives.
A more enlightened approach involves leveraging optimized contextual scorecards, machine learning algorithms and outlier detection -- all AI-infused strategies to improve fraud detection and accuracy.
AI enables organizations to build and monitor predictive, explainable and scalable advanced machine learning models to predict fraudulent applications. Depending on business requirements and data availability, both supervised learning and unsupervised learning approaches can be used.
Supervised Learning to Identify Fraud Patterns
Supervised learning involves traditional scorecards and machine learning. Traditional scorecards enable organizations to learn complex relationships from identified fraud to then predict fraud. With machine learning, advanced analytics tools including graph databases are used to discover unknown key relationships, interactions and indicators. By doing this, organizations can identify patterns too complex for traditional scorecards to detect.
Unsupervised Learning for Outlier Detection
Unsupervised learning includes outlier detection which learns from patterns and identifies aberrance. With outlier detection, businesses can look for new and emerging types of fraud by identifying outlier behaviour and utilising tags to differentiate fraud and non-fraud. Similar to supervised approaches, this enables organizations to uncover potential unknown risk factors and immediately mitigate any impact, where necessary.
Four Key Components of an AI-Infused Approach to Fight Fraud
There are four key elements in an AI-infused approach to fraud prevention:
Data Diversity: By leveraging traditional and alternative data, organizations can improve model accuracy, while reducing bias and promoting financial inclusion. An organization’s data is no longer enough, supplementing with third-party data and intelligence sources adds an uplift in accuracy and power of detection.
Strategic Model Selection: This involves choosing the most appropriate algorithm (Gradient Boosting Decision Trees, Random Forests, Deep Neural Networks, etc.) depending on the nature of the dataset, and the use case. Organizations without data science sophistication can leverage automated model development approaches where low-code user input suffices.
Explainability in Model Predictions: This entails fulfilling AI transparency expectations for regulation, audit and clear business practices. Adopting LIME and SHAP explanation techniques, as examples, enable users to understand how and why a model has made a certain prediction. Similarly, without data science sophistication, organizations can seek solutions with these approaches embedded, showing the end user the business-interpretable output in interfaces and dashboards.
Scalability in Data Models: Organizations can reduce the development time from months to days and automatically train, test, monitor and manage data models through sophisticated and accessible DevOps for machine learning, known as “MLOps.” To be successful with an AI project, an organization needs an MLOps solution that simplifies the deployment, monitoring, and retraining of data models. This is something that an organization can build internally, however partnering with an external resource is a cost-effective option.
By adopting AI-infused strategies, organizations can transition from traditional policy-based approaches to those that leverage predictive, explainable and scalable machine learning algorithms to radically improve the speed and accuracy of fraud decisioning.